The Potential of Multi-Task Learning in CFDST Design: Load-Bearing Capacity Design with Three MTL Models.
Materials (Basel, Switzerland)(2024)
摘要
Concrete-filled double steel tubes (CFDSTs) are a load-bearing structure of composite materials. By combining concrete and steel pipes in a nested structure, the performance of the column will be greatly improved. The performance of CFDSTs is closely related to their design. However, existing codes for CFDST design often focus on how to verify the reliability of a design, but specific design parameters cannot be directly provided. As a machine learning technique that can simultaneously learn multiple related tasks, multi-task learning (MTL) has great potential in the structural design of CFDSTs. Based on 227 uniaxial compression cases of CFDSTs collected from the literature, this paper utilized three multi-task models (multi-task Lasso, VSTG, and MLS-SVR) separately to provide multiple parameters for CFDST design. To evaluate the accuracy of models, four statistical indicators were adopted (R2, RMSE, RRMSE, and ρ). The experimental results indicated that there was a non-linear relationship among the parameters of CFDSTs. Nevertheless, MLS-SVR was still able to provide an accurate set of design parameters. The coefficient matrices of two linear models, multi-task Lasso and VSTG, revealed the potential connection among CFDST parameters. The latent-task matrix V in VSTG divided the prediction tasks of inner tube diameter, thickness, strength, and concrete strength into three groups. In addition, the limitations of this study and future work are also summarized. This paper provides new ideas for the design of CFDSTs and the study of related codes.
更多查看译文
关键词
axial compression capacity,circular concrete-filled double-skin steel tube column (CFDST),CFDST design,load-bearing capacity,multi-task learning,multi-task Lasso
AI 理解论文
溯源树
样例
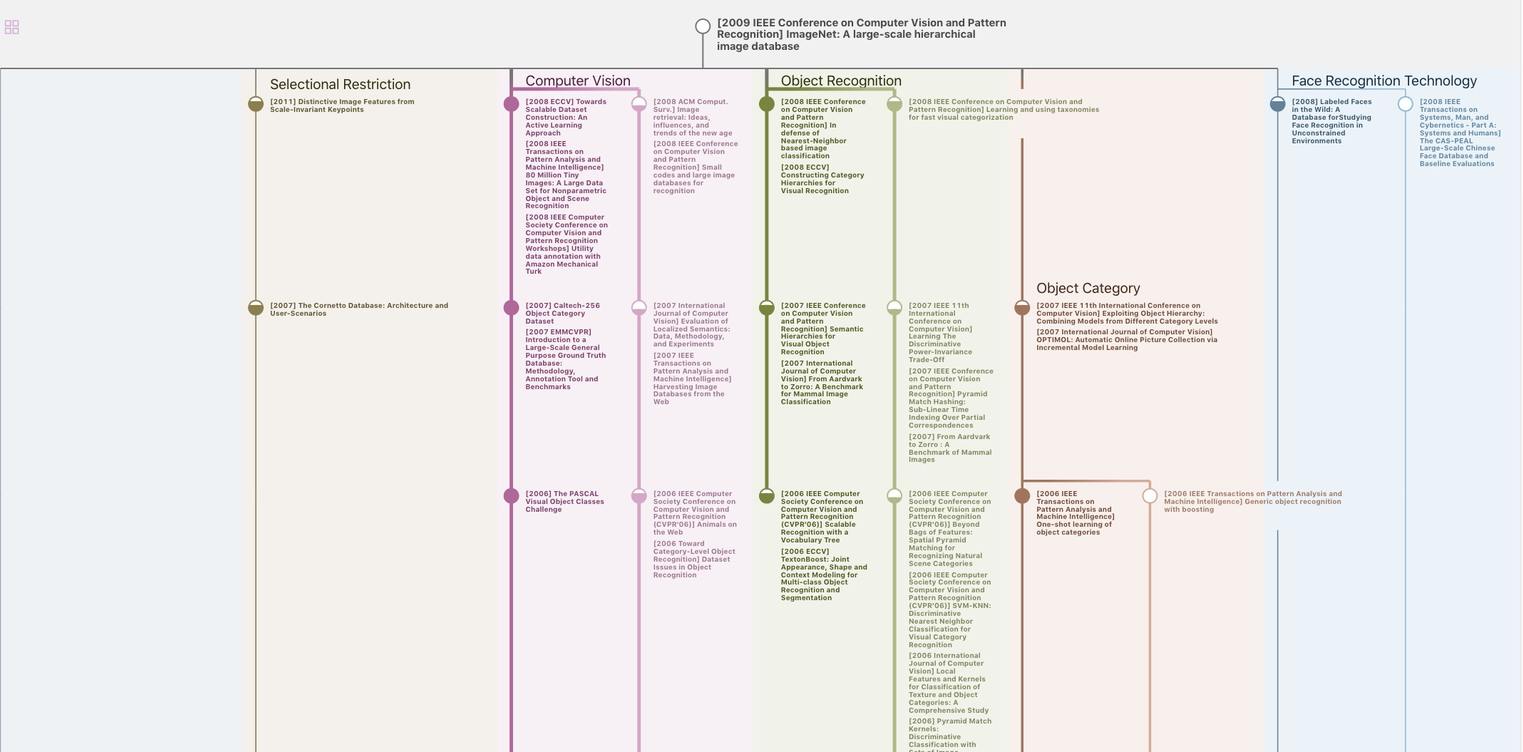
生成溯源树,研究论文发展脉络
Chat Paper
正在生成论文摘要