MAST-GCN: Multi-Scale Adaptive Spatial-Temporal Graph Convolutional Network for EEG-Based Depression Recognition
IEEE Transactions on Affective Computing(2024)
摘要
Recently, depression recognition through EEG has gained significant attention. However, two challenges have not been properly addressed in prior automated depression recognition and classification studies: (1) EEG data lacks an explicit topological structure. (2) Capturing spatio-temporal features of EEG signals is difficult. In this paper, we propose Multi-scale Adaptive Spatial-Temporal Graph Convolutional Network (MAST-GCN) for mining latent topological structure among EEG channels and capturing discriminative spatio-temporal features. First, we integrate Adaptive Graph Convolution (AGC) that merges the inherent graph construction method with a data-driven graph reconstruction method. The model uses attention mechanism to learn an adaptive topological structure and semantic information from different layers and classes. Second, we propose Multi-Scale Time Convolutional Layer (MS-TCL), which captures long-term dependence from EEG data. Since Graph Convolution is weak for aggregating the spatio-temporal information, we have implemented a 3D Graph Convolution (G3D) to directly capture the spatio-temporal dependencies by reconstructing the spatio-temporal graph. The experimental results demonstrate that MAST-GCN consistently outperforms state-of-the-art methods on two datasets. Furthermore, we use the gradient-based saliency maps for interpretability analysis, discovering the active brain regions and important electrode pairs related to depression.
更多查看译文
关键词
Depression Recognition,EEG,Graph Convolutional Network,Attention
AI 理解论文
溯源树
样例
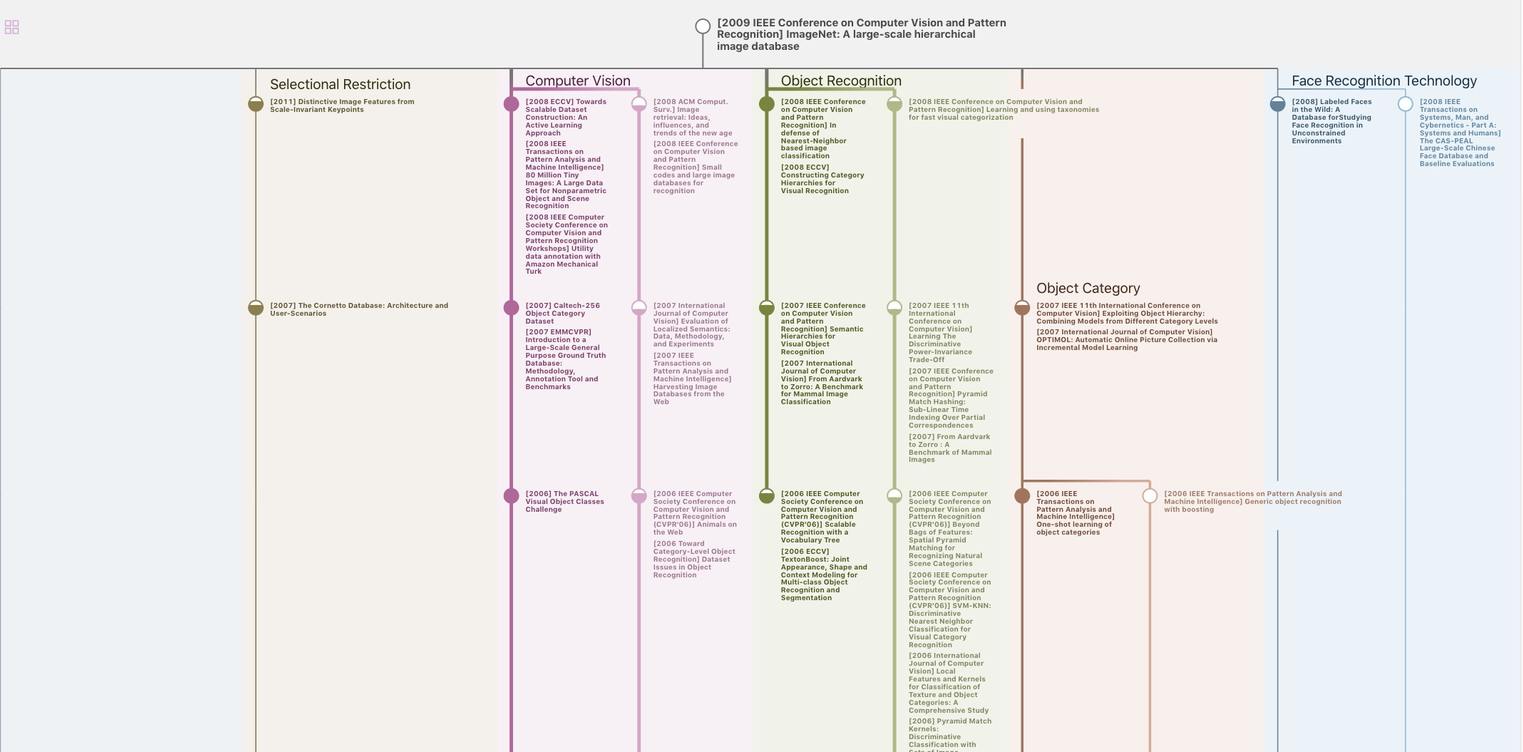
生成溯源树,研究论文发展脉络
Chat Paper
正在生成论文摘要