Temperature-dependent resistance constrained PV accommodation capacity improvement based on Multi-objective DRL
IEEE Transactions on Sustainable Energy(2024)
摘要
Against the backdrop of the low-carbon energy transition, distribution system operators face the urgent challenge of balancing the contradictory demands of high photovoltaic (PV) accommodation capacity and low operation cost. Meanwhile, most iteration-based PV accommodation capacity improvement methods are limited by imprecise line resistance and the conflicting relationship between decision efficiency and modeling accuracy. To this end, a two-timescale distribution network dispatching approach based on muti-objective DRL is proposed. This approach is an online decision-making method based on real-time data and robust to temperature-dependent resistance via constructing a two-stage decision-making model based on multi-objective Markov decision process considering the weather factors. Also, the proposed model has a vectorized reward function to assess the trade-off between the economy and accommodation capacity for better operation. A novel multi-objective DRL (MODRL) algorithm based on the tchebycheff norm is proposed, which decomposes the proposed decision-making model into multiple sub-models for learning Pareto optimal policies. Comparative tests on the IEEE 33-bus system validate that the proposed method effectively acquires optimization strategies under varying user preferences to improve economic and PV accommodation capacity. The proposed algorithm obtains more diverse Pareto fronts and high-quality solutions than other state-of-the-art MODRLs.
更多查看译文
关键词
Distribution network reconfiguration,reactive power optimization,multi-objective optimization,deep reinforcement learning,photovoltaic
AI 理解论文
溯源树
样例
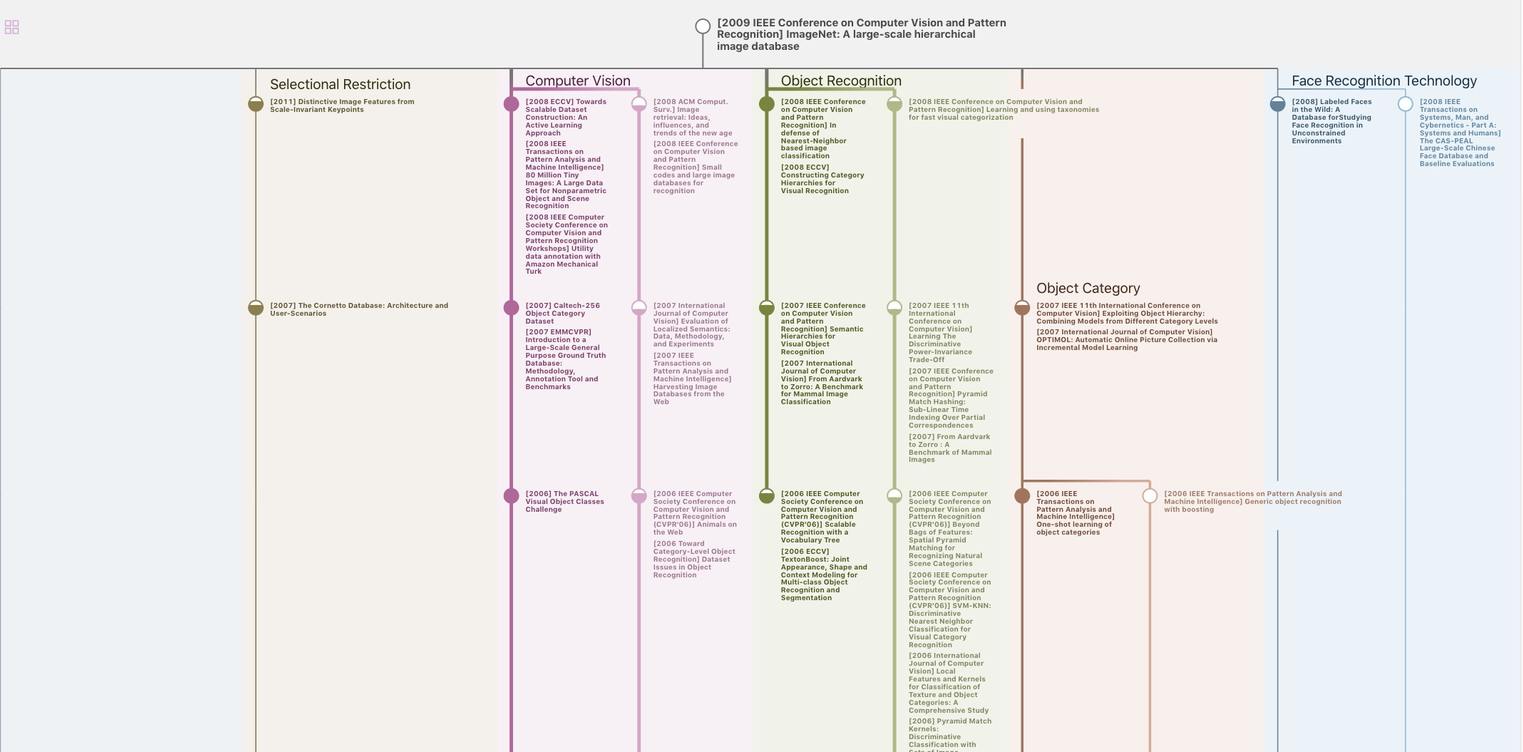
生成溯源树,研究论文发展脉络
Chat Paper
正在生成论文摘要