Improving Eye-Tracking Data Quality: A Framework for Reproducible Evaluation of Detection Algorithms.
Sensors (Basel, Switzerland)(2024)
摘要
High-quality eye-tracking data are crucial in behavioral sciences and medicine. Even with a solid understanding of the literature, selecting the most suitable algorithm for a specific research project poses a challenge. Empowering applied researchers to choose the best-fitting detector for their research needs is the primary contribution of this paper. We developed a framework to systematically assess and compare the effectiveness of 13 state-of-the-art algorithms through a unified application interface. Hence, we more than double the number of algorithms that are currently usable within a single software package and allow researchers to identify the best-suited algorithm for a given scientific setup. Our framework validation on retrospective data underscores its suitability for algorithm selection. Through a detailed and reproducible step-by-step workflow, we hope to contribute towards significantly improved data quality in scientific experiments.
更多查看译文
关键词
eye-tracking,pupil detection algorithm,methodological framework,detection quality
AI 理解论文
溯源树
样例
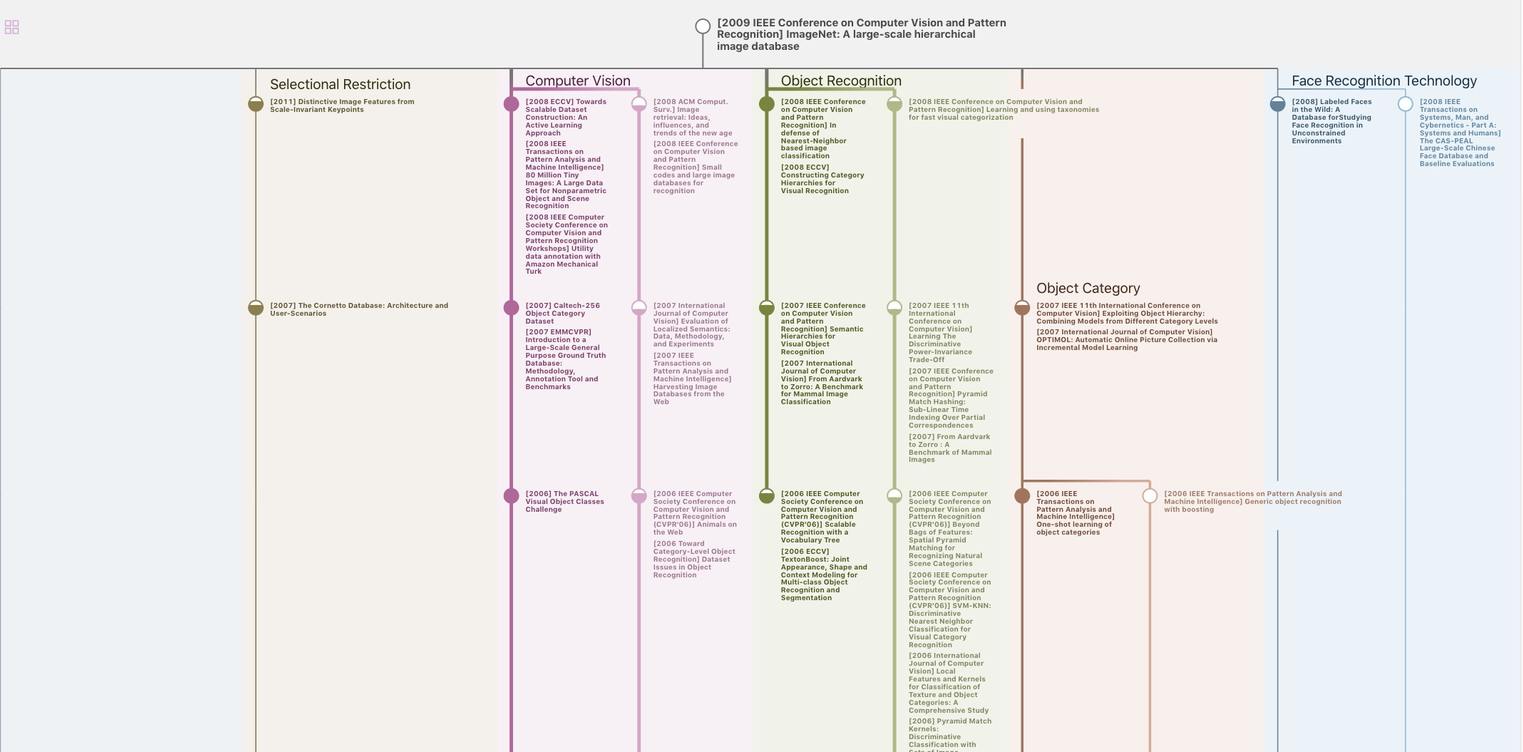
生成溯源树,研究论文发展脉络
Chat Paper
正在生成论文摘要