Comparison of hypospadias phenotype pixel segmentation to GMS score.
Journal of Pediatric Urology(2024)
摘要
Background
Hypospadias phenotype assessment determines if the anatomy is favorable for reconstruction. Glans-Urethral Meatus-Shaft (GMS) has been adopted in an effort to standardize hypospadias classification. While extremely subjective, GMS has been widely used to classify the severity of the phenotype to predict surgical outcomes. The use of digital image analysis has proven to be feasible and prior efforts by our team have demonstrated that machine learning algorithms can emulate an expert’s assessment of the phenotype. Nonetheless, the creation of these image recognition algorithms is highly subjective. In order to reduce a subjective input in the evaluation of the phenotype, we propose a novel approach to analyze the anatomy using digital image pixel analysis and to compare the results using the GMS score. Our hypothesis is that pixel cluster segmentation can discriminate between favorable and unfavorable anatomy.
Objective
To evaluate whether image segmentation and digital pixel analysis are able to analyze favorable vs unfavorable hypospadias anatomy in a less subjective manner than GMS score.
Methods
A total of 148 patients with different types of hypospadias were classified by 1 of 5 independent experts following the GMS score into “favorable” (GG), “moderately favorable” (GM) and “unfavorable” (GP) glans. From there, 592 images were generated using digital image segmentation. 584 were included for final analysis due to certain images being excluded for poor image quality or inadequate capture of target anatomy. For each image, the region of interest was segmented separately by two evaluators into “glans,” “urethral plate,” “foreskin” and “periurethral plate”. The values obtained for each segmented region using machine-learning statistical pixel k-means cluster analysis were analyzed and compared to the GMS score given to that image using an ANOVA analysis.
Results
Analysis of image segmentation demonstrated that k-means pixel cluster analysis discriminated “favorable” vs “unfavorable” urethral plates. There was a significant difference between scores when comparing the GG and GM groups (p = 0.03) and GG and GP groups (p = 0.05). Pixel cluster analysis could not discriminate between “moderately favorable” and “unfavorable” urethral plates.
Conclusions
Through our analysis, we found significant pairwise difference for different tissue qualities. Digital image segmentation and statistical k-means cluster analysis can discriminate anatomical features in a similar way to the GMS score. Future research can target discerning between different tissue qualities in an effort to predict surgical outcomes for hypospadias repair.
更多查看译文
关键词
hypospadias,machine learning,k-means,GMS score
AI 理解论文
溯源树
样例
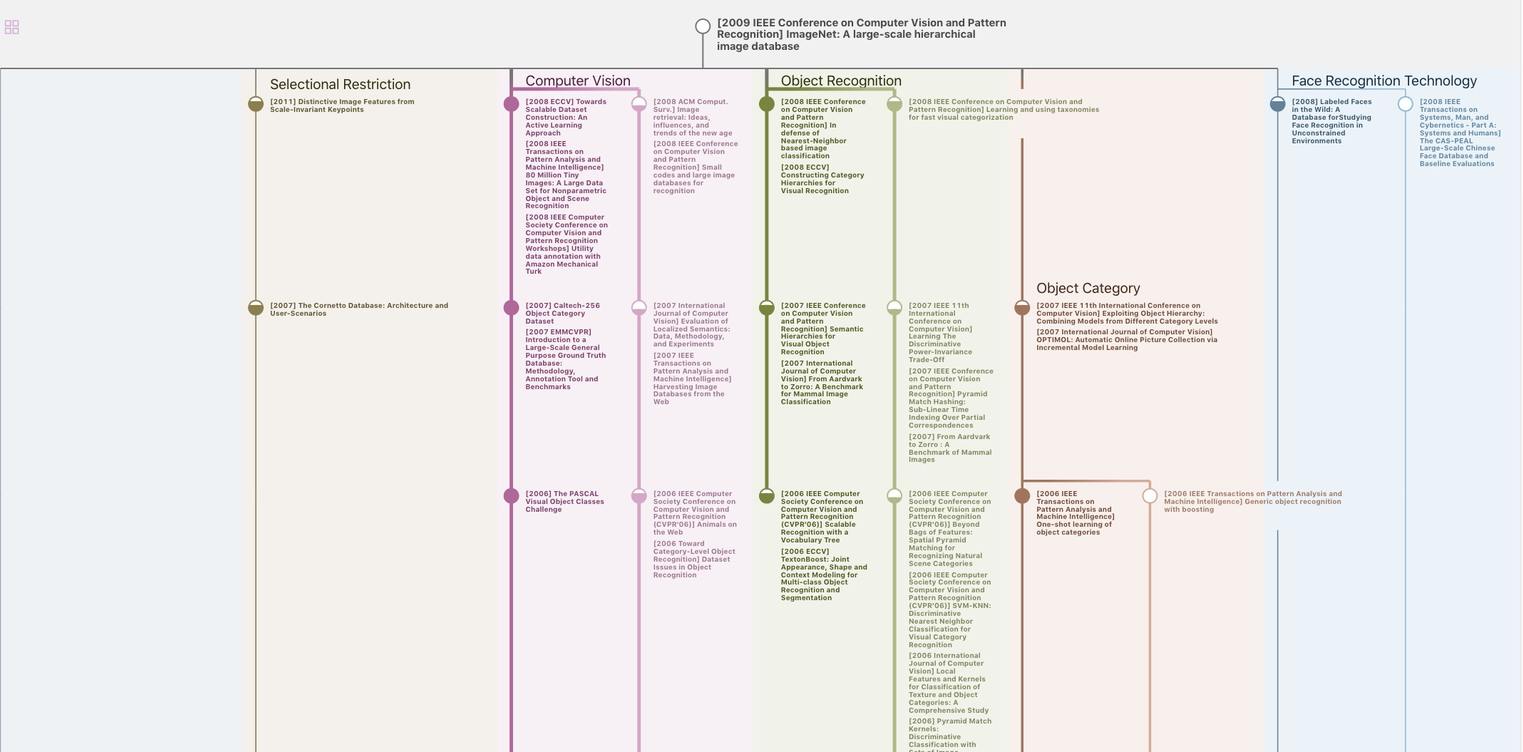
生成溯源树,研究论文发展脉络
Chat Paper
正在生成论文摘要