Low-rank tensor learning with projection distance metric for multi-view clustering
International Journal of Machine Learning and Cybernetics(2024)
摘要
Multi-view subspace approaches have been extensively studied for their ability to project data onto a low-dimensional space, which is in favour of the clustering task. However, most existing models mainly concentrate on reconstructing data from the sample space, neglecting crucial information from the feature space, and failing to learn an optimal representation. For addressing this issue, we present a new joint framework, dubbed low-rank tensor learning with projection distance metric. This model recovers the original data by learning two low-rank factors, which thoroughly exploits the essential data information. Specifically, a low-rank constraint is introduced on a tensor that integrates subspace representations of all view data, enabling it to capture high-order relationships among views while recovering data from the sample space. Meanwhile, a low-rank projection matrix calculated by decomposing the original features is utilized to enhance data structures via exploring relationships among feature dimensions. Additionally, a distance metric learned by the projection matrix is introduced to leverage the local structure embedded in samples, thereby encouraging the learned representation to be more discriminative. Extensive experimental results on six datasets indicate the superiority of the proposed model.
更多查看译文
关键词
Multi-view learning,Low-rank tensor,Projection distance,Representation learning,Subspace clustering
AI 理解论文
溯源树
样例
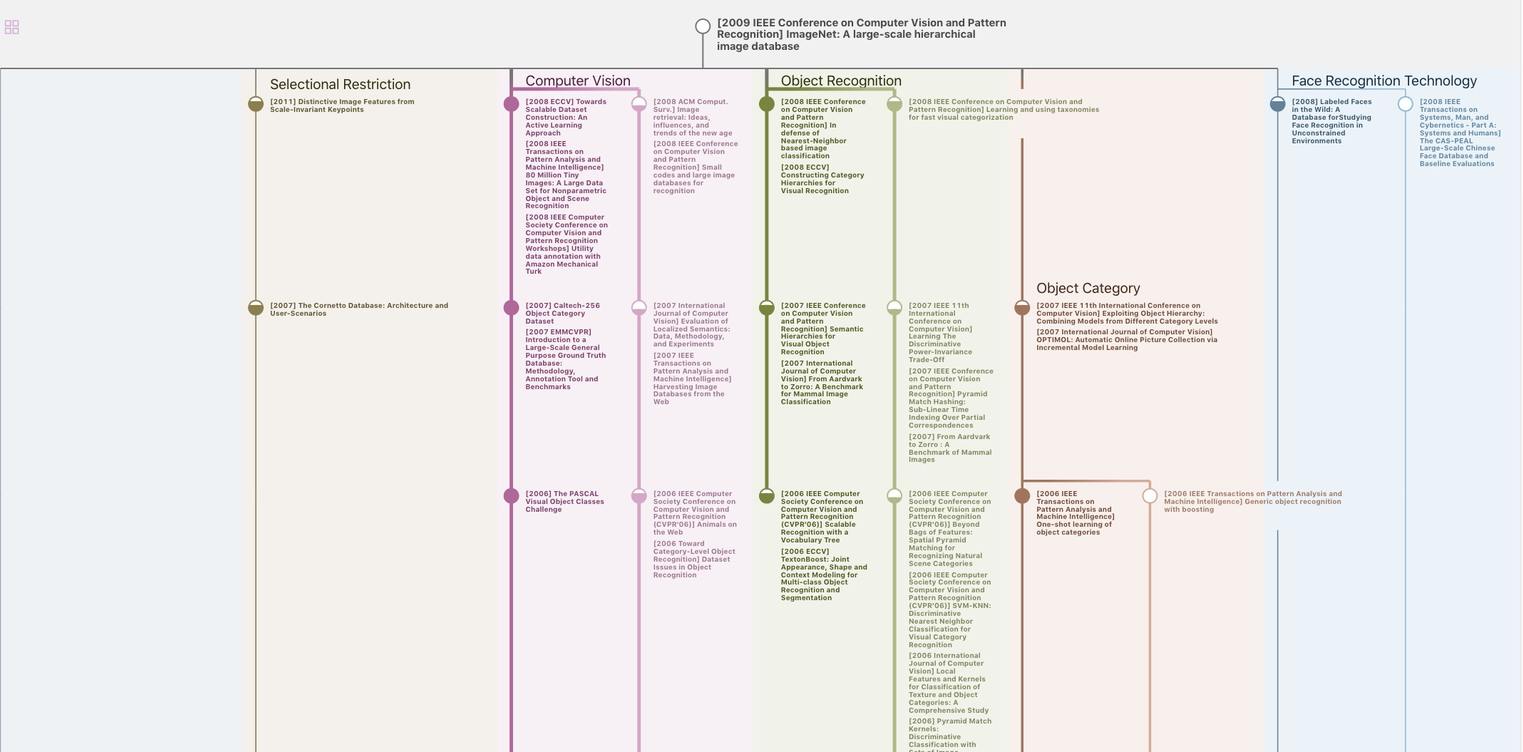
生成溯源树,研究论文发展脉络
Chat Paper
正在生成论文摘要