One-shot neuroanatomy segmentation through online data augmentation and confidence aware pseudo label
Medical Image Analysis(2024)
摘要
Recently, deep learning-based brain segmentation methods have achieved great success. However, most approaches focus on supervised segmentation, which requires many high-quality labeled images. In this paper, we pay attention to one-shot segmentation, aiming to learn from one labeled image and a few unlabeled images. We propose an end-to-end unified network that joints deformation modeling and segmentation tasks. Our network consists of a shared encoder, a deformation modeling head, and a segmentation head. In the training phase, the atlas and unlabeled images are input to the encoder to get the multi-scale features. The features are then fed to the multi-scale deformation modeling module to estimate the atlas-to-image deformation field. The deformation modeling module implements the estimation at the feature level in a coarse-to-fine manner. Then, we employ the field to generate the augmented image pair through online data augmentation. We do not apply any appearance transformations cause the shared encoder could capture appearance variations. Finally, we adopt supervised segmentation loss for the augmented image. Considering that the unlabeled images still contain rich information, we introduce confidence aware pseudo label for them to further boost the segmentation performance. We validate our network on three benchmark datasets. Experimental results demonstrate that our network significantly outperforms other deep single-atlas-based and traditional multi-atlas-based segmentation methods. Notably, the second dataset is collected from multi-center, and our network still achieved promising segmentation performance on both the seen and unseen test set, revealing its robustness. The source code will be available at https://github.com/zhangliutong/brainseg.
更多查看译文
关键词
One-shot learning,Neuroanatomy segmentation,Data augmentation,Deformation modeling
AI 理解论文
溯源树
样例
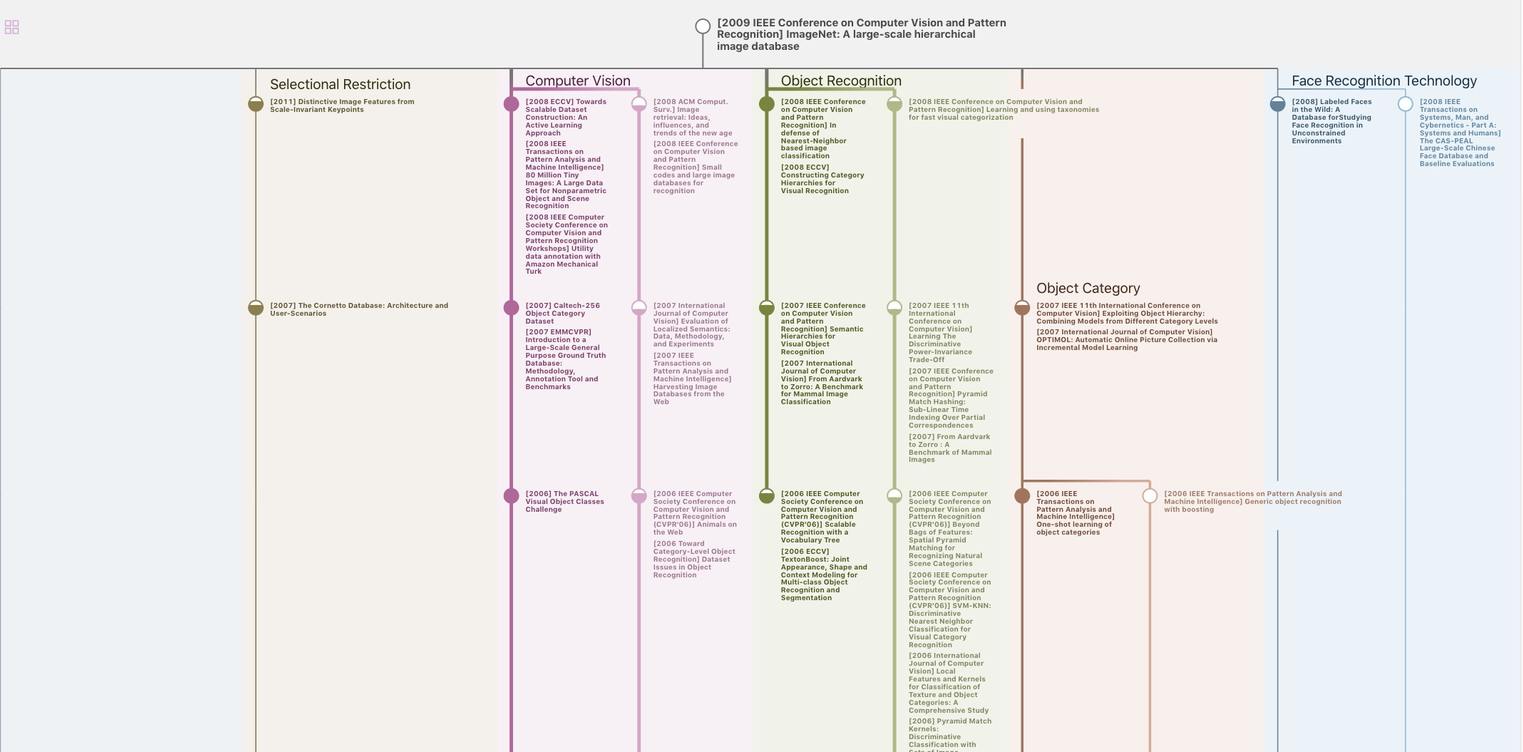
生成溯源树,研究论文发展脉络
Chat Paper
正在生成论文摘要