Efficient EndoNeRF reconstruction and its application for data-driven surgical simulation
INTERNATIONAL JOURNAL OF COMPUTER ASSISTED RADIOLOGY AND SURGERY(2024)
摘要
Purpose The healthcare industry has a growing need for realistic modeling and efficient simulation of surgical scenes. With effective models of deformable surgical scenes, clinicians are able to conduct surgical planning and surgery training on scenarios close to real-world cases. However, a significant challenge in achieving such a goal is the scarcity of high-quality soft tissue models with accurate shapes and textures. To address this gap, we present a data-driven framework that leverages emerging neural radiance field technology to enable high-quality surgical reconstruction and explore its application for surgical simulations.Method We first focus on developing a fast NeRF-based surgical scene 3D reconstruction approach that achieves state-of-the-art performance. This method can significantly outperform traditional 3D reconstruction methods, which have failed to capture large deformations and produce fine-grained shapes and textures. We then propose an automated creation pipeline of interactive surgical simulation environments through a closed mesh extraction algorithm.Results Our experiments have validated the superior performance and efficiency of our proposed approach in surgical scene 3D reconstruction. We further utilize our reconstructed soft tissues to conduct FEM and MPM simulations, showcasing the practical application of our method in data-driven surgical simulations.Conclusion We have proposed a novel NeRF-based reconstruction framework with an emphasis on simulation purposes. Our reconstruction framework facilitates the efficient creation of high-quality surgical soft tissue 3D models. With multiple soft tissue simulations demonstrated, we show that our work has the potential to benefit downstream clinical tasks, such as surgical education.
更多查看译文
关键词
3D reconstruction,NeRF,Robotic surgery,Surgery simulation
AI 理解论文
溯源树
样例
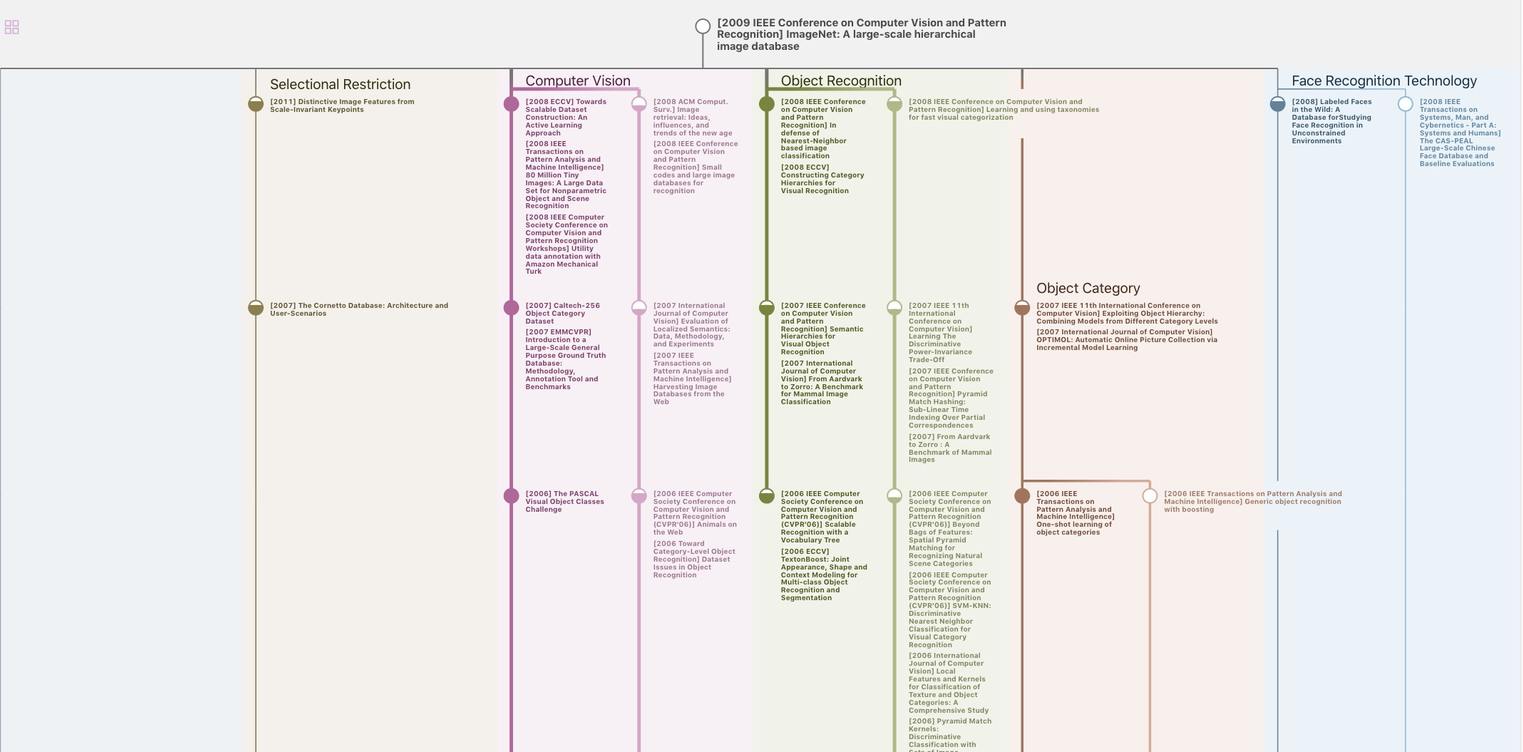
生成溯源树,研究论文发展脉络
Chat Paper
正在生成论文摘要