The effect of time normalization and biomechanical signal processing techniques of ground reaction force curves on deep-learning model performance
Journal of Biomechanics(2024)
摘要
Time-series data are common in biomechanical studies. These data often undergo pre-processing steps such as time normalization or filtering prior to use in further analyses, including deep-learning classification. In this context, it remains unclear how these preprocessing steps affect deep-learning model performance. Thus, the aim of this study is to assess the effect of time-normalization and filtering on the performance of deep-learning classification models. We also investigated the effect of amplitude scaling. Using a public dataset (Gutenburg Gait Database, a ground reaction force database of level overground walking at self-selected walking speed involving 350 healthy individuals), we trained convolutional neural network (CNN) and long short-term memory (LSTM) models to predict sex (binary male, female) using three-dimensional ground-reaction forces to which we applied different processing approaches: zero padding, interpolation to 100% of signal, filtering, and scaling (min–max, body mass). The results show that transformations resulted in differences in model performances. Highest performance was obtained using unfiltered data, zero-padding, and min–max amplitude scaling (F1-score of 91 and 87% for CNN and LSTM, respectively). Not filtering data and using min–max scaling generally improve performance for both model architectures. For interpolation, results are not consistent across model architectures. This study suggests that processing steps must be considered in applications where deep-learning classification performance is relevant.
更多查看译文
关键词
Biomechanics,Ground reaction forces,Machine learning,Gutenburg Gait Database
AI 理解论文
溯源树
样例
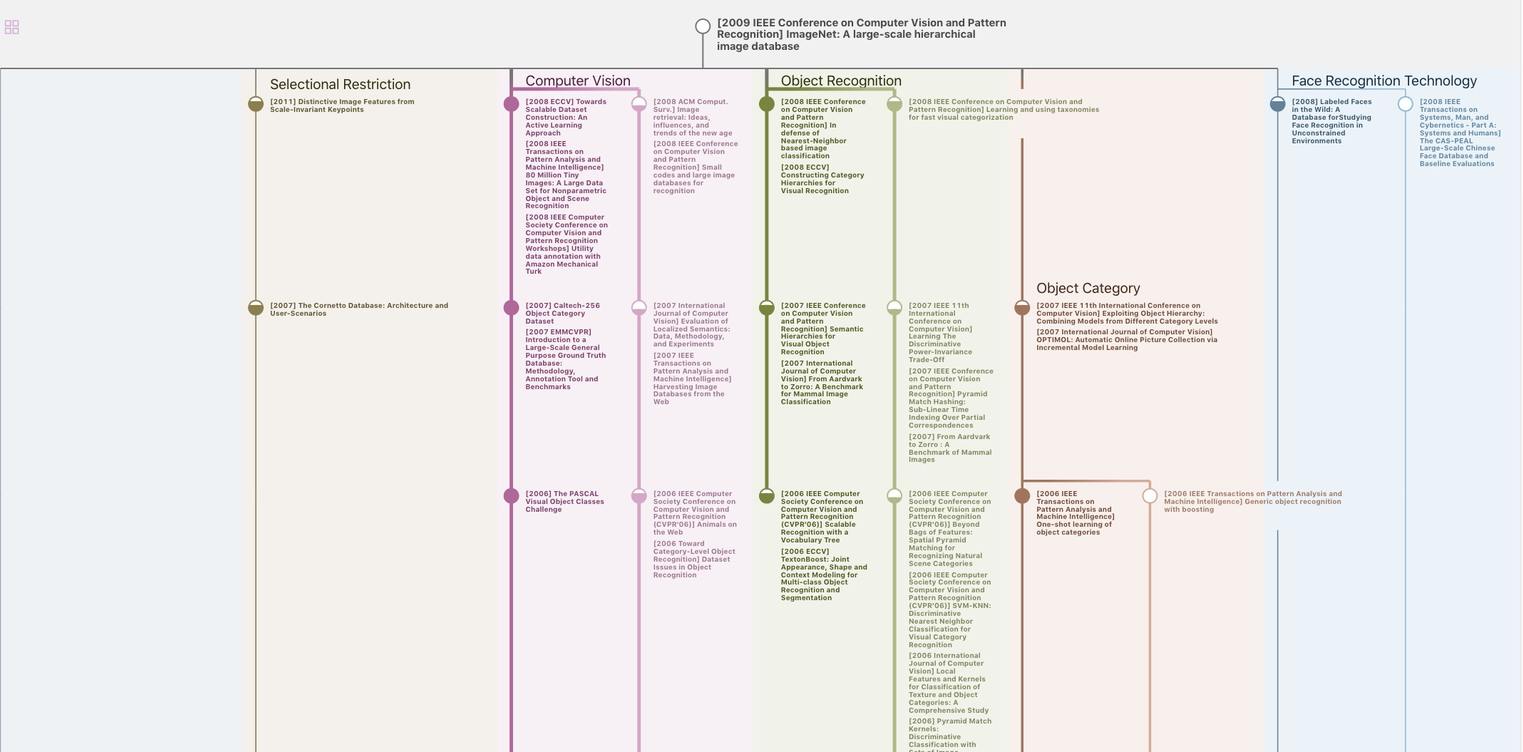
生成溯源树,研究论文发展脉络
Chat Paper
正在生成论文摘要