Optimal depth and a novel approach to variational quantum process tomography
arxiv(2024)
摘要
In this work, we present two new methods for Variational Quantum Circuit
(VQC) Process Tomography onto n qubits systems: PT_VQC and U-VQSVD.
Compared to the state of the art, PT_VQC halves in each run the required
amount of qubits for process tomography and decreases the required state
initializations from 4^n to just 2^n, all while ensuring high-fidelity
reconstruction of the targeted unitary channel U. It is worth noting that,
for a fixed reconstruction accuracy, PT_VQC achieves faster convergence per
iteration step compared to Quantum Deep Neural Network (QDNN) and tensor
network schemes.
The novel U-VQSVD algorithm utilizes variational singular value decomposition
to extract eigenvectors (up to a global phase) and their associated eigenvalues
from an unknown unitary representing a general channel. We assess the
performance of U-VQSVD by executing an attack on a non-unitary channel Quantum
Physical Unclonable Function (QPUF). U-VQSVD outperforms an uninformed
impersonation attack (using randomly generated input states) by a factor of 2
to 5, depending on the qubit dimension.
For the two presented methods, we propose a new approach to calculate the
complexity of the displayed VQC, based on what we denote as optimal depth.
更多查看译文
AI 理解论文
溯源树
样例
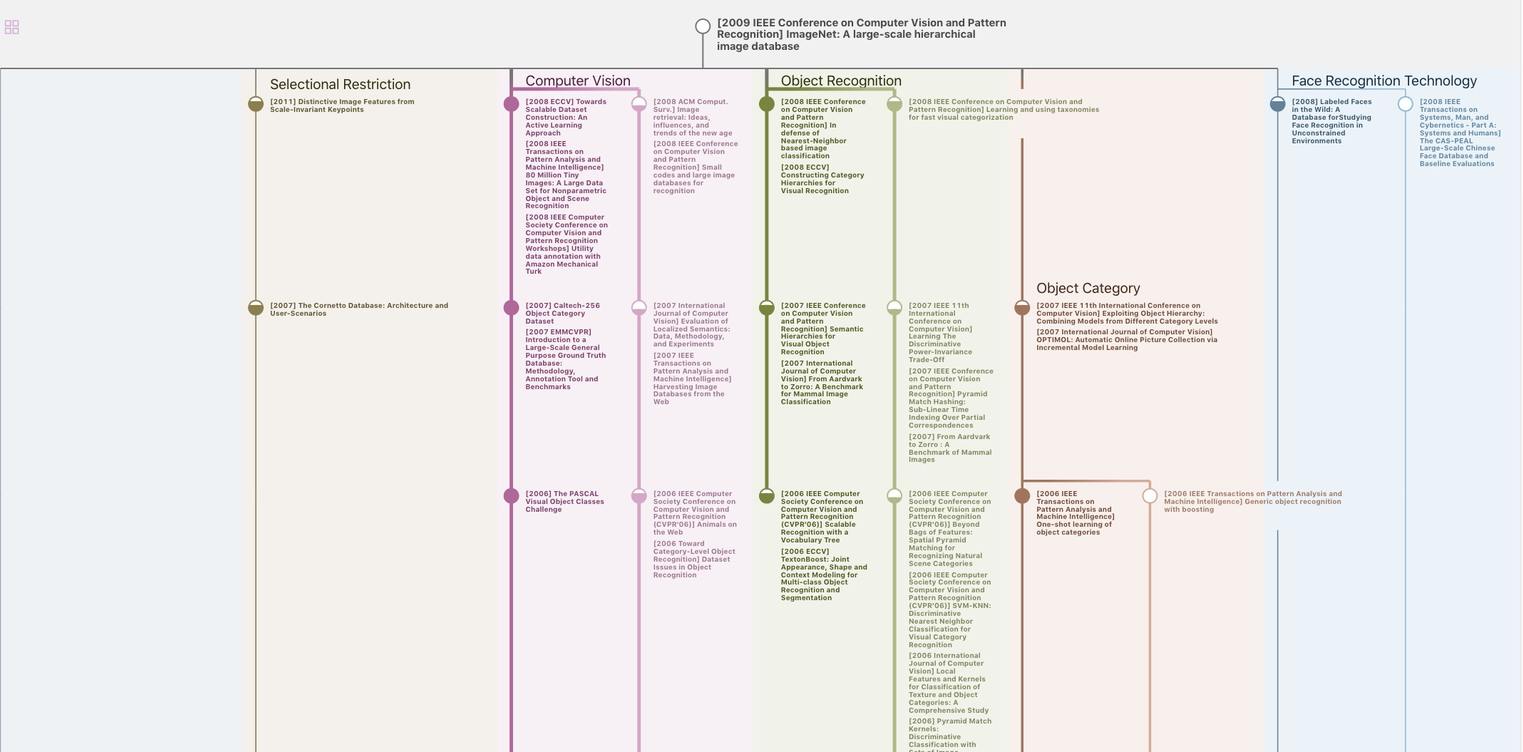
生成溯源树,研究论文发展脉络
Chat Paper
正在生成论文摘要