Research on Terahertz Image Analysis of Thin-Shell Seeds Based on Semantic Segmentation
SPECTROCHIMICA ACTA PART A-MOLECULAR AND BIOMOLECULAR SPECTROSCOPY(2024)
摘要
Assessing crop seed phenotypic traits is essential for breeding innovations and germplasm enhancement. However, the tough outer layers of thin-shelled seeds present significant challenges for traditional methods aimed at the rapid assessment of their internal structures and quality attributes. This study explores the potential of combining terahertz (THz) time-domain spectroscopy and imaging with semantic segmentation models for the rapid and non-destructive examination of these traits. A total of 120 watermelon seed samples from three distinct varieties, were curated in this study, facilitating a comprehensive analysis of both their outer layers and inner kernels. Utilizing a transmission imaging modality, THz spectral images were acquired and subsequently reconstructed employing a correlation coefficient method. Deep learning-based SegNet and DeepLab V3+ models were employed for automatic tissue segmentation. Our research revealed that DeepLab V3+ significantly surpassed SegNet in both speed and accuracy. Specifically, DeepLab V3+ achieved a pixel accuracy of 96.69 % and an intersection over the union of 91.3 % for the outer layer, with the inner kernel results closely following. These results underscore the proficiency of DeepLab V3+ in distinguishing between the seed coat and kernel, thereby furnishing precise phenotypic trait analyses for seeds with thin shells. Moreover, this study accentuates the instrumental role of deep learning technologies in advancing agricultural research and practices.
更多查看译文
关键词
Terahertz spectroscopy imaging,Thin-shelled seed phenotypic traits,Semantic segmentation,SegNet,DeepLab V3+
AI 理解论文
溯源树
样例
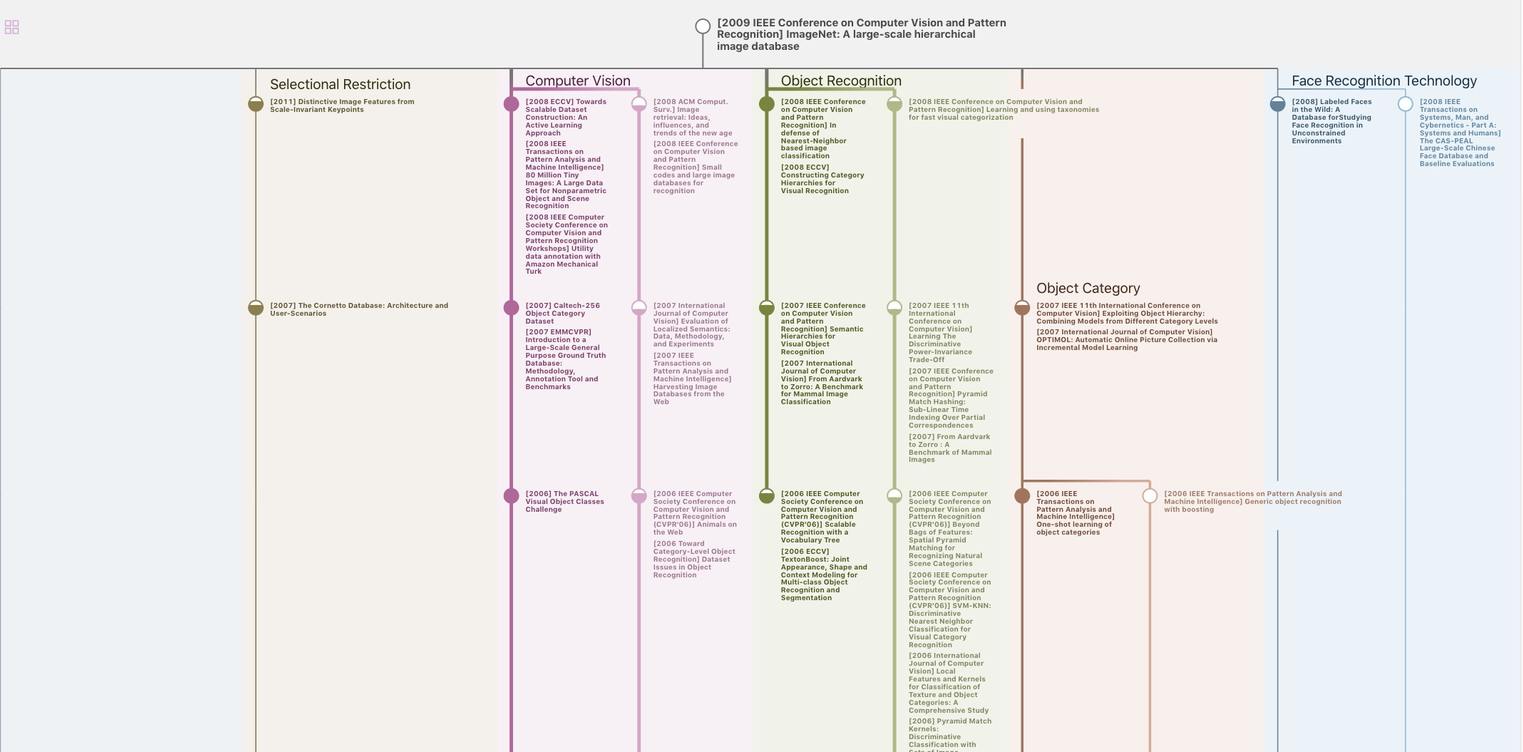
生成溯源树,研究论文发展脉络
Chat Paper
正在生成论文摘要