AK-Gibbs: An active learning Kriging model based on Gibbs importance sampling algorithm for small failure probabilities
Computer Methods in Applied Mechanics and Engineering(2024)
摘要
In engineering practices, it is a time-consuming procedure to estimate the small failure probability of highly nonlinear and dimensional limit state functions and Kriging-based methods are more effective representatives. However, it is an important challenge to construct the candidate importance sample pool for Kriging-based small failure probability analysis methods with multiple input random variables when the Metropolis-Hastings (M-H) algorithm with acceptance-rejection sampling principle is employed. To address the challenge and estimate the reliability of structures in a more efficient and accurate way, an active learning Kriging model based on the Gibbs importance sampling algorithm (AK-Gibbs) is proposed, especially for the small failure probabilities with nonlinear and high-dimensional limit state functions. A new active learning function that can be directly linked to the global error is first constructed. Weighting coefficients of the joint probability density function in the new active learning function are then determined to select the most probable points (MPPs) and update samples efficiently and accurately. The Gibbs importance sampling algorithm is derived based on the Gibbs algorithm to effectively establish the candidate importance sample pool. An improved global error-based stopping criterion is finally constructed to avoid pre-mature or late-mature for the estimation of small failure probabilities with complicated failure domains. Two numerical and four engineering examples are respectively employed to elaborate and validate the effectiveness of the proposed method.
更多查看译文
关键词
Reliability analysis,Small failure probability,Active learning function,Kriging model,Gibbs sampling
AI 理解论文
溯源树
样例
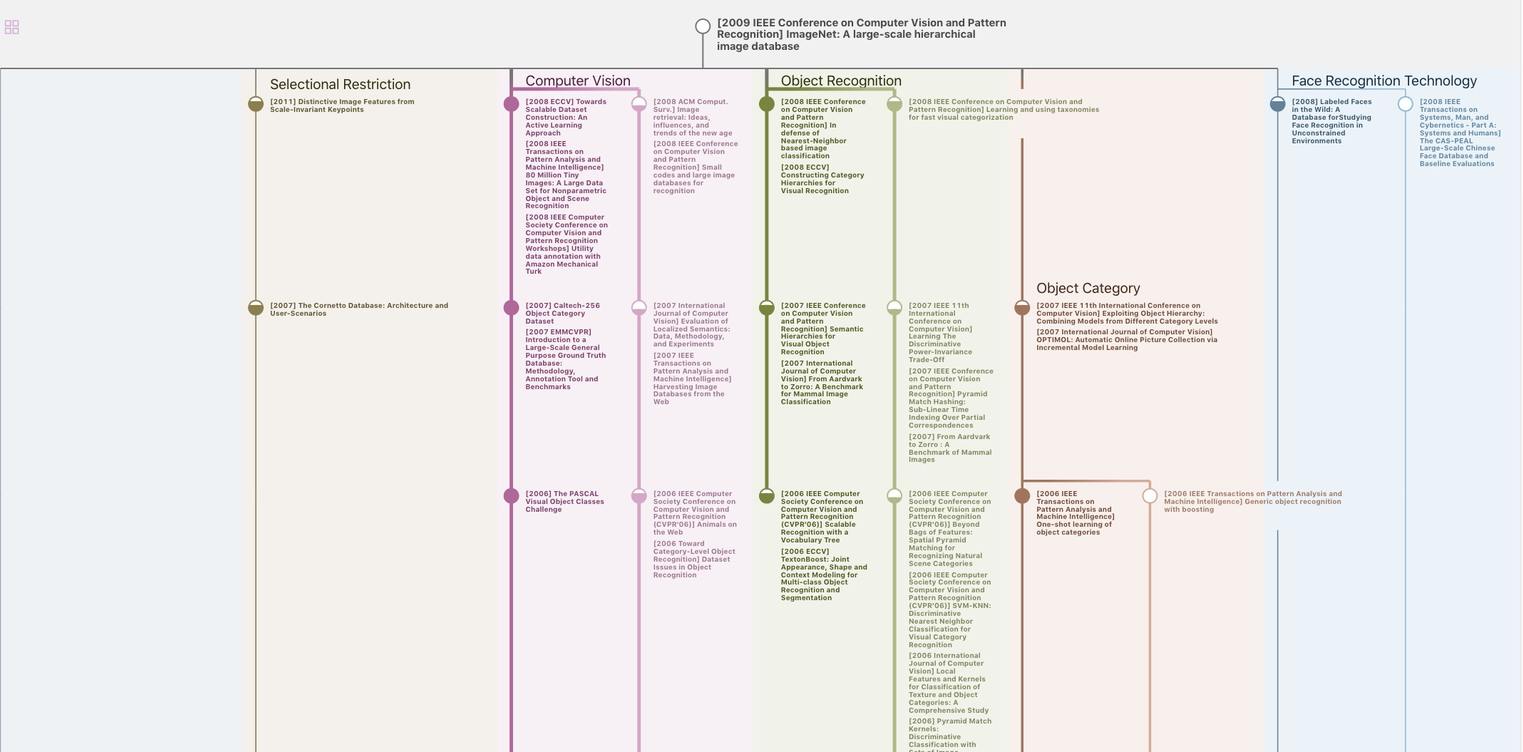
生成溯源树,研究论文发展脉络
Chat Paper
正在生成论文摘要