Progressive generative adversarial network for generating high-dimensional and wide-frequency signals in intelligent fault diagnosis
Engineering Applications of Artificial Intelligence(2024)
摘要
Imbalance is a typical characteristic of data in the field of intelligent fault diagnosis. As a data augmentation method that both balances data and extends information, the generative adversarial network has aroused widespread concern in the data imbalance problem. However, it still suffers from high difficulty in model training and poor quality of generated samples. To address this issue, this paper proposes a progressive generative adversarial network, which is a strategy able to gradually generate complex signals from low-frequency signals to wide-frequency signals. In the developed network, on the one hand, decomposing signals into different frequency bands explicitly reduces the difficulty of directly generating high-dimensional and wide-frequency signals. On the other hand, different generative models can focus on different frequency bands, avoiding the interference of high-energy signals on the fault feature frequencies. In order to achieve good generative performance, the generators and training method for the progressive generative adversarial network are elaborately designed. The correlation shortcut avoids information suppression due to the poor selection of noise amplitudes, and the focusing shortcut forces the body of the generator to focus on key feature bands. Ultimately, the superiority of the progressive generative network is verified by evaluating the quality of the generated samples on three datasets and applying the generated samples to solve the data imbalance problem.
更多查看译文
关键词
Intelligent fault diagnosis,Imbalanced learning,Few-shot learning,Data augmentation,Generative adversarial network
AI 理解论文
溯源树
样例
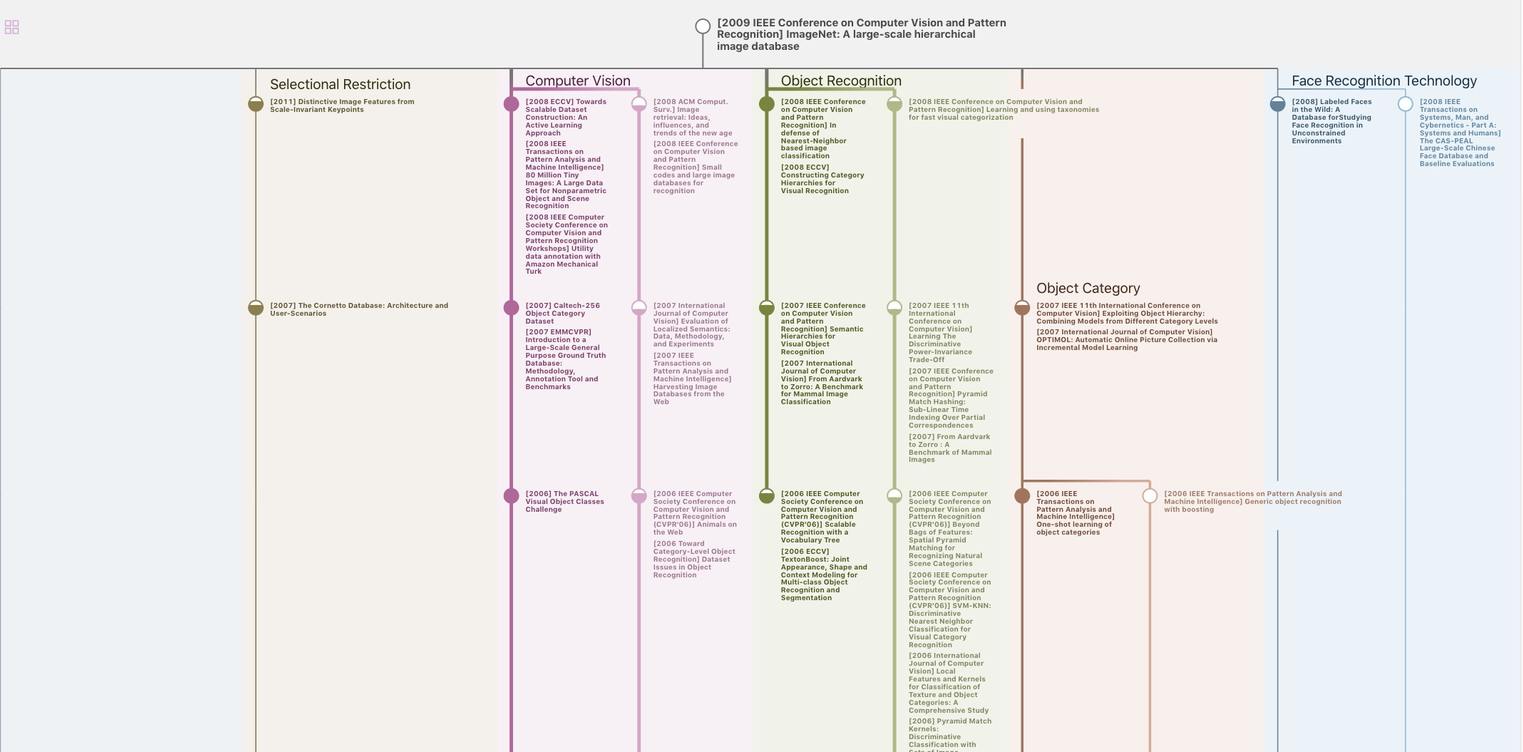
生成溯源树,研究论文发展脉络
Chat Paper
正在生成论文摘要