Data Generation and Augmentation Method for Deep Learning-Based VDU Leakage Signal Restoration Algorithm.
IEEE Trans. Inf. Forensics Secur.(2024)
摘要
This study analyzes the phenomenon of electromagnetic (EM) leakage that occurs through cables and explores the potential for information forensics using deep learning-based image-processing algorithms. We focus on the transition-minimized differential signaling (TMDS) interface to analyze information leakage caused by the inherent differential signal synchronization errors in video graphics controllers (VGC). Our analysis includes detailed mathematical modeling of the EM leakage phenomena from the video display unit (VDU) interface that uses the TMDS protocol. Furthermore, this study presents mathematical models for distortions and alterations caused by the VDU characteristics and its associated RF front-end system. Utilizing mathematical models of EM phenomena, this paper presents a method for creating training datasets for deep learning-based signal processing algorithms by generating and augmenting pseudo leakage signals (PLS) that closely resemble actual leakage signals. This study confirms the practical utility of signal enhancement models trained with generated and augmented PLS in real-world scenarios. Validation involves applying the trained model to measured actual VDU leakage signals and evaluating the results using image quality metrics: peak signal-to-noise ratio (PSNR), signal-to-noise ratio (SNR), and the structural similarity index measure (SSIM). Ultimately, this study demonstrates the potential to develop deep learning models using theoretically generated PLS for VDU-targeted side-channel attacks, where collecting real training data poses a challenge. This suggests the potential for expanding into high-performance deep learning algorithms in future developments.
更多查看译文
关键词
TEMPEST,LCD monitor,information Leakage,Electromagnetic Interference (EMI),Compromising Emanations (CE),deep learning,Convolutional Neural Network (CNN),data augmentation
AI 理解论文
溯源树
样例
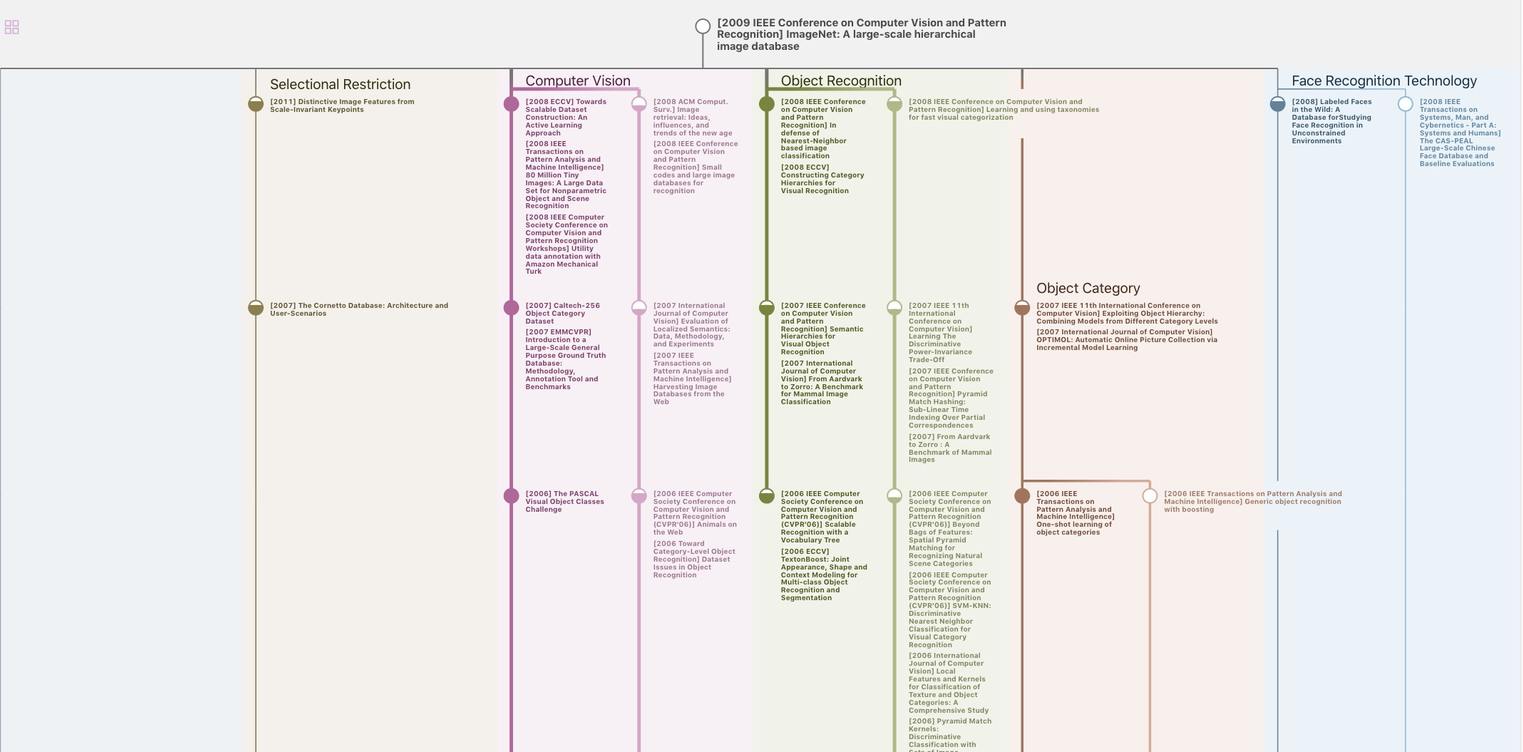
生成溯源树,研究论文发展脉络
Chat Paper
正在生成论文摘要