Operational Risk Assessment of Hydropower Units Based on Pssca-Vmd-Cnn-Gbilstm and Multi-Feature Fusion
Computers and Electrical Engineering(2024)
Abstract
Hydroelectric units are an essential part of hydropower plants nowadays, and assessing and predicting the operating conditions of hydroelectric units is of great significance for the reliable operation of hydropower plants. Firstly, an optimization method based on polynomial regression with Pearson and Spearman fusion and Sine Cosine Algorithm (SCA) algorithm (PSSCA) is proposed to optimize the parameters in the variational modal decomposition (VMD), so as to suppress the influence of noise on the vibration signals of hydroelectric units. Then, the sample entropy and root-mean-square fusion of the VMD decomposed modal functions are reconstructed into a hybrid feature vector set so that this vector set can maximally contain the feature information of the vibration signals of the hydroelectric units. Finally, the CNN-GBiLSTM assessment model is constructed by combining the Convolutional Neural Network (CNN) model, the Bi-directional Long Short-Term Memory (BiLSTM) model, and the global average pooling layer, which is used to predict the upper and lower limits of the healthy vibration of the hydropower unit, and to construct the risk indicator of the hydropower unit operation by combining the values of the upper and lower limits. The results of simulation and comparative experiments show that the method proposed in this study can visually represent the three operational risk scenarios of a hydropower unit: low risk, medium risk, and high risk. It can effectively accomplish the operational risk assessment of hydropower units and improve the assessment accuracy and assessment speed, thus proving the effectiveness and superiority of the method.
MoreTranslated text
Key words
Hydroelectric unit risk assessment,Variational modal decomposition,Pearson and Spearman fusion method polynomial regression model,CNN-GBiLSTM,Hybrid feature set
AI Read Science
Must-Reading Tree
Example
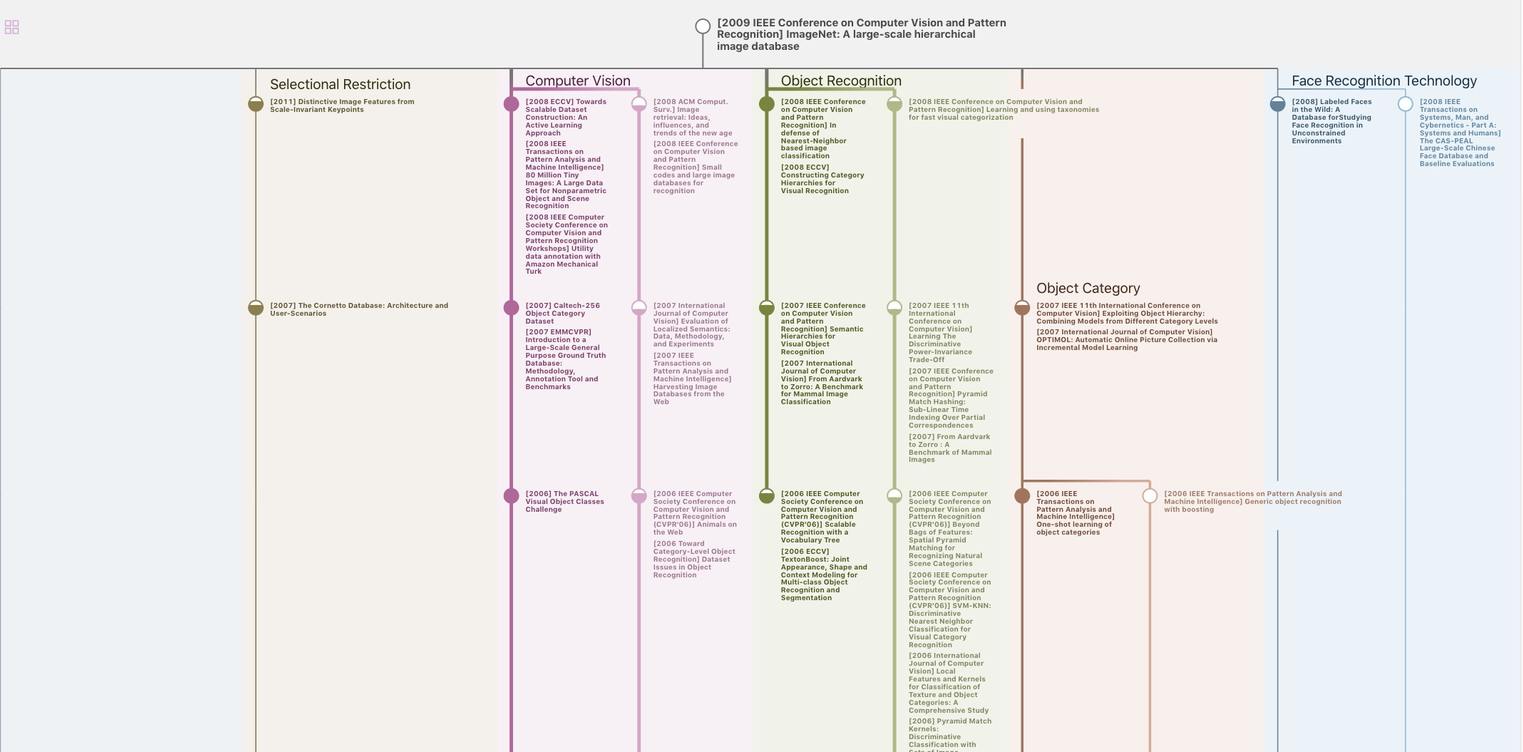
Generate MRT to find the research sequence of this paper
Chat Paper
Summary is being generated by the instructions you defined