Arbitrary-Scale Image Super-Resolution via Degradation Perception
IEEE Transactions on Computational Imaging(2024)
摘要
In recent years, with the rapid development of deep learning, super-resolution research oriented towards arbitrary scale (e.g., arbitrary integer and non-integer scale factors) factors has achieved great success. However, in terms of pixel space, the degradation in the same image at arbitrary scale factors is spatially variable. Similarly, the degradation is variable for different scale factors. In this paper, we propose a method that can adaptively deal with varying degradation at different scale factors, which consists of two parts. The first part, Image Refinement Network (IRN), adopts a dynamic convolution method to deal with different degradations under arbitrary scale factors on a pixel-by-pixel basis. It solves the spatial invariance problem of the ordinary convolution kernel. For well calculating the pixel mapping relationships that change during the super-resolution of arbitary scale factors, we propose a second Module, Super-Resolution Encoding Guidance Module (SREGM). It takes the high-resolution pixel space as a reference frame and uses the modelling results as prior information to better guide the high-resolution reconstruction. Extensive experiments have shown that our method achieves good results in the super-resolution of a single image with an arbitrary scale factor.
更多查看译文
关键词
Super-resolution,arbitrary scale,super-resolution encoding guidance module,image refinement network
AI 理解论文
溯源树
样例
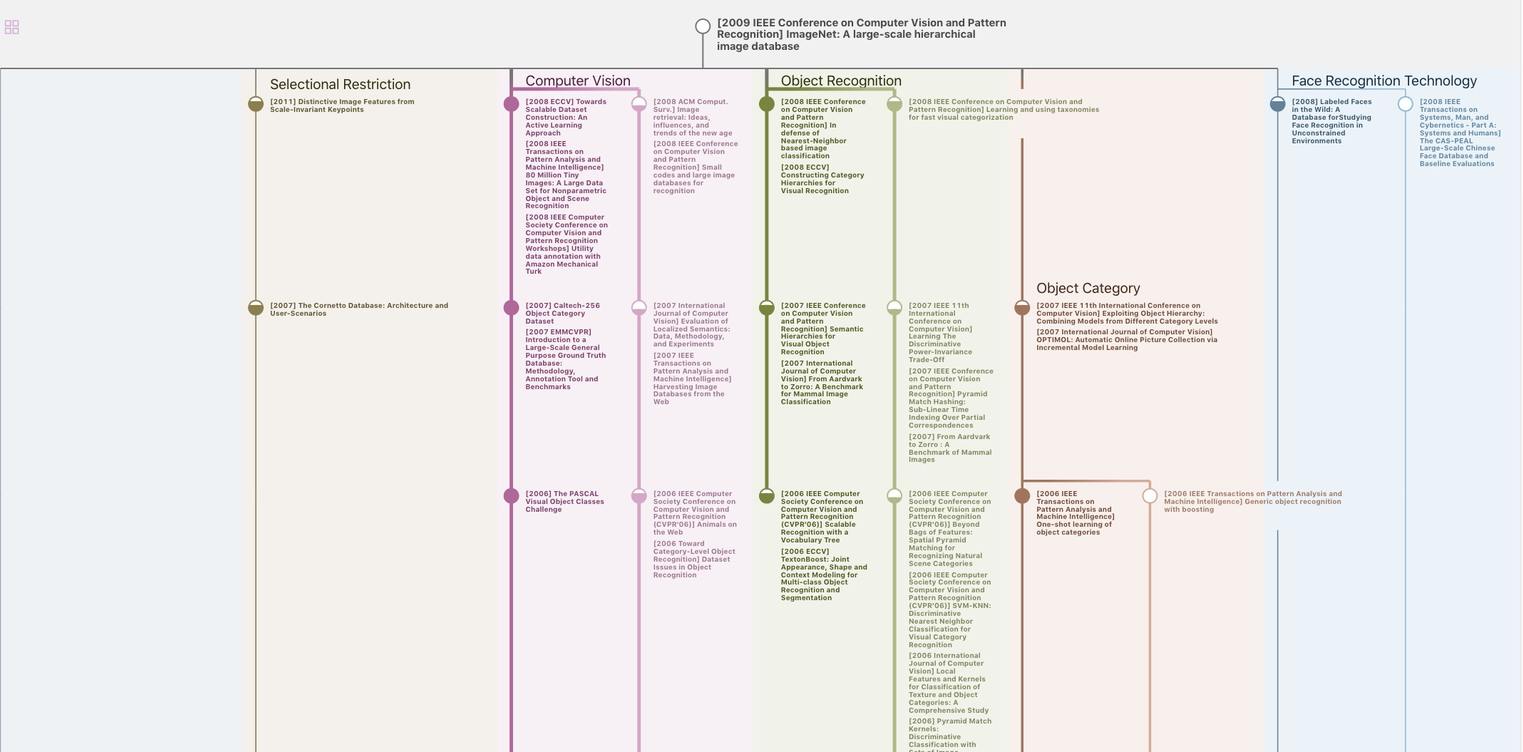
生成溯源树,研究论文发展脉络
Chat Paper
正在生成论文摘要