Research on Meta-Learning Based Few-Shot Chinese Text Classification Algorithms
2023 5th International Academic Exchange Conference on Science and Technology Innovation (IAECST)(2023)
摘要
Current text classification methods face limitations due to dataset size, hindering fast learning and generalization in few-shot scenarios. Our proposed method, Reptile-MAM, transforms multiclassification tasks into binary ones with limited source domain data. Using a task-agnostic MacBert model, we extract generic features and employ the lightweight fine-tuning method MAM for rapid adaptation to new tasks. Classification training, based on sentence pair implication relationships, completes parameter updates for the lightweight model. Reptile is used for initial parameter learning, enhancing generalization on new tasks by aggregating weighted updates. Validation on Chinese few-shot learning problems using public datasets demonstrates superior performance compared to previous methods, highlighting the effectiveness of our approach, especially with the integration of lightweight fine-tuning.
更多查看译文
关键词
few-shot learning,meta-learning,text classification
AI 理解论文
溯源树
样例
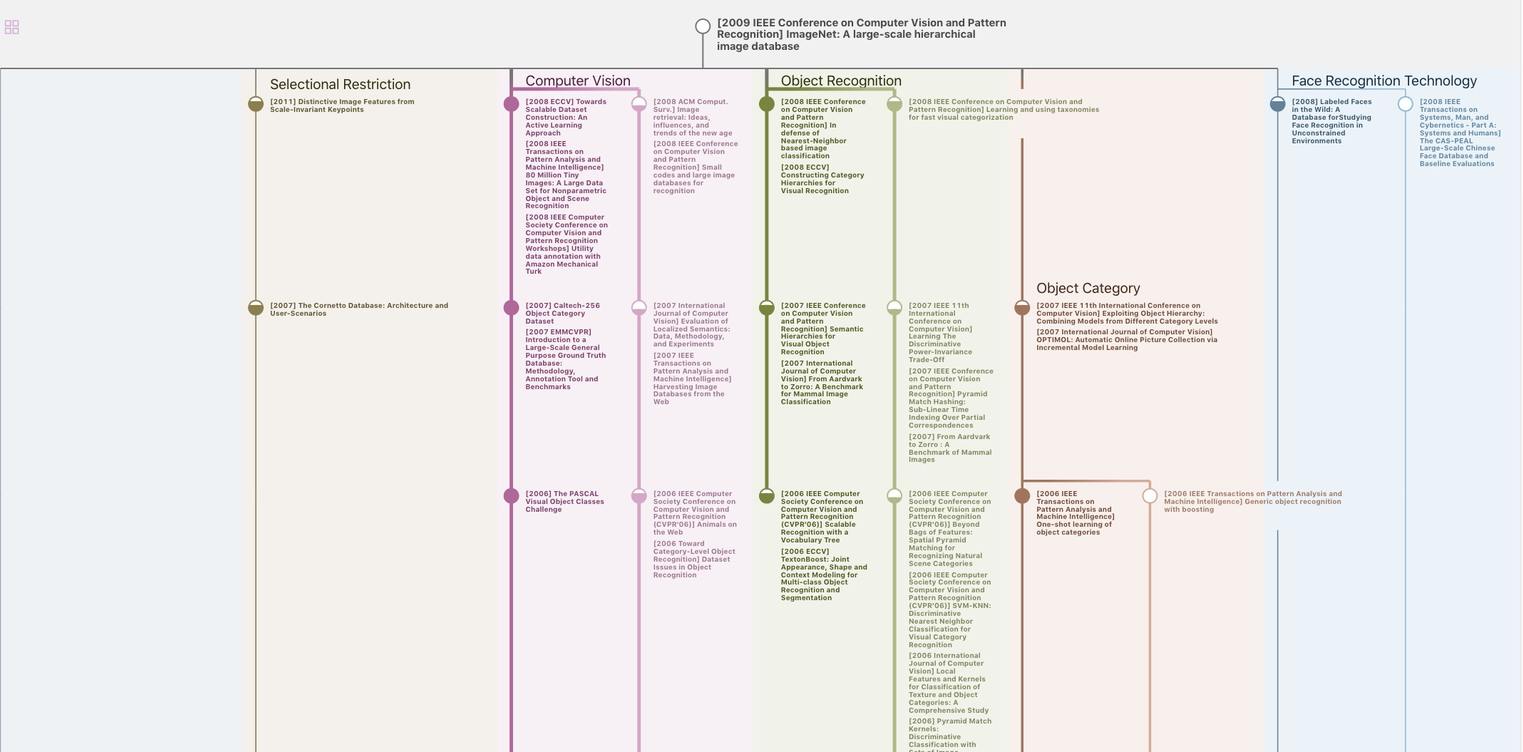
生成溯源树,研究论文发展脉络
Chat Paper
正在生成论文摘要