Human Mobility: Prediction and Predictability.
Annual IEEE International Conference on Pervasive Computing and Communications(2024)
摘要
Predicting human mobility is of significant social and economic benefits, such as for urban planning and infectious disease prevention, e.g., COVID-19. Predictability, namely to what extent a trustworthy prediction can be made from limited data, is key to exploiting prediction for informed decision-making. Current approaches to predictability are usually model-specific along with a relative measurement, leading to varying approximate results and the lack of benchmark assessment criteria. To address this, this study proposes a model-independent method based on permutation entropy to compute an absolute measure of predictability, in particular to derive the maximum level of prediction. Special emphasis is placed on investigating the sensitivity of the predictability methods to changing data loss rates and data lengths. The method has been evaluated using a public dataset with the mobile data of 500,000 individuals. Initial results show a 92%-tighter than before potential predictability and prove the hypothesis of correlation between the minimum amount of data and the level of accuracy of prediction.
更多查看译文
AI 理解论文
溯源树
样例
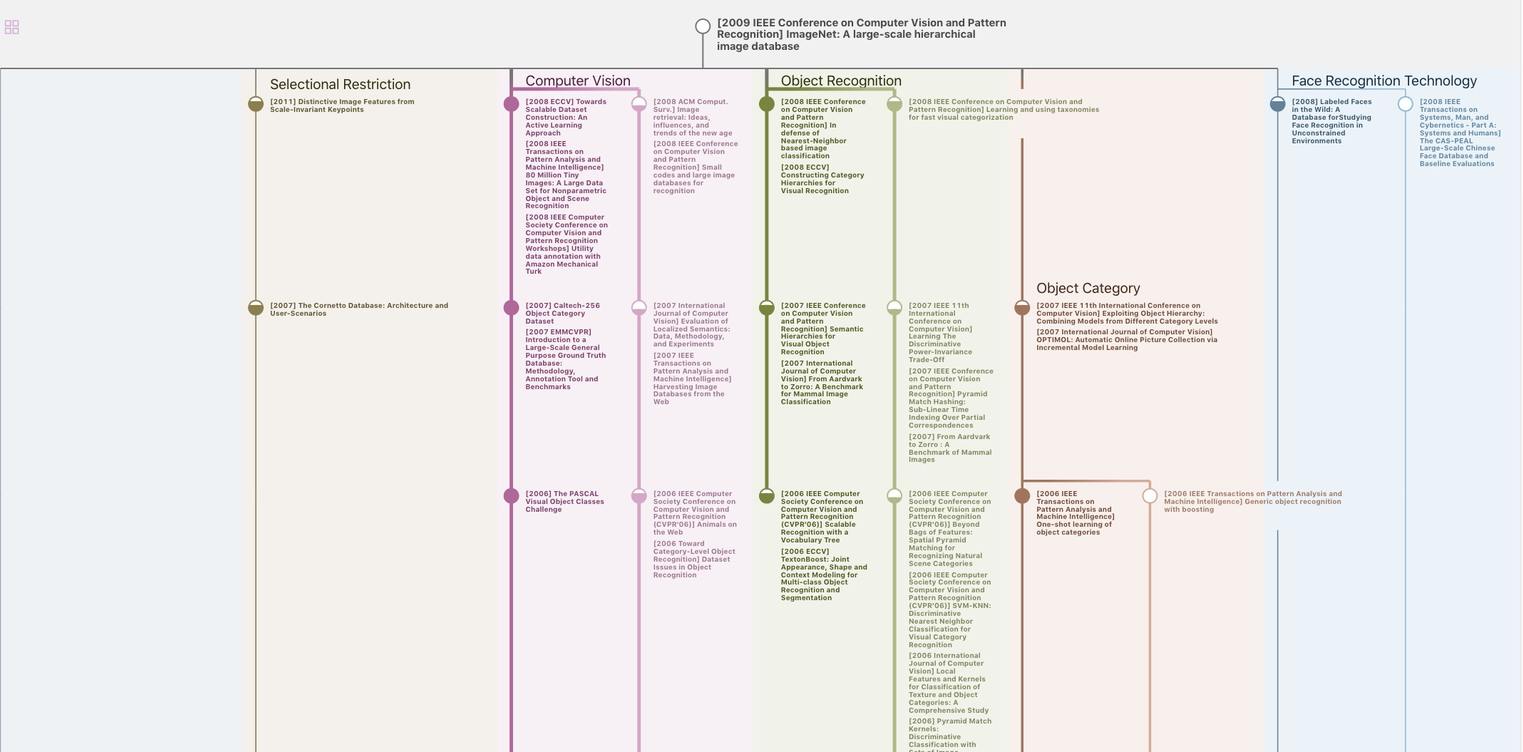
生成溯源树,研究论文发展脉络
Chat Paper
正在生成论文摘要