External Correlates of Adult Digital Problem-Solving Process
ZEITSCHRIFT FUR PSYCHOLOGIE-JOURNAL OF PSYCHOLOGY(2024)
摘要
Computerized assessments and interactive simulation tasks are increasingly popular and afford the collection of process data, i.e., an examinee's sequence of actions (e.g., clickstreams, keystrokes) that arises from interactions with each task. Action sequence data contain rich information on the problem-solving process but are in a nonstandard, variable-length discrete sequence format. Two methods that directly extract features from the raw action sequences, namely multidimensional scaling and sequence-to-sequence autoencoders, produce multidimensional numerical features that summarize original sequence information. This study explores the utility of action sequence features in understanding how problem-solving behavior relates to cognitive proficiencies and demographic characteristics. This is empirically illustrated with the process data from the 2012 PIAAC PSTRE digital assessment. Regularized regression results showed that action sequence features are more predictive of examinees' demographic and cognitive characteristics compared to final outcomes. Partial least squares analysis further aided the identification of behavioral patterns systematically associated with demographic/cognitive characteristics.
更多查看译文
关键词
process data,sequence analysis,computerized assessment,multidimensional scaling,autoencoder
AI 理解论文
溯源树
样例
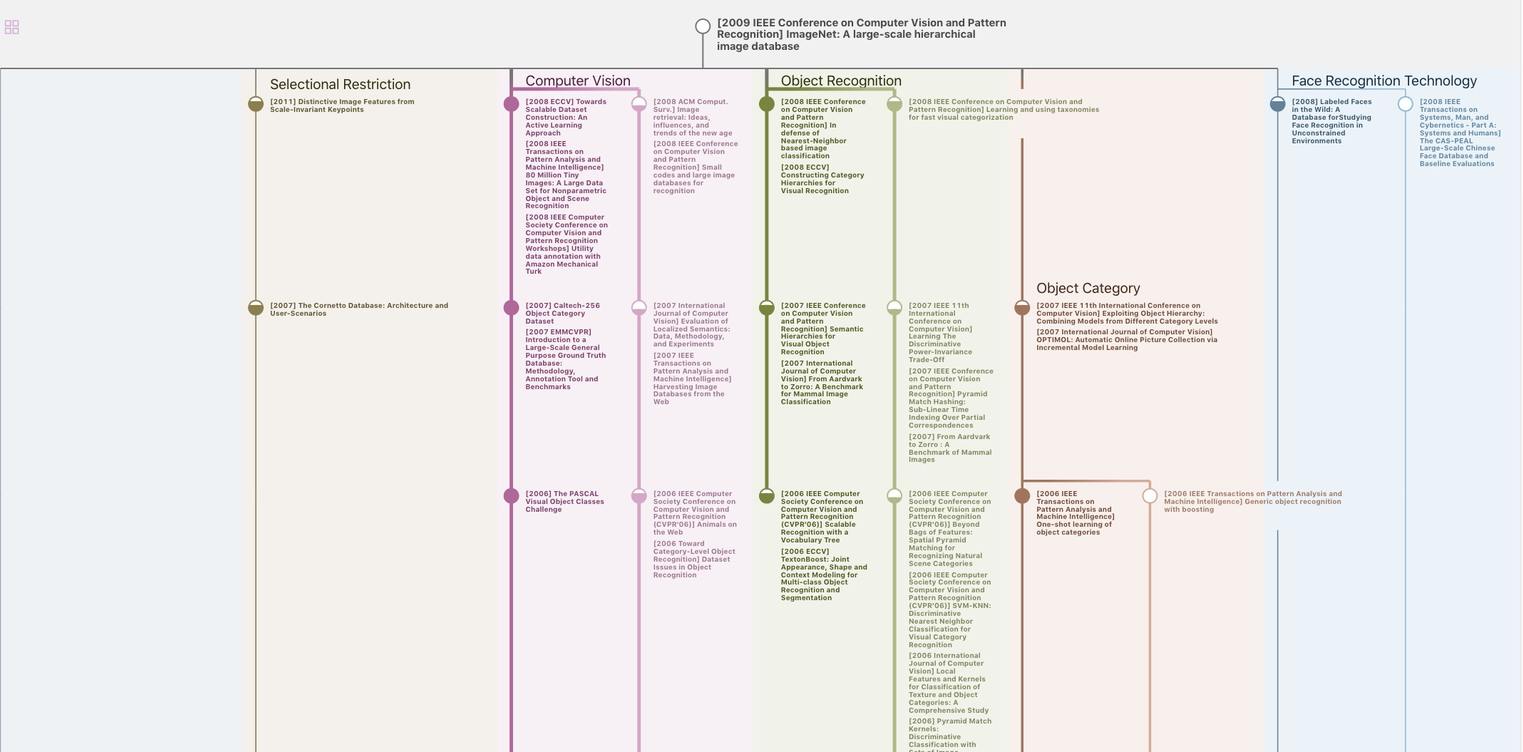
生成溯源树,研究论文发展脉络
Chat Paper
正在生成论文摘要