Precipitation Modeling Based on Spatio-Temporal Variation in Lake Urmia Basin Using Machine Learning Methods
Water(2024)
摘要
The amount of rainfall in different regions is influenced by various factors, including time, place, climate, and geography. In the Lake Urmia basin, Mediterranean air masses significantly impact precipitation. This study aimed to model precipitation in the Lake Urmia basin using monthly rainfall data from 16 meteorological stations and five machine learning methods (RF, M5, SVR, GPR, and KNN). Eight input scenarios were considered, including the monthly index, longitude, latitude, altitude, distance from stations to Lake Urmia, and distance from the Mediterranean Sea. The results revealed that the random forest model consistently outperformed the other models, with a correlation rate of 0.968 and the lowest errors (RMSE = 5.66 mm and MAE = 4.03 mm). This indicates its high accuracy in modeling precipitation in this basin. This study’s significant contribution is its ability to accurately model monthly precipitation using spatial variables and monthly indexes without measuring precipitation. Based on the findings, the random forest model can model monthly rainfall and create rainfall maps by interpolating the GIS environment for areas without rainfall measurements.
更多查看译文
关键词
precipitation modeling,machine learning,spatio-temporal change,M5 tree model,random forest model,Lake Urmia basin
AI 理解论文
溯源树
样例
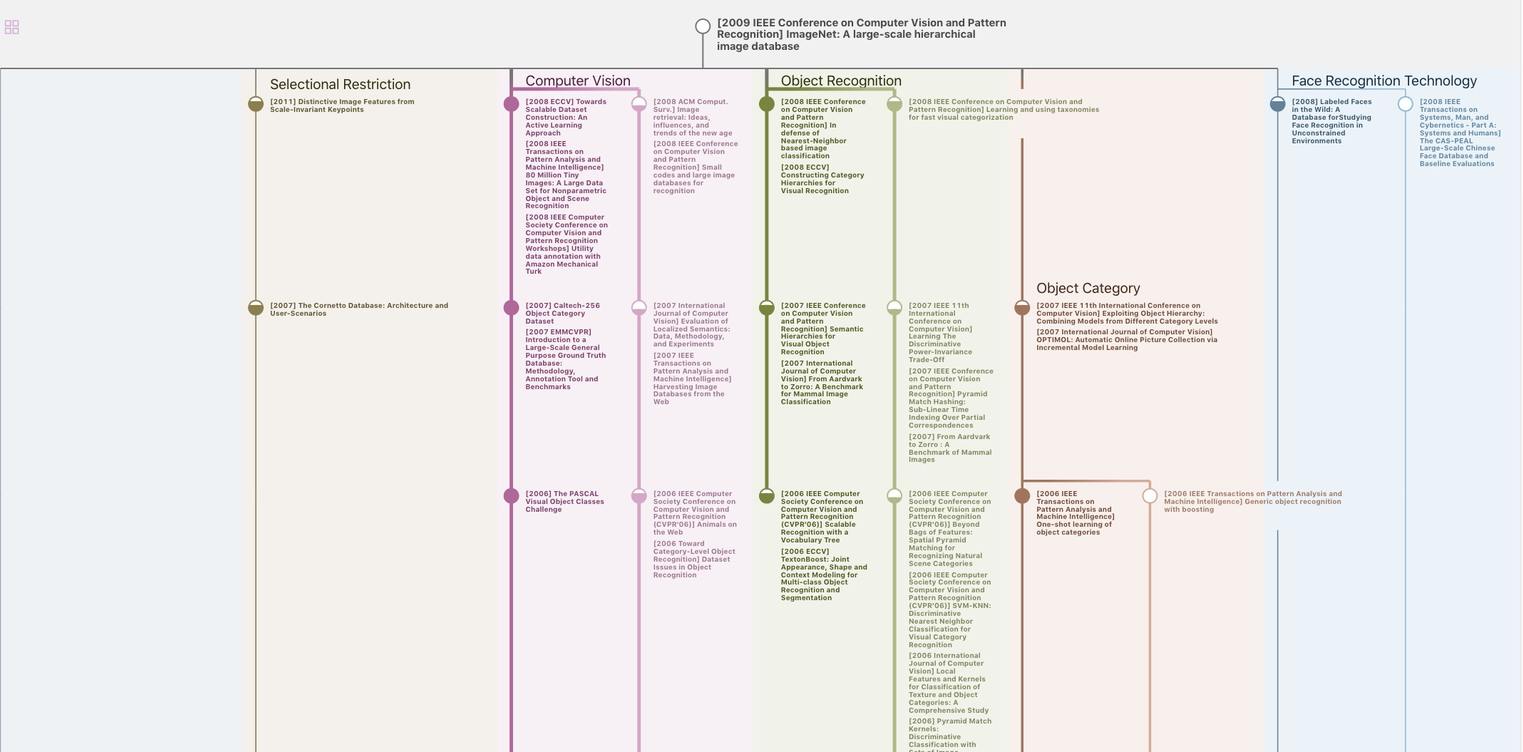
生成溯源树,研究论文发展脉络
Chat Paper
正在生成论文摘要