PCSAGAN: a physics-constrained generative network based on self-attention for high-fidelity flow field reconstruction
Journal of Visualization(2024)
摘要
We propose a physics-constrained generative adversarial network, PCSAGAN, based on the self-attention mechanism for high-fidelity flow field reconstruction, which can generate high-resolution and high-precision volumes for two-dimensional time-varying datasets. PCSAGAN consists of two discriminators and one generator, capable of preserving temporal coherence and spatial characteristics. It avoids the heavy computation of multilayer convolution and obtains spatial flow evolution information by using the self-attention mechanism. A soft-constraint loss function is further employed to effectively utilize the underlying physical properties. Moreover, the dataset is generated from real-world simulations rather than artificial synthesis, which ensures generalizability to practical applications. We compare PCSAGAN against volume upscaling methods using BI, ESPCN and SSR-TVD, and demonstrate its effectiveness with several time-varying flow fields through quantitative and qualitative evaluations. Meanwhile, a re-analysis framework is introduced to enable potential later visualization and exploratory analysis of the reconstructed flow field data.
更多查看译文
关键词
Time-varying data visualization,Deep learning,Super-resolution,Generative adversarial network
AI 理解论文
溯源树
样例
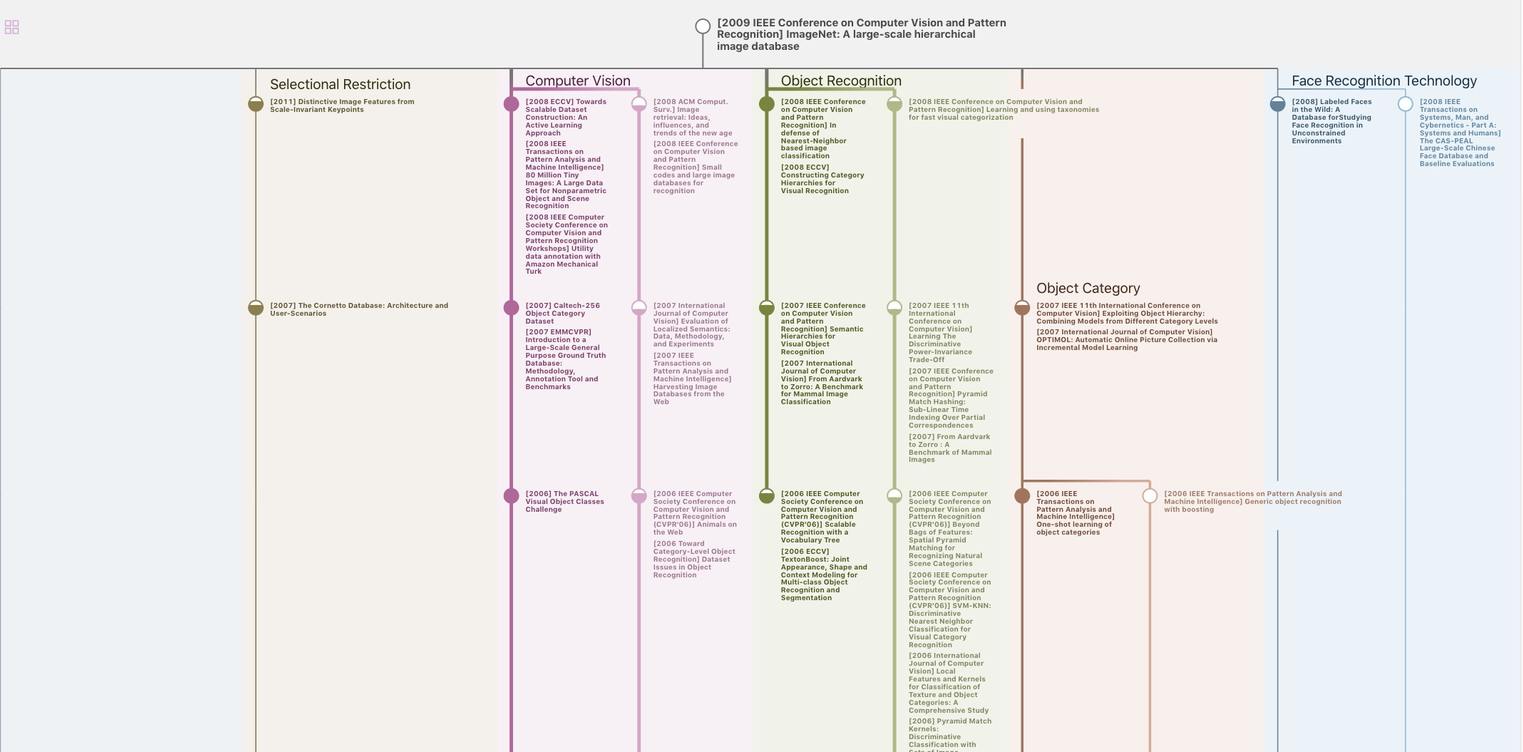
生成溯源树,研究论文发展脉络
Chat Paper
正在生成论文摘要