Exploring Activity Recognition in Multi-device Environments using Hierarchical Federated Learning.
Annual IEEE International Conference on Pervasive Computing and Communications(2024)
摘要
Federated learning enables collaborative training of machine learning models on internet of things (IoT) devices, without the need to share their local raw data. There are many applications of federated learning, including mobile and distributed computing for human activity, fitness, and health monitoring. Recently, hierarchical federated learning approaches have been proposed to allow for more efficient model aggregation, tailored to the specific requirements or attributes of particular environments (e.g., limited processing resources and network connectivity). Hierarchical federated learning, to date, has been demonstrated mostly in single-device environments, with limited research in available in multi-device environments. This paper presents an approach for activity recognition in multi-device envi-ronmentsusing hierarchical federated learning that demonstrates the advantages of distributing processing capabilities across the cloud-edge-client continuum. Considering heterogeneous devices in a multi-device environment, this paper proposes an accuracy-and energy-aware device selection mechanism that efficiently utilises computing resources of resource-constrained embedded devices to improve performance in terms of activity recognition and battery consumption (active lifetime). When evaluated using a publicly available activity recognition dataset, the proposed mechanism offers significantly enhanced performance compared to the state-of-the-art.
更多查看译文
AI 理解论文
溯源树
样例
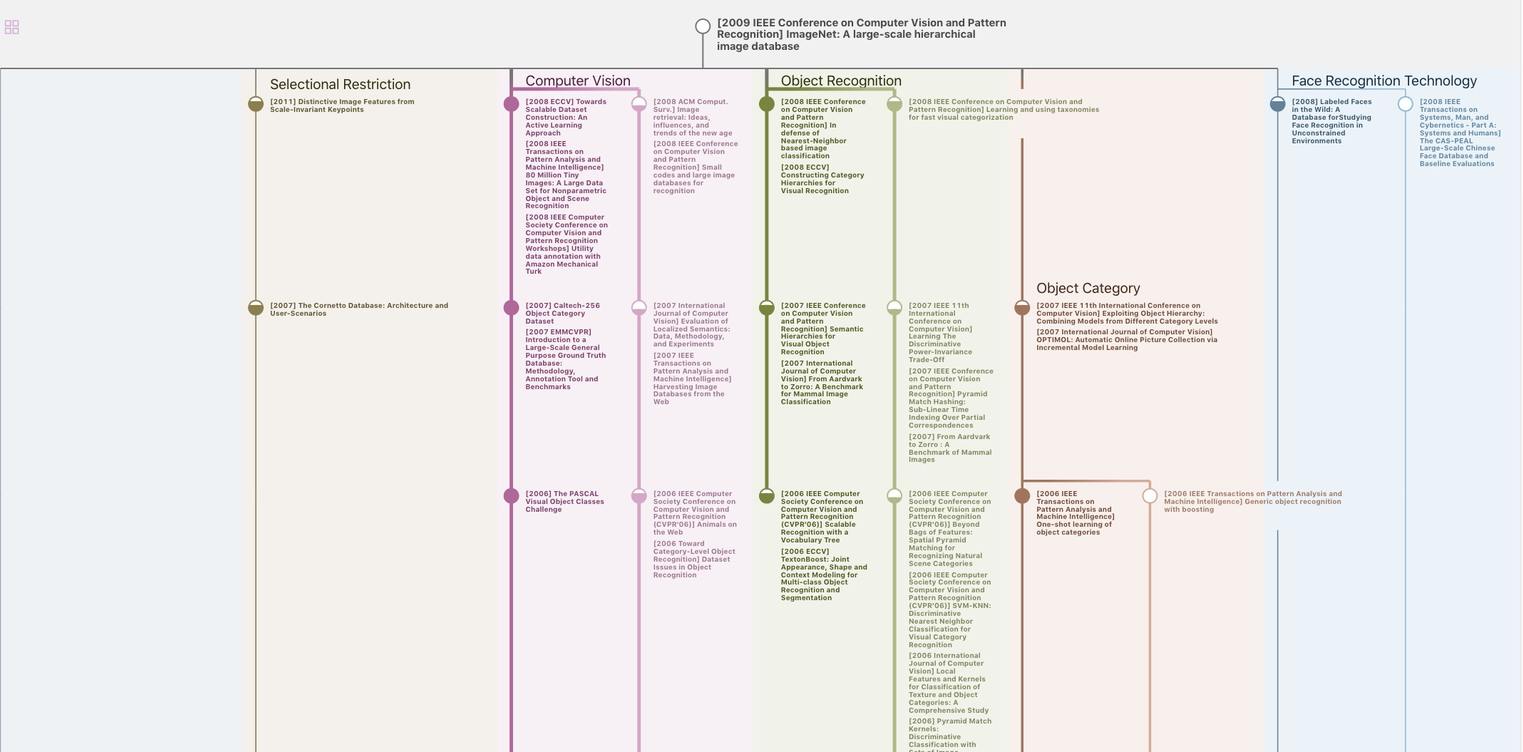
生成溯源树,研究论文发展脉络
Chat Paper
正在生成论文摘要