Assessing the Reproducibility, Stability, and Biological Interpretability of Multimodal Computed Tomography Image Features for Prognosis in Advanced Non‐small Cell Lung Cancer
iRADIOLOGY(2024)
摘要
Abstract Background Despite the existence of proposed prognostic features on computed tomography (CT) for patients with advanced‐stage non‐small cell lung cancer (NSCLC), including radiologists' handcrafted (RaH) features, radiomics features, and deep learning features, comprehensive studies that examine their reproducibility, stability, and biological interpretability remain limited. Methods The Image Biomarker Standardization Initiative‐reported tolerance, Kappa, interclass correlation coefficient, and coefficient of variance were employed to identify reproducible features among RaH, radiomics, and deep learning features derived from NSCLC phantoms. The reproducible features were then input into six artificial intelligence algorithms to develop prognostic models for targeted therapy and immunotherapy using real‐world patients with advanced‐stage NSCLC to assess their capability and stability. Pathway enrichment was also conducted to explore the underlying biological pathways associated with these reproducible features. Results Reproducible features in advanced NSCLC included RaH features (9/9, 100%), radiomics features (572/1835, 31.17%), and deep learning features (3442/4096, 84.03%). Among the six artificial intelligence‐based prognostic methods, the RaH features exhibited least variability. We also observed that the optimal CT‐based prognostic approach differed depending on treatment regimens for advanced NSCLC. In analysis using the Cancer Genome Atlas Program lung adenocarcinoma dataset, the identified reproducible prognostic features, specifically tumor size‐derived radiomics and RaH features, showed significant associations with five key signaling pathways involved in NSCLC survival outcomes (false‐discovery rate p < 0.05). Conclusions By elucidating the reproducibility, stability, and biological associations of prognostic CT features, our study provides valuable evidence for future NSCLC studies and modeling approaches.
更多查看译文
关键词
artificial intelligence,computed tomography,critical pathways,non‐small cell lung cancer,x‐ray
AI 理解论文
溯源树
样例
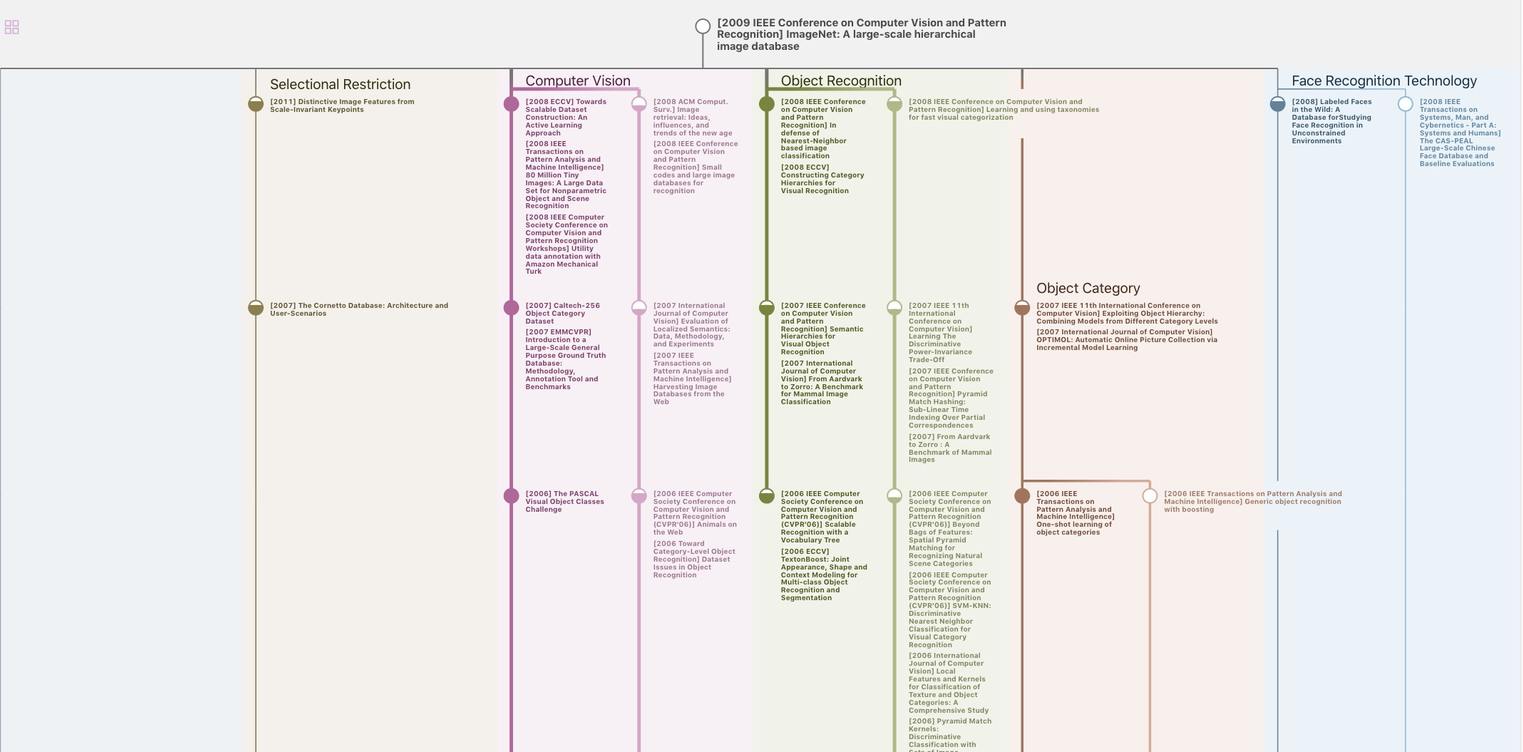
生成溯源树,研究论文发展脉络
Chat Paper
正在生成论文摘要