An Effective Screening of COVID‐19 Pneumonia by Employing Chest X‐ray Segmentation and Attention‐based Ensembled Classification
IET Image Process(2024)
摘要
AbstractQuick and accurate diagnosis of COVID‐19 is crucial in preventing its transmission. Chest X‐ray (CXR) imaging is often used for diagnosis, however, even experienced radiologists may misinterpret the results, necessitating computer‐aided diagnosis. Deep learning has yielded favourable results previously, but overfitting, excessive variance, and generalization errors may occur due to noise and limited datasets. Ensemble learning can improve predictions by using robust techniques. Therefore, this study, proposes two‐fold strategy that combines advanced and robust algorithms, including DenseNet201, EfficientNetB7, and Xception, to achieve faster and more accurate COVID‐19 detection. Segmented lung images were generated from CXR images using the residual U‐Net model, and two attention‐based ensemble neural networks were used for classification. The COVID‐19 radiography dataset was used to evaluate the proposed approach, which achieved an accuracy of 98.21%, 93.4%, and 89.06% for two, three, and four classes respectively which outperformed previous studies by a significant margin considering COVID, viral pneumonia, and lung opacity simultaneously. Despite the similarity in CXR images of COVID, pneumonia, and lung opacity, the proposed approach achieved 89.06% accuracy, demonstrating its ability to recognize distinguishable features. The developed algorithm is expected to have applications in clinics for diagnosing different diseases using X‐ray images.
更多查看译文
关键词
chest X-ray,DenseNet201,EfficientNetB7,ensemble learning,segmentation,Xception
AI 理解论文
溯源树
样例
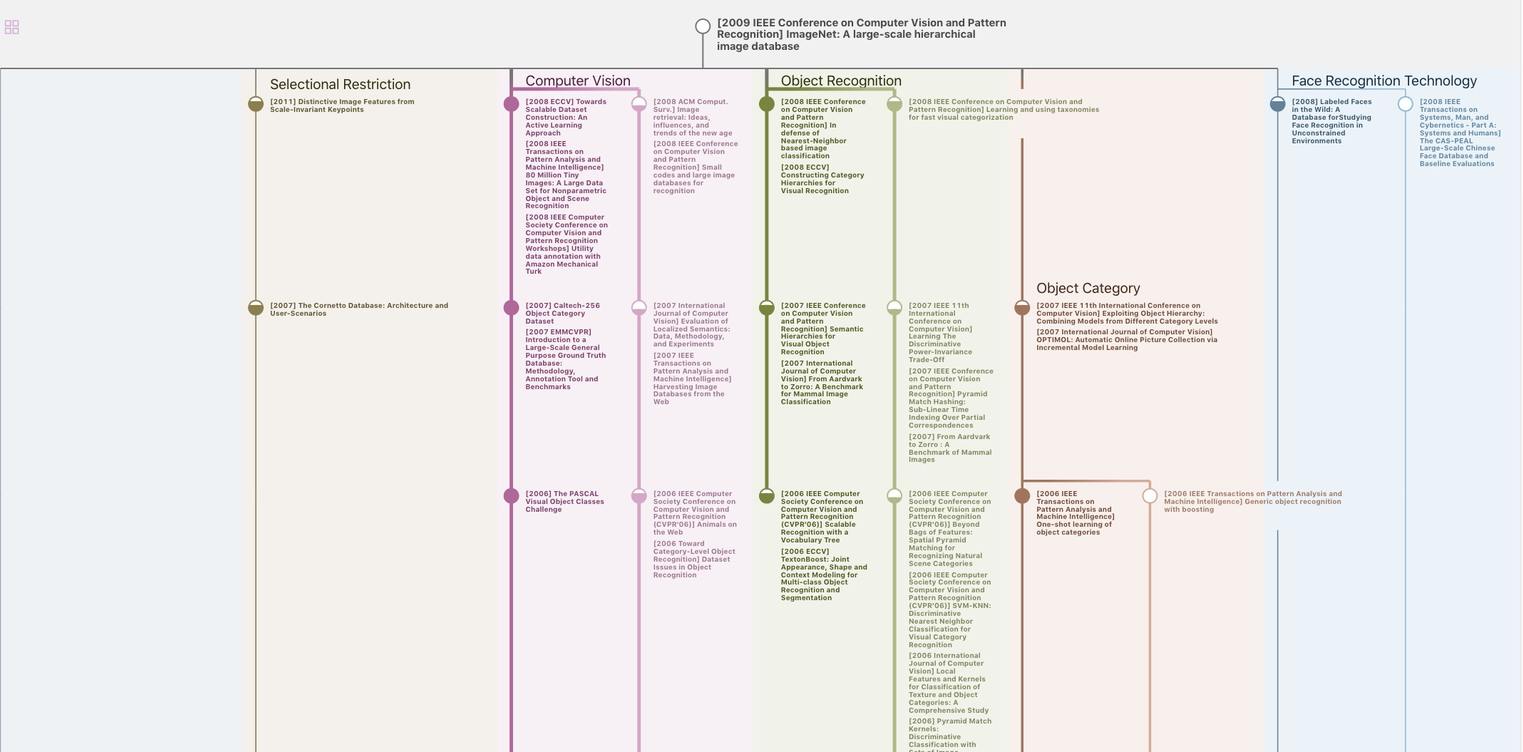
生成溯源树,研究论文发展脉络
Chat Paper
正在生成论文摘要