Integration of Planning and Deep Reinforcement Learning in Speed and Lane Change Decision-Making for Highway Autonomous Driving
IEEE transactions on transportation electrification(2024)
摘要
The intricate interactions with other road users and the diversity of traffic environments create a challenging decision-making task for autonomous driving systems. While offline learning solutions are renowned for their high execution efficiency and the ability to approximate the optimal policy across the entire state space, they are often unsafe and fragile when encountering untrained states. Conversely, online planning methods possess the capacity to thoroughly assess how current decisions influence future outcomes online, and therefore have better generalization. Nevertheless, these approaches face difficulties in terms of execution efficiency and are susceptible to becoming stuck in locally optimal solutions. In this context, this paper proposes an Integrated Planning and Learning (IPL) algorithm based on the reinforcement learning framework for speed and lane change decision-making on highways. Specifically, at each decision time step, this method utilizes the offline learned model to guide an online Monte Carlo Tree Search (MCTS) algorithm for heuristic search, aiming to formulate a forward-looking policy. The experimental results show that the IPL algorithm performs better generalization when faced with unknown scenarios, and its asymptotic performance is better than other benchmark algorithms. In addition, in contrast to the MCTS-based online planning method, the IPL algorithm enhances execution efficiency and comes closer to achieving global optimality.
更多查看译文
关键词
Decision-making,offline learning,online planning,highway autonomous driving
AI 理解论文
溯源树
样例
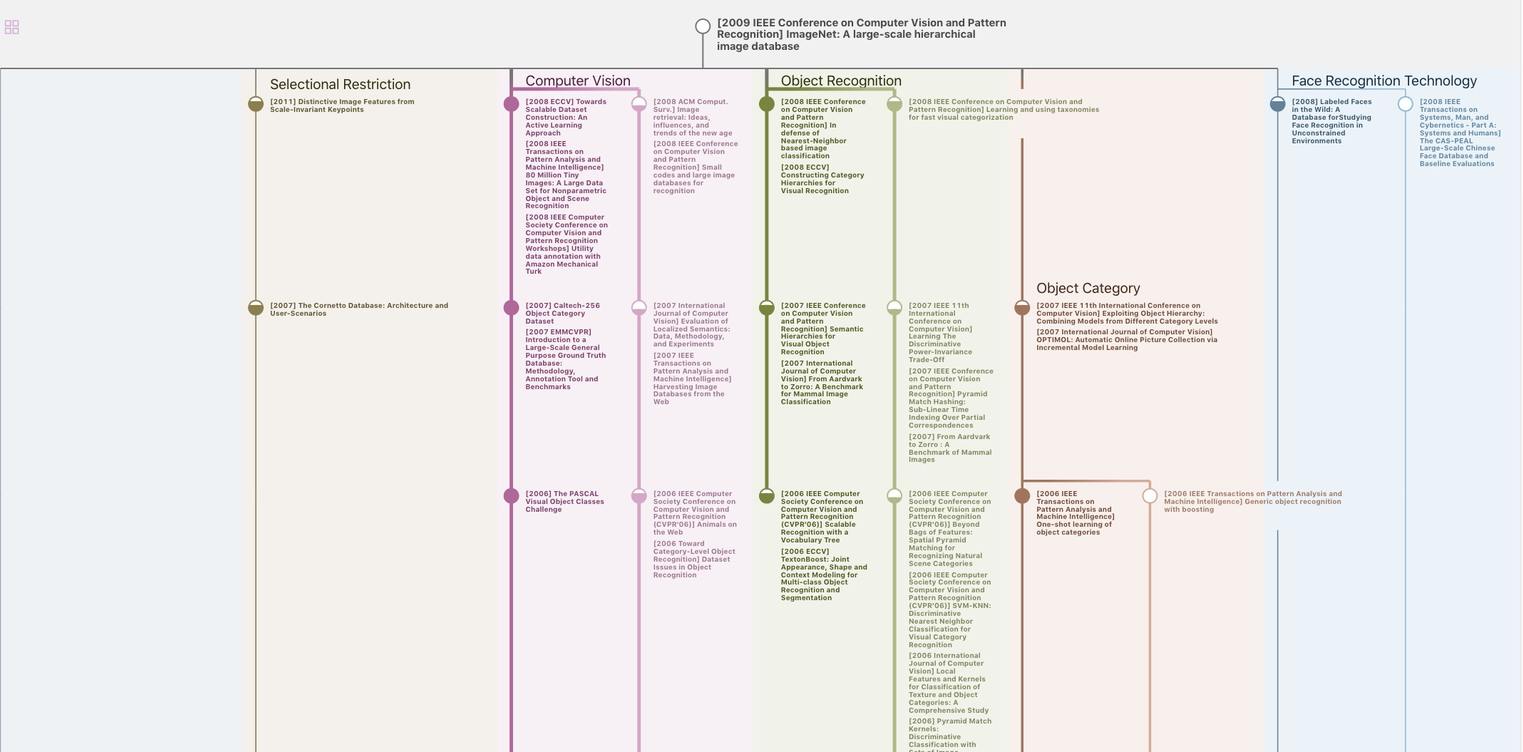
生成溯源树,研究论文发展脉络
Chat Paper
正在生成论文摘要