Domain Invariant Driving Behaviour Prediction Based on Autoencoder Anomaly Detection.
Annual IEEE International Conference on Pervasive Computing and Communications(2024)
摘要
Driving behavior prediction has become increasingly popular and challenging in developing advanced driver assistance systems (ADAS) systems. The main challenge lies with the varying domains and surroundings. Current ADAS systems fail to predict when a driver drives in a different environment, often leading to accidents. To mitigate these challenges, one possible approach is to understand the changes observed in the regular driving behavior of the driver. This paper presents a model for predicting driving behavior across different domains using autoencoder anomaly detection and, thereby, defines a metric to compute the driving behavior score. The experimental setup includes a pilot study for feature selection and a semi-controlled experiment for data collection across multiple countries. The findings highlight the significant role of in-vehicle driving features and physiological features of the driver in predicting domain-invariant driving behavior based on contextual information.
更多查看译文
关键词
ADAS Systems,Domain Adaptation,Anomalies,Driving behavior,Multi-modal datasets
AI 理解论文
溯源树
样例
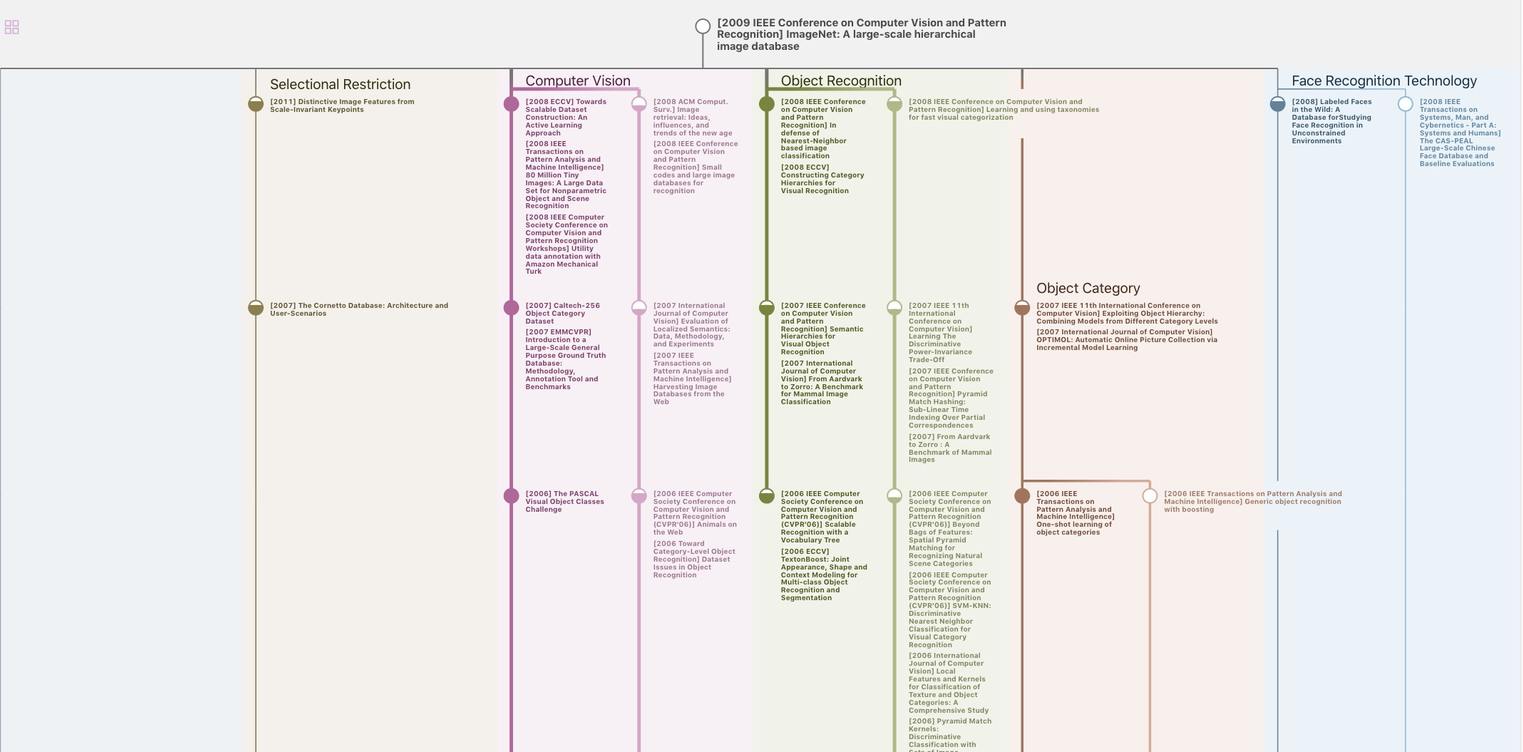
生成溯源树,研究论文发展脉络
Chat Paper
正在生成论文摘要