Intelligent decision-making for binary coverage: Unveiling the potential of the multi-armed bandit selector
Expert Systems with Applications(2024)
摘要
In this article, we propose the integration of a novel reinforcement learning technique into our generic and unified framework. This framework enables any continuous metaheuristic to operate in binary optimization, with the technique in question known as the Multi-Armed Bandit. Population-based metaheuristics comprise multiple individuals that cooperatively and globally explore the search space using their limited individual capabilities. Our framework allows these population-based metaheuristics to continue leveraging their original movements, designed for continuous optimization, once they are binary encoded. The generality of the framework has facilitated the instantiation of popular algorithms from the optimization, machine learning, and evolutionary computing communities. Furthermore, it permits the design of new and innovative optimization instances using various component strategies, reflecting the framework’s modularity. The results comparing two statistical techniques and three hybridizations coming from Machine Learning, have shown to obtain a better performance with the metahuristics in Grey Wolf Optimizer and Whale Optimization Algorithm.
更多查看译文
关键词
Binarization schemes selection,Binary optimization,Metaheuristics,Reinforcement learning,Decision making
AI 理解论文
溯源树
样例
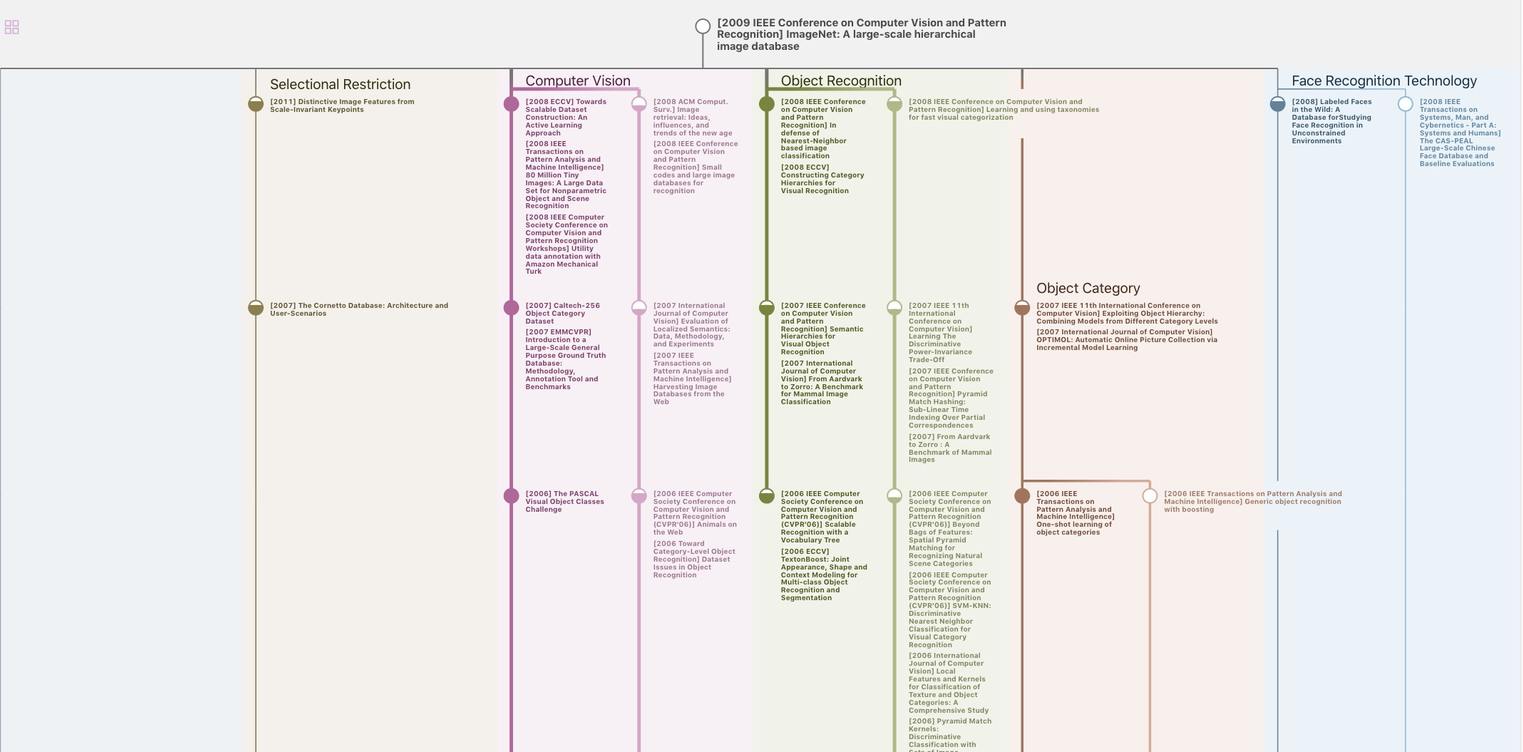
生成溯源树,研究论文发展脉络
Chat Paper
正在生成论文摘要