P066 Predictive Modeling of Infection Risk in Lupus Nephritis using Multiple Machine Learning Algorithms
Rheumatology(2024)
摘要
Abstract Background/Aims The incidence of infections is considerably higher among patients with lupus nephritis (LN), and these infections significantly contribute to mortality rates. This study analysed the characteristics of peripheral blood lymphocyte subsets in LN patients, aiming at using machine learning (ML) methods to explore the risk factors of infection and establish a most effective ML algorithm to predict the occurrence of co-infection in LN. Methods This retrospective study encompassed LN patients, consisting of 111 non-infected individuals and 72 infected individuals. Additionally, 206 healthy controls (HCs) matched age and sex were recruited. The patient’s basic information, infection site, pathogen type, clinical manifestation, patient medication, and auxiliary laboratory indexes were recorded. Eight ML methods were compared to establish a corresponding model through a training group, and then verify the results in a test group. We trained the ML models, including Logistic Regression (LR), Decision Tree (DT), K-Nearest Neighbors (KNN), Support Vector Machine (SVM), Multi-Layer Perceptron (MLP), Random Forest (RF), Ada boost (Ada), Extreme Gradient Boosting (XGB), after selecting variables for LN infection prediction in the training set, and further evaluated potential predictors of infection. Results The levels of T, B, helper T, suppressor T, Natural killer cells in the infected patients were significantly decreased when compared with both non-infected LN patients and HCs. The number of regulatory T cells in LN was significantly lower than HCs, and the infected patients had the fewest regulatory T cells among all these groups. Regarding localization of infection, the respiratory tract was most frequently involved (69.4%), followed by gastrointestinal (20.8%) and urinary tract infection (12.5%). Concerning pathogens, bacterial infection was more common. Viral included Epstein Barr virus, cytomegalovirus, and respiratory syncytial virus. In training group, the AUC values, accuracy and precision of DT, RF, Ada, and XGB were 1, 100%, and 100%, respectively. As for the modeled effects of predicting LN co-infection in test groups by the eight algorithms, the three algorithms with the highest AUC values were SVM, RF and XGB, with values of 0.85, 0.81 and 0.76, respectively. Among the algorithms, XGB algorithm has the highest accuracy (80%) and precision (84.62%), thus it is recommended to be used for prediction in clinical practice. In addition, the top four weighting factors for infection identified of LN by the XGB in ML were T, red blood cell, hospital length of stay, and lymphocyte%. Conclusion Both innate and adaptive immune systems in LN patients are disturbed, and monitoring the lymphocyte subsets may provide a reference for the prevention and treatment of infection. Our research found that the XGB algorithm performs better than other models with the most accuracy in prediction, which may be one of the preferred algorithms in LN patients with co-infection for future research. Disclosure J. Zhang: None. P. Cai: None. J. Liu: None. Q. Xie: None.
更多查看译文
AI 理解论文
溯源树
样例
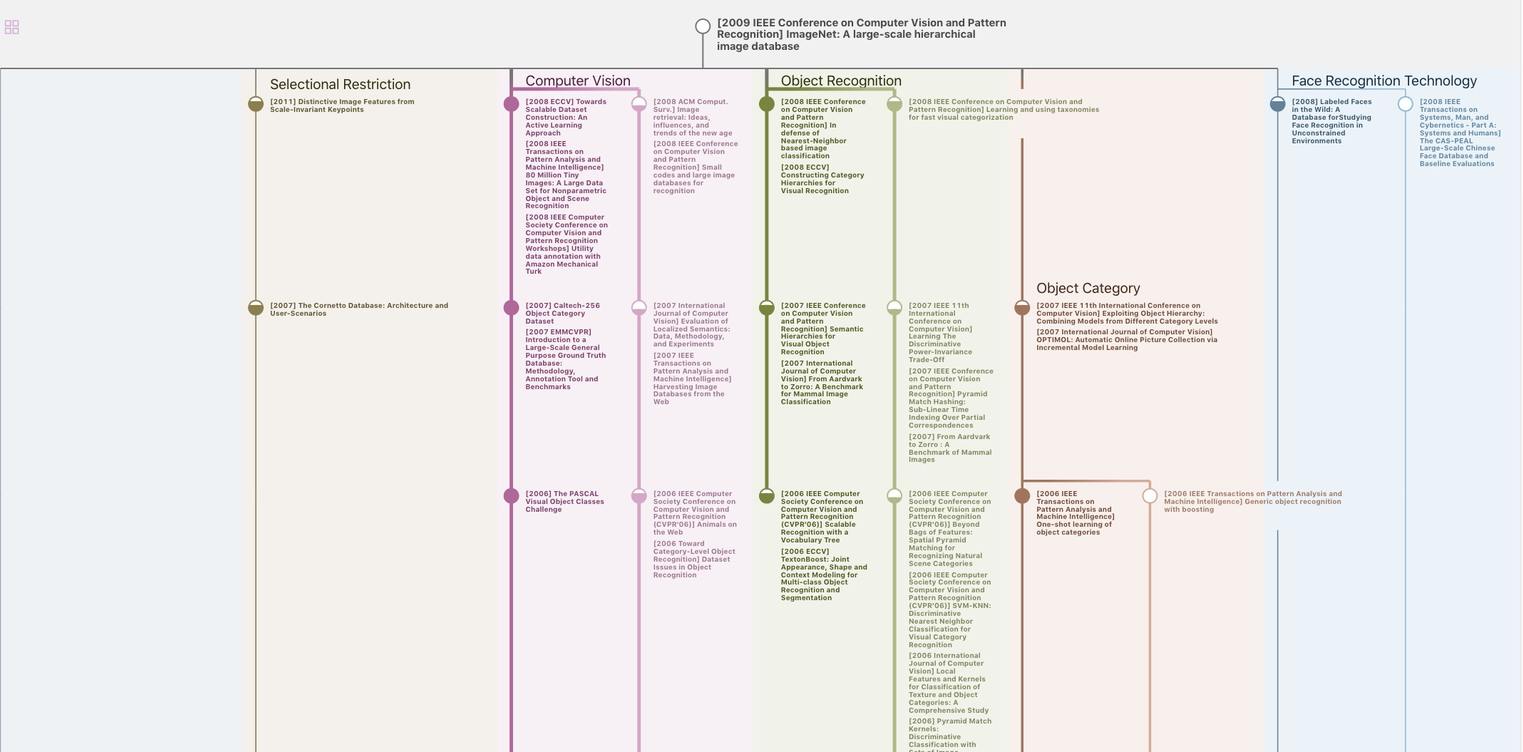
生成溯源树,研究论文发展脉络
Chat Paper
正在生成论文摘要