Biologically-Informed Excitatory and Inhibitory Balance for Robust Spiking Neural Network Training
arxiv(2024)
摘要
Spiking neural networks drawing inspiration from biological constraints of
the brain promise an energy-efficient paradigm for artificial intelligence.
However, challenges exist in identifying guiding principles to train these
networks in a robust fashion. In addition, training becomes an even more
difficult problem when incorporating biological constraints of excitatory and
inhibitory connections. In this work, we identify several key factors, such as
low initial firing rates and diverse inhibitory spiking patterns, that
determine the overall ability to train spiking networks with various ratios of
excitatory to inhibitory neurons on AI-relevant datasets. The results indicate
networks with the biologically realistic 80:20 excitatory:inhibitory balance
can reliably train at low activity levels and in noisy environments.
Additionally, the Van Rossum distance, a measure of spike train synchrony,
provides insight into the importance of inhibitory neurons to increase network
robustness to noise. This work supports further biologically-informed
large-scale networks and energy efficient hardware implementations.
更多查看译文
AI 理解论文
溯源树
样例
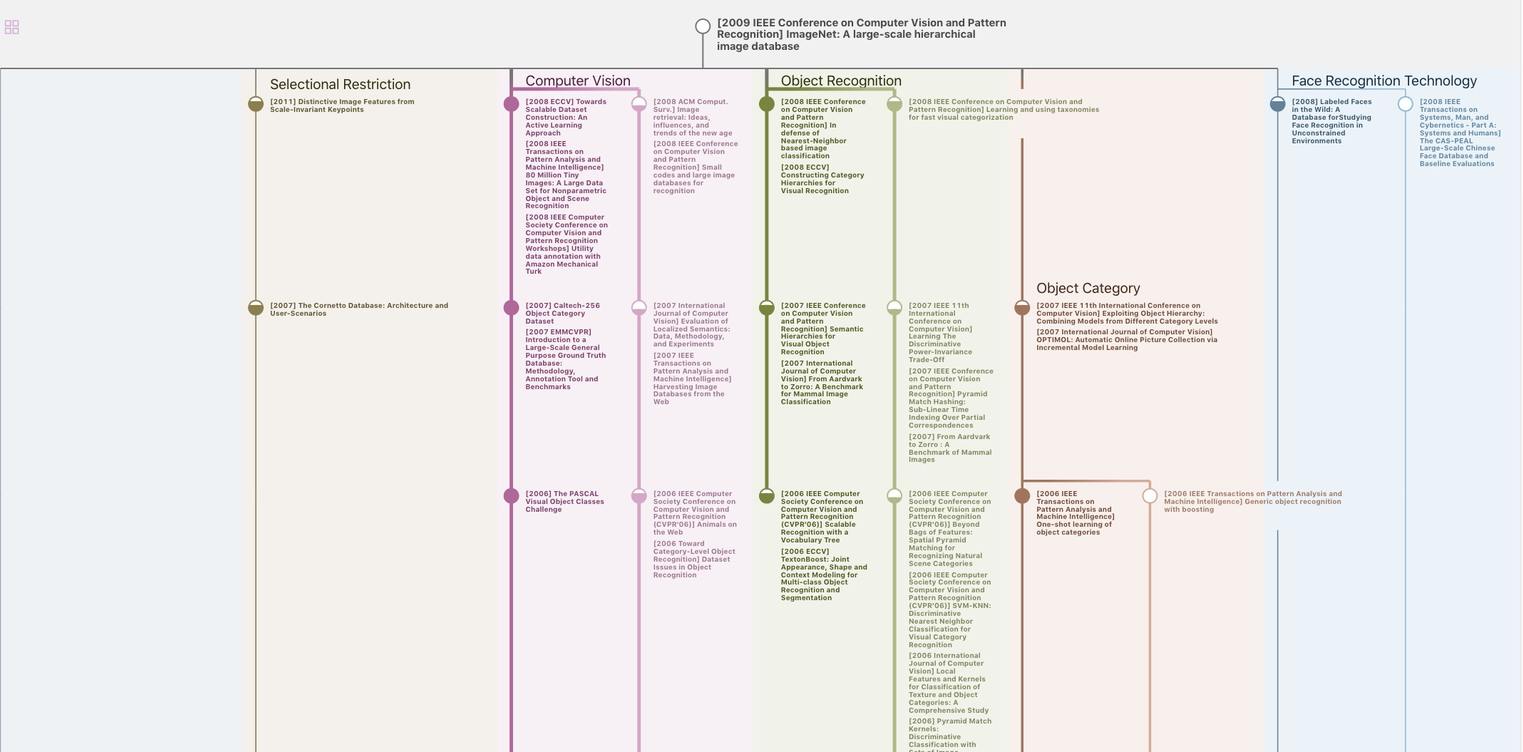
生成溯源树,研究论文发展脉络
Chat Paper
正在生成论文摘要