Invariant Features for Accurate Predictions of Quantum Chemical UV-vis Spectra of Organic Molecules
SOUTHEASTCON 2024(2024)
摘要
Including invariance of global properties of a phys-ical system as an intrinsic feature in graph neural networks (GNNs) enhances the model's robustness and generalizability and reduces the amount of training data required to obtain a desired accuracy for predictions of these properties. Existing open source GNN libraries construct invariant features only for specific GNN architectures. This precludes the generalization of invariant features to arbitrary message passing neural network (MPNN) layers which, in turn, precludes the use of these libraries for new, user-specified predictive tasks. To address this limitation, we implement invariant MPNNs into the flexible and scalable HydraGNN architecture. HydraGNN enables a seamless switch between various MPNNs in a unified layer sequence and allows for a fair comparison between the predictive performance of different MPNNs. We trained this enhanced HydraGNN archi-tecture on the ultraviolet-visible (UV-vis) spectrum of GDB-9 molecules, a feature that describes the molecule's electronic exci-tation modes, computed with time-dependent density functional tight binding (TD-DFTB) and available open source through the GDB-9-Ex dataset. We assess the robustness (i.e., accuracy and generalizability) of the predictions obtained using different invariant MPNNs with respect to different values of the full width at half maximum (FWHM) for the Gaussian smearing of the theoretical peaks. Our numerical results show that incorporating invariance in the HydraGNN architecture significantly enhances both accuracy and generalizability in predicting UV-vis spectra of organic molecules.
更多查看译文
关键词
Graph Neural Networks,Deep Learning,Com-putational Chemistry,Ultraviolet-Visible Spectrum,Density Functional Tight Binding
AI 理解论文
溯源树
样例
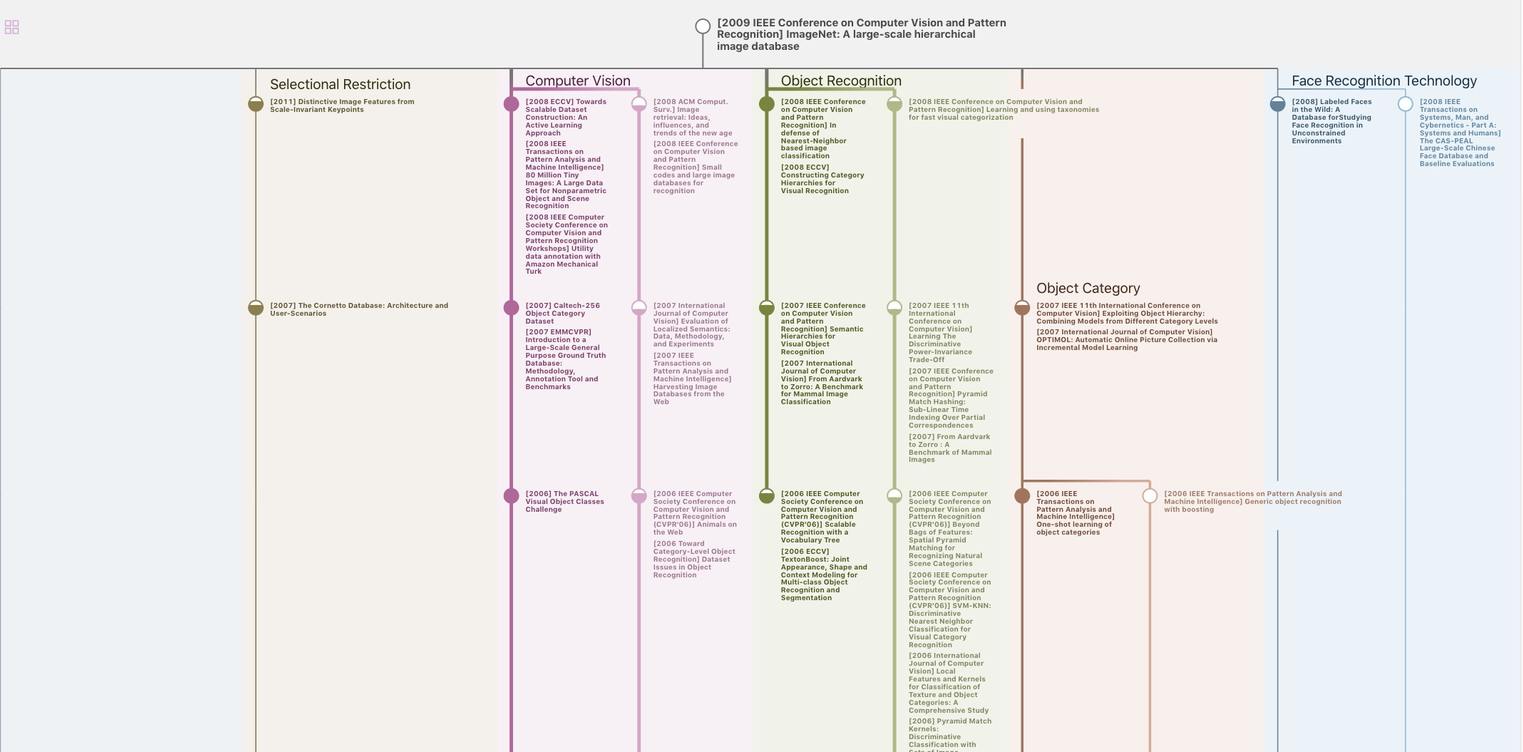
生成溯源树,研究论文发展脉络
Chat Paper
正在生成论文摘要