Deep Learning Approach for Quantifying Abdominal Adipose Tissue in Low dose CT Examination
Korean Society of Computed Tomographic Technology(2024)
摘要
As the obese population increases, computed tomography (CT) tests that can quantitatively measure abdominal obesity have been applied in various studies. To reduce the burden of radiation exposure in CT examinations, various image reconstruction methods such as filtered back projection (FBP) and iterative reconstruction (IR) have been developed, and deep learning iterative reconstruction (DLIR) techniques combined with artificial intelligence have recently emerged. We applied FBP, Adaptive Statistical Iterative Reconstruction-V (ASIR-V) at 30%, DLIR Low, Medium, and High - to low-dose CT images using only 20% of the standard abdominal examination protocols. We compared the measured fat area, total amount, and muscle mass, and evaluated them based on the images applying ASIR-V at 30% to the standard protocols. As a result of the study, the fat area and the amount of fat in the test group decreased by approximately 3~4% in VFA and VFV, and up to about 8% decreased in SFA and SFV in low-dose protocol images applying FBP and ASIR-V 30%. In addition, muscle mass also decreased by 3~6% in image reconstruction images, excluding DLIR. In the case of DLIR, there was either no difference in all items or a slight difference of less than 2%. Image reconstruction methods aimed at reducing noise may impact the results of follow up fat measurement tests. Therefore, the same inspection protocol and reconstruction methods should be applied for accurate evaluation.
更多查看译文
AI 理解论文
溯源树
样例
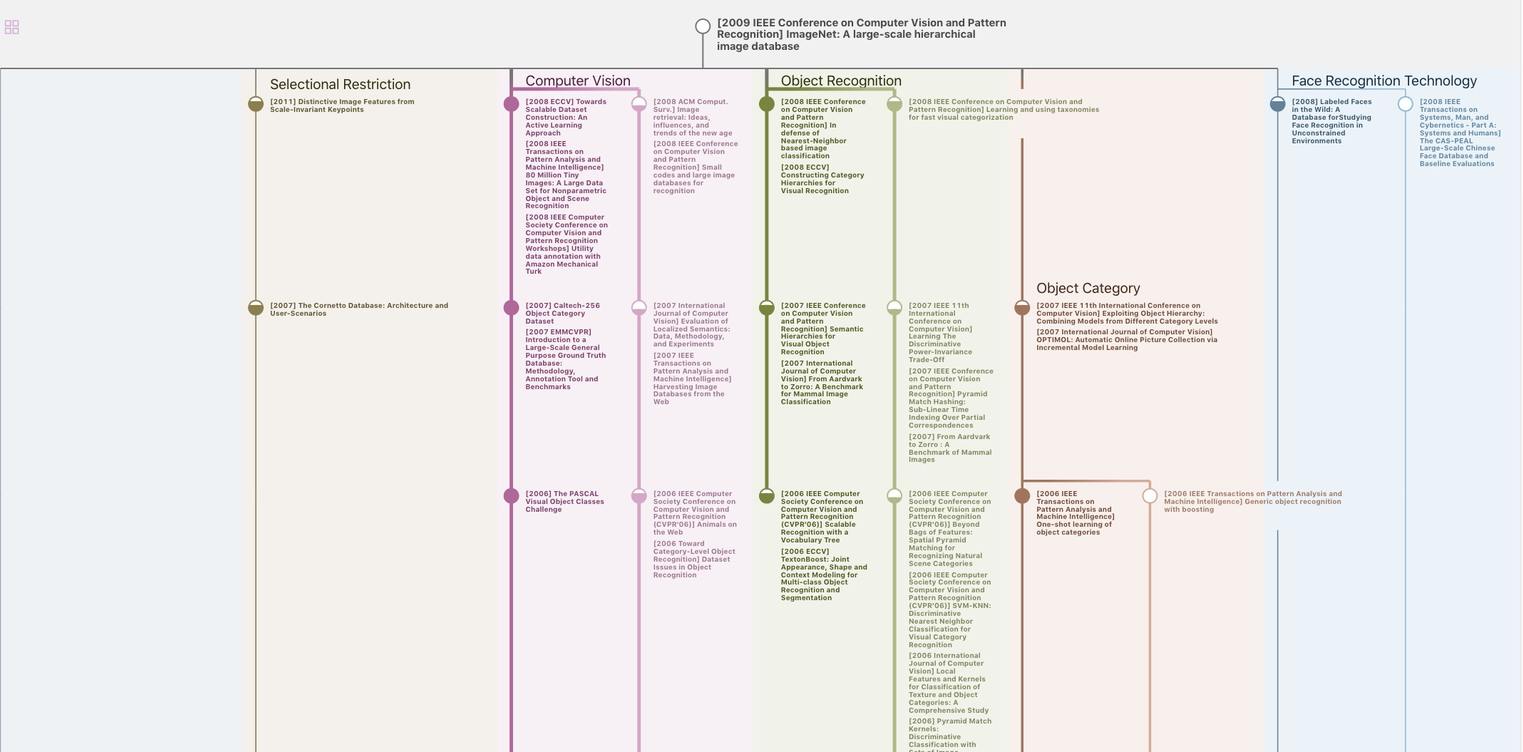
生成溯源树,研究论文发展脉络
Chat Paper
正在生成论文摘要