A fuzzy C-means clustering-based hybrid multivariate time series prediction framework with feature selection
IEEE Transactions on Fuzzy Systems(2024)
摘要
Multivariate time series prediction (MTSP) stands as a significant and challenging frontier in the data science domain, garnering considerable interest among researchers. Extreme learning machine (ELM) has emerged as a popular machine learning algorithm capable of effectively addressing MTSP challenges. However, the high-dimensional and nonlinear nature of prediction information within big data contexts exposes certain limitations in ELM's prediction performance. To address this issue, this paper proposes a hybrid MTSP framework based on fuzzy C-means (FCM) clustering coupled with feature selection. The framework begins with a possibility distribution (PD)-based feature selection algorithm designed to evaluate information quality and describe information uncertainty via multi-source information fusion. Subsequently, a robust FCM algorithm is developed, optimizing the clustering process by incorporating feature differences and neighbor information of samples while employing a multi-metric hybrid strategy to determine cluster numbers. Additionally, an enhanced dual-kernel ELM (EDKELM) network is established to enhance prediction capabilities. The resulting hybrid MTSP framework with feature selection excels in autonomously discovering intrinsic featuremodel connections, exhibiting superior prediction performance, and demonstrating excellent generalization ability. Experimental results using real-world datasets showcase the competitiveness of the proposed framework over existing machine learning prediction models in resolving multivariate prediction challenges.
更多查看译文
关键词
Possibility theory,Fuzzy C-means clustering,Extreme learning machine,Feature selection,Multivariate time series prediction
AI 理解论文
溯源树
样例
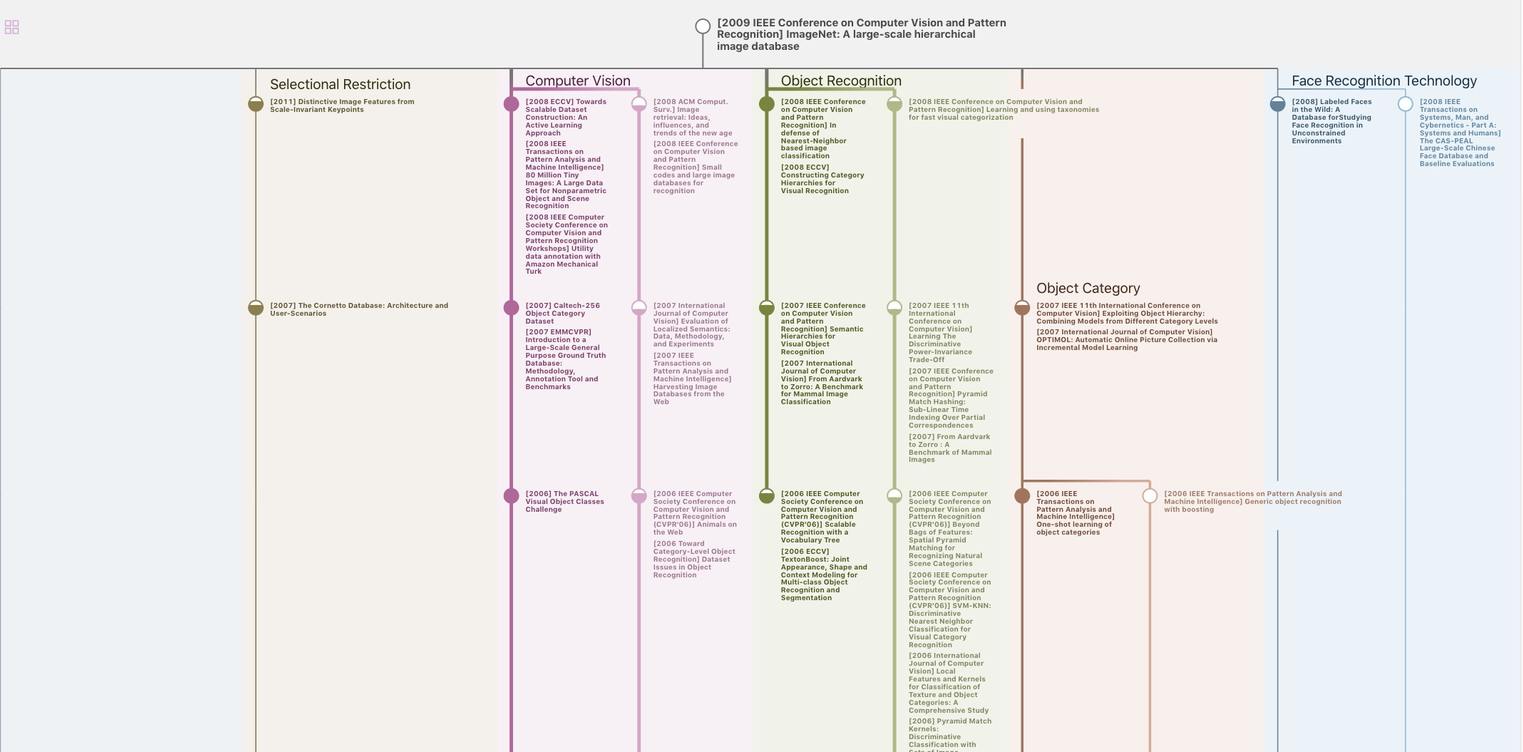
生成溯源树,研究论文发展脉络
Chat Paper
正在生成论文摘要