Online Streaming Feature Selection Based on Label Group Correlation and Neighborhood Rough Set
2023 13th International Conference on Information Technology in Medicine and Education (ITME)(2023)
摘要
Most of the existing multi-label feature selection algorithms select features based on the condition that the feature space is statically known., which may not align well with real-world application scenarios. In this paper., we propose a novel method called label group correlation based online streaming feature selection (LGOFS)., inspired by the neighborhood rough set. Specifically., LGOFS starts by dividing the label set into multiple label groups based on the similarity relationship between labels. Labels within the same group exhibit high correlation., whereas the labels from different groups are relatively weak correlation. Then., neighborhood rough set model., specifically tailored for multi-label learning., is reconstructed based on label correlation. Following this., the importance of features is evaluated using a reconstructed feature dependency function. Finally., we build a streaming feature selection strategy., which includes online importance selection and online subset updating. The experiment results demonstrate that the proposed LGOFS algorithm outperforms several popular algorithms.
更多查看译文
关键词
component,Feature selection,Label correlation,Neighborhood rough set,Streaming features
AI 理解论文
溯源树
样例
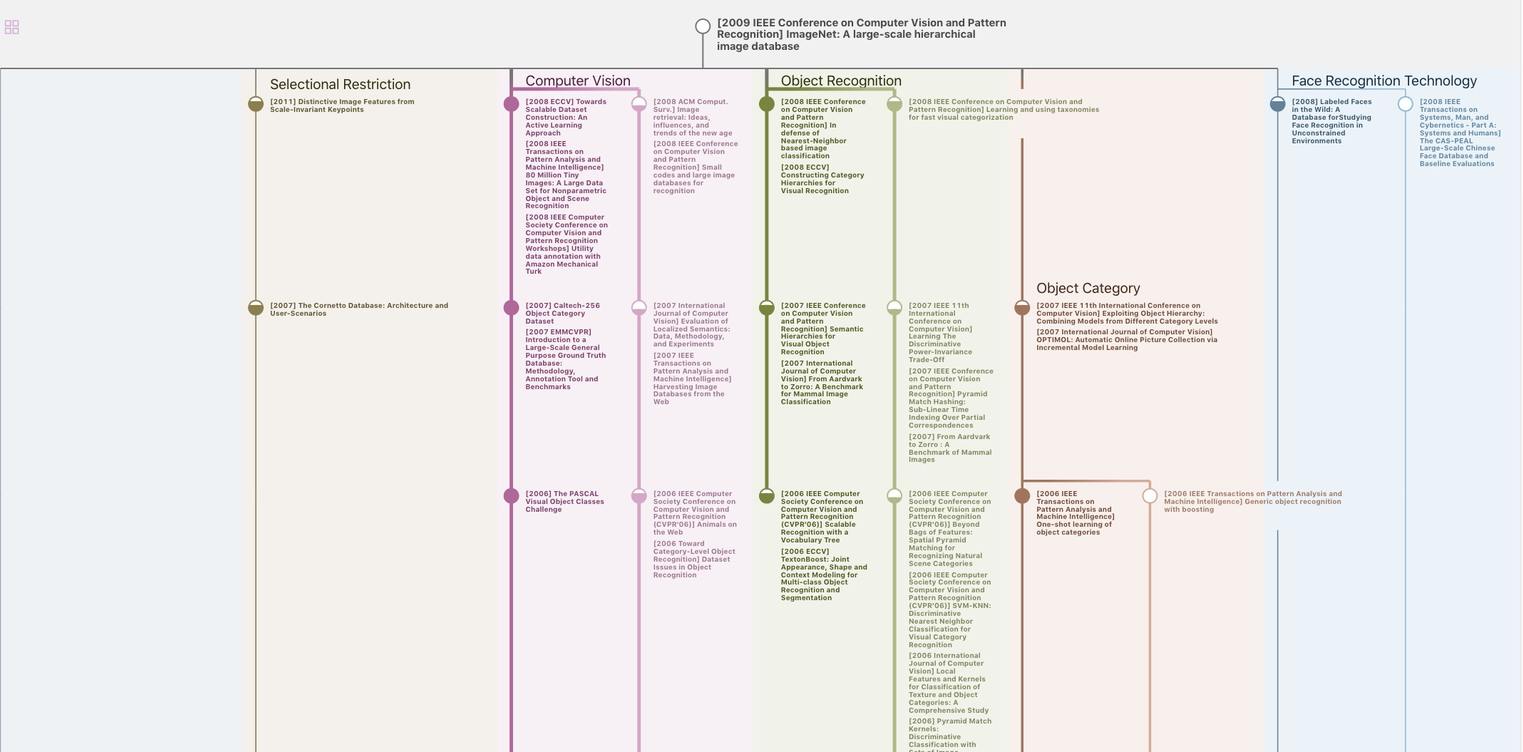
生成溯源树,研究论文发展脉络
Chat Paper
正在生成论文摘要