A Predictive Machine Learning-Based Analysis for In-Tube Co-current Steam Condensation Heat Transfer in the Presence of Non-condensable Gases
Arabian Journal for Science and Engineering(2024)
摘要
The primary objective of this study is to develop a predictive model that accurately estimates the condensation heat transfer coefficient (HTC) in the context of in-tube co-current flow condensation, with specific consideration given to the influence of non-condensable gases (NCGs). The need to find useful models and/or correlations that can accurately predict how well a passive condenser in the Economic Simplified Boiling Water Reactor will transfer heat during condensation is greatly warranted. This innovative cooling system utilizes gravity to condense water vapor, effectively dissipating heat from the containment vessel in the event of an accident and transferring the heat into the secondary cooling system. For the development of the forecasting model, we employed Multi-Gens Genetic Programming implemented in MATLAB. This approach possesses the capability of enabling visualization and articulating a transparent mathematical expression that directly establishes the connection between inputs and outputs. This model was specifically developed for forecasting the HTC during the condensation process, considering two distinct types of NCG: air, helium, and a mixture of air and helium, in addition to data for pure steam. The model was trained using a dataset comprising 2913 data points gathered from five experimental references. The model's input comprises a varied range of parameters, including local location ( z ), temperature of the steam/NCG mixture ( T_mix ), condenser wall temperature ( T_wall ), coolant flow rate ( β_c ), total pressure ( P_tot ), condenser inner diameter ( D_1 ), condenser outer diameter ( D_2 ), molecular weight (MM), NCG mass fraction ( w_NCG ), and mixture Reynolds number ( Re_mix ). The output of our model corresponds to the condensation HTC ( h ). Four specific correlations were examined with the third correlation attaining the highest anticipated values within a deviation range of ± 30, reaching 90.9
更多查看译文
关键词
Condensation HTC,ESBWR,In-tube co-current flow condensation,Machine learning,Multi-gens genetic programming,Non-condensable gases
AI 理解论文
溯源树
样例
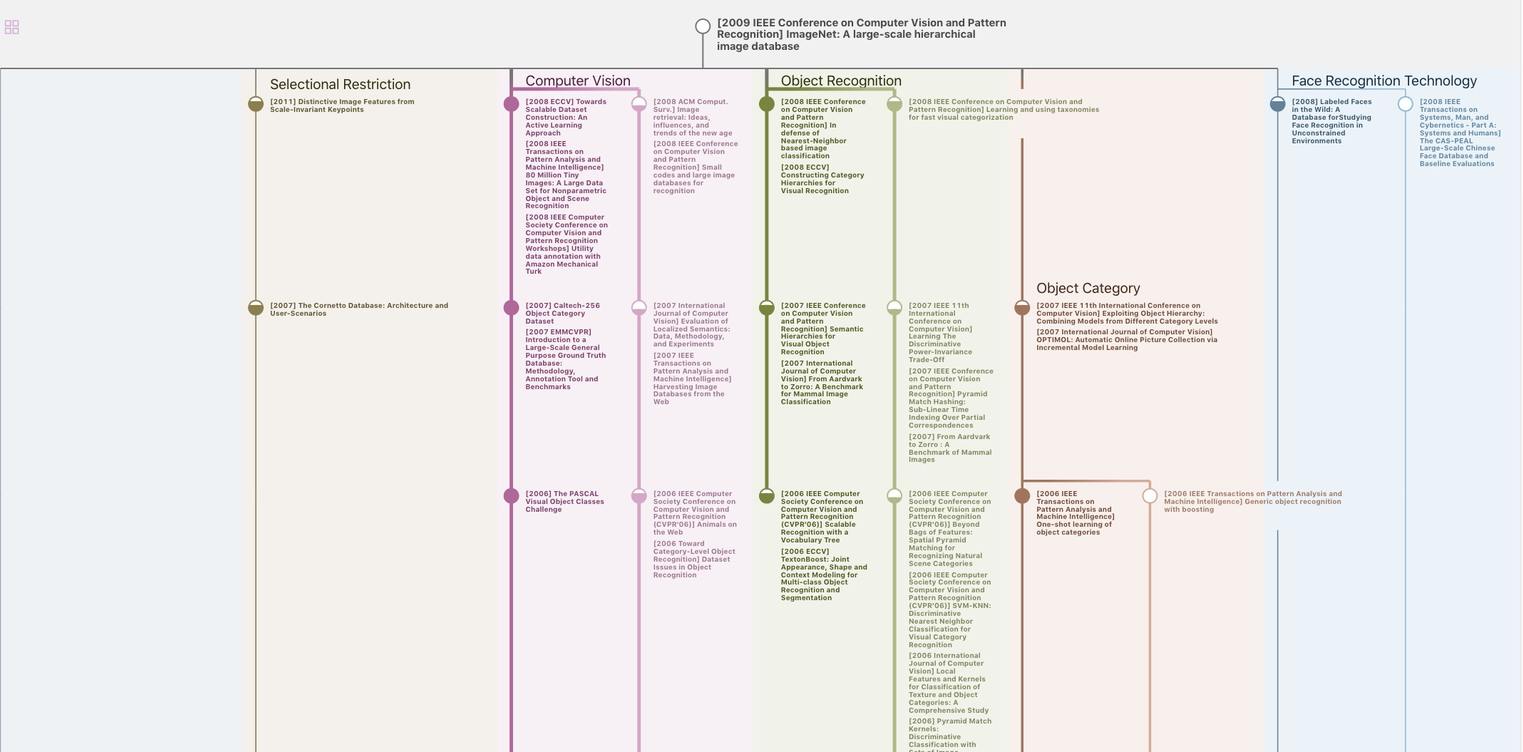
生成溯源树,研究论文发展脉络
Chat Paper
正在生成论文摘要