Sequence can Secretly Tell You What to Discard
arxiv(2024)
摘要
Large Language Models (LLMs), despite their impressive performance on a wide
range of tasks, require significant GPU memory and consume substantial
computational resources. In addition to model weights, the memory occupied by
KV cache increases linearly with sequence length, becoming a main bottleneck
for inference. In this paper, we introduce a novel approach for optimizing the
KV cache which significantly reduces its memory footprint. Through a
comprehensive investigation, we find that on LLaMA2 series models, (i) the
similarity between adjacent tokens' query vectors is remarkably high, and (ii)
current query's attention calculation can rely solely on the attention
information of a small portion of the preceding queries. Based on these
observations, we propose CORM, a KV cache eviction policy that dynamically
retains important key-value pairs for inference without finetuning the model.
We validate that CORM reduces the inference memory usage of KV cache by up to
70
更多查看译文
AI 理解论文
溯源树
样例
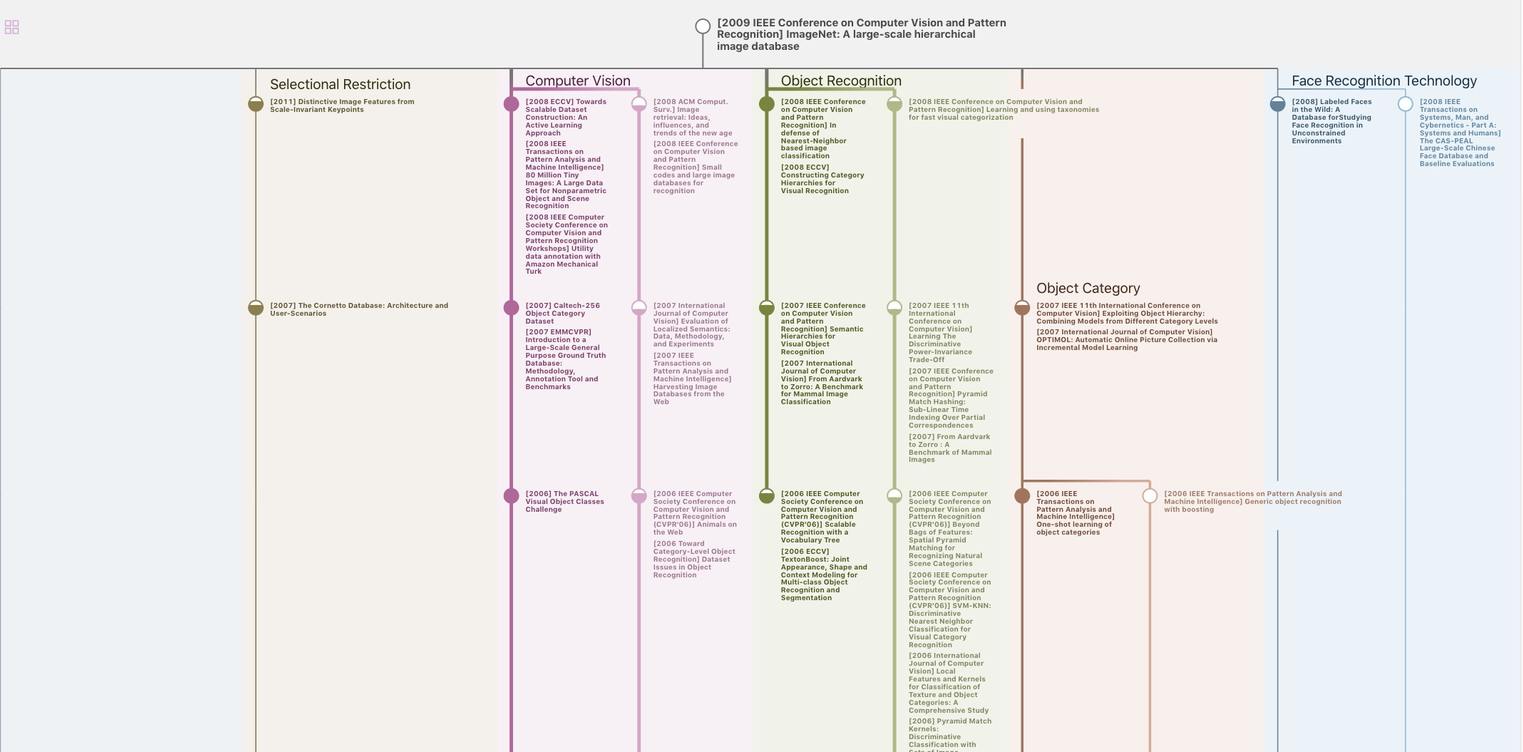
生成溯源树,研究论文发展脉络
Chat Paper
正在生成论文摘要