Federated Learning for ASR Based on FedEA
2024 IEEE 7th Advanced Information Technology, Electronic and Automation Control Conference (IAEAC)(2024)
摘要
With the advancement and refinement of federated learning, integrating federated learning with speech recognition has spawned innovative ideas and approaches for training speech recognition models. This study presents a novel federated aggregation method, Federated Error Average(FedEA), which fuses federated learning with wav2vec2.0, a renowned self-supervised pre-trained speech recognition model. It features a redesign to enable the aggregation model to address scenarios where malicious users introduce substantial interference data effectively. Experimental assessments were conducted using the 178-hour professional recording Aishell dataset. The results of these experiments demonstrate that an error rate as low as 10.84% can be achieved on the Aishell dataset, even in the absence of malicious user interference and the necessity for a language model. Moreover, when a substantial number of malicious users are introduced to disrupt the data, the innovative FedEA aggregation method can allocate smaller weights to ensure robust convergence and recognition performance of the aggregation model. Remarkably, the experimental outcomes surpass existing mainstream aggregation methods.
更多查看译文
关键词
Federated learning,automatic speech recognition,FedEA,self-supervised models
AI 理解论文
溯源树
样例
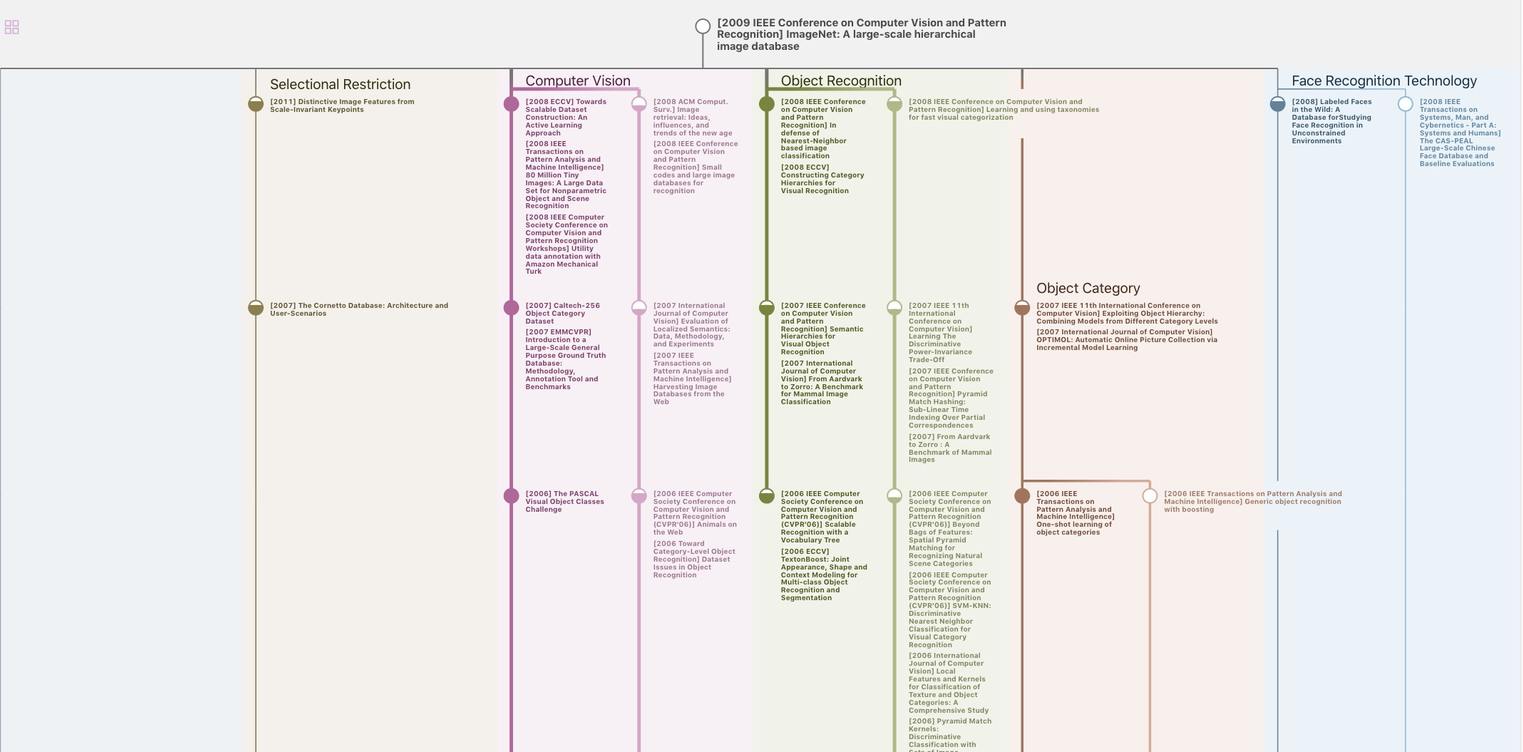
生成溯源树,研究论文发展脉络
Chat Paper
正在生成论文摘要