An Improved Method for Rapid Un-Collapsed Building Extraction from Post-Disaster High-Resolution Remote Sensing Imagery Based on Multi-Scale Feature Alignment
INTERNATIONAL JOURNAL OF DIGITAL EARTH(2024)
Abstract
ABSTRACTQuick and accurate extraction of un-collapsed buildings from post-disaster High-resolution Remote Sensing Images (HRSIs) is imperative for emergency response. Pre-disaster HRSIs could serve as auxiliary data for training models to expedite this extraction process. However, the effectiveness of models trained directly on pre-disaster HRSIs tends to diminish when applied to post-disaster scenarios, mainly due to the notable discrepancies between these datasets. The current popular approach to mitigate this issue involves aligning features from pre- and post-disaster images using an unsupervised domain adversarial learning framework. However, conventional methods often fall short in reducing the substantial disparity between pre- and post-disaster images, due to a lack of comprehensive alignment of category-level and multi-scale features. To overcome these limitations, we propose the Multi-scale Global and Category-attention Features Alignment Network (MGCAN). This novel approach further refines feature alignment strategies by concurrently aligning both multi-scale global and category-attention features, thus effectively narrowing the gap between pre- and post-disaster HRSIs. Extensive experiments have demonstrated that MGCAN significantly improves the accuracy of un-collapsed building extraction from post-disaster HRSIs. Moreover, compared to other state-of-the-art domain adversarial networks, MGCAN exhibits superior performance in different disaster scenarios.
MoreTranslated text
Key words
Post-disaster,un-collapsed building extraction,category-attention feature,multi-scale feature,unsupervised domain adversarial
AI Read Science
Must-Reading Tree
Example
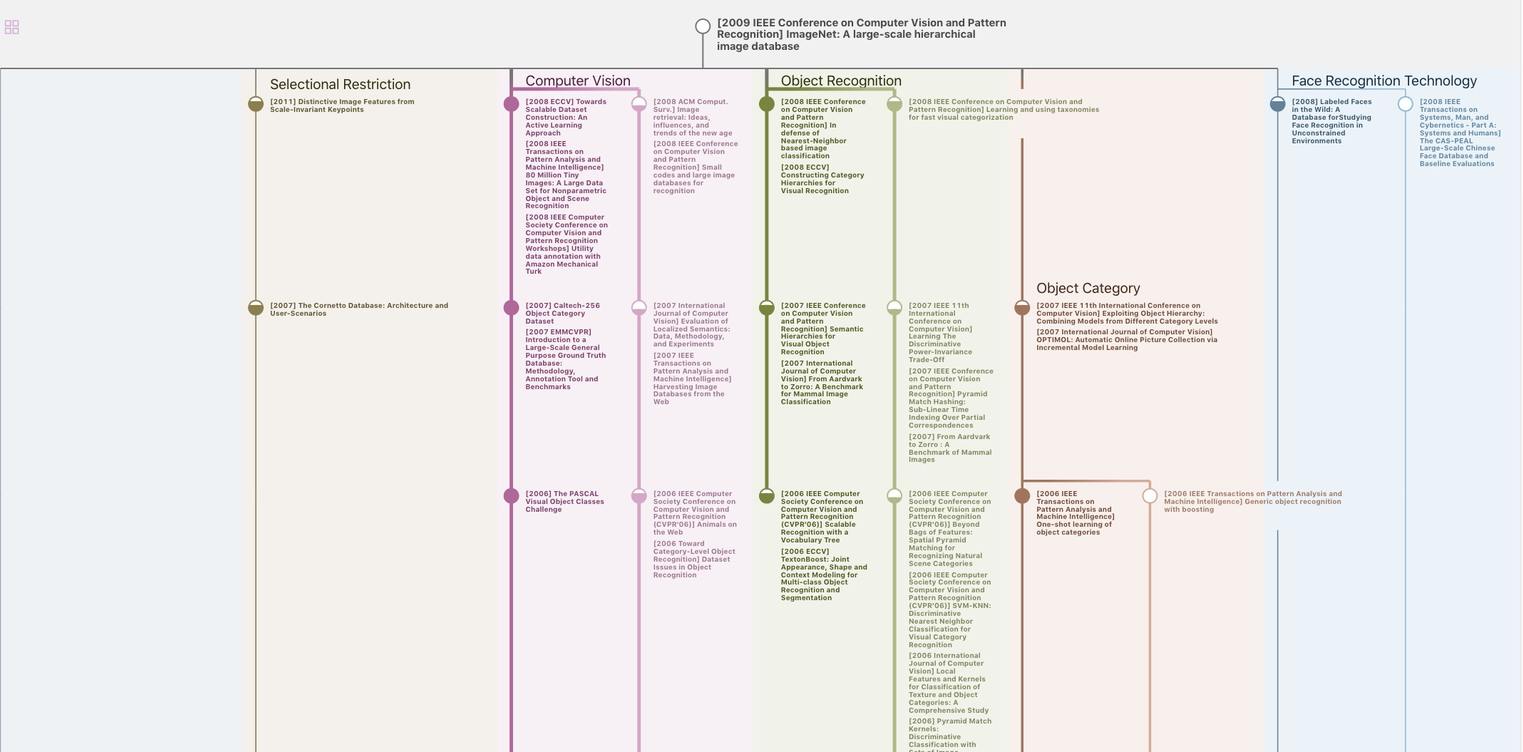
Generate MRT to find the research sequence of this paper
Chat Paper
Summary is being generated by the instructions you defined