The Generative Adversarial Neural Network with Multi-Layers Stack Ensemble Hybrid Model for Landslide Prediction in Case of Training Sample Imbalance
Stochastic environmental research and risk assessment(2024)
摘要
Gilgit-Baltistan, Pakistan, is particularly susceptible to landslides due to various geological, tectonics, meteorological, and anthropogenic factors consequently. However, the persisting conundrum of landslide database/data imbalance stands as a formidable challenge within this domain. To better stabilize the objective of landslide prediction, stacking ensemble Machine Learning and Generative Adversarial Network (GAN) were applied, because previous research in this area has mostly been limited by a lack of data. GAN is employed to synthesize training samples, ensuring the creation of a balanced dataset. Stacking ensemble architecture involves two stages of learning: the first class of learners incorporates diverse machine learning algorithms, while, the second level logistic regression model integrates prediction based on the strong learner, thereby enhancing overall prediction performance. To investigate landslide susceptibility in District Chilas, Northern Pakistan, we employed optical remote sensing and introduced a GAN with a Multi-Layers Hybrid Model (MLHM). This study involved the preparation of a spatial database with a total of 106 landslides and ten major landslide factors. We utilized a hybrid ensemble model and compared its performance with different algorithms like Conventional Neural Network, Artificial Neural network, Decision Tree, K-Nearest Neighbouring, and Hybrid Model, achieving accuracies of 0.91, 0.92, 0.90, 0.89, and 0.93, respectively. this approach has with Hybrid architecture learning accuracy of 0.98. The GAN with MLHM developed improved landslide susceptibility assessment with cross-comparison of Persistent Scattered Interferometric Synthetic Aperture Radar (PS-InSAR) investigation to ensure the safe functioning of KKH.
更多查看译文
关键词
Landslide Prediction,Feature Selection Technique,Data Balancing,Generative Adversarial Network,Multi-Layers Hybrid Model,PS-InSAR
AI 理解论文
溯源树
样例
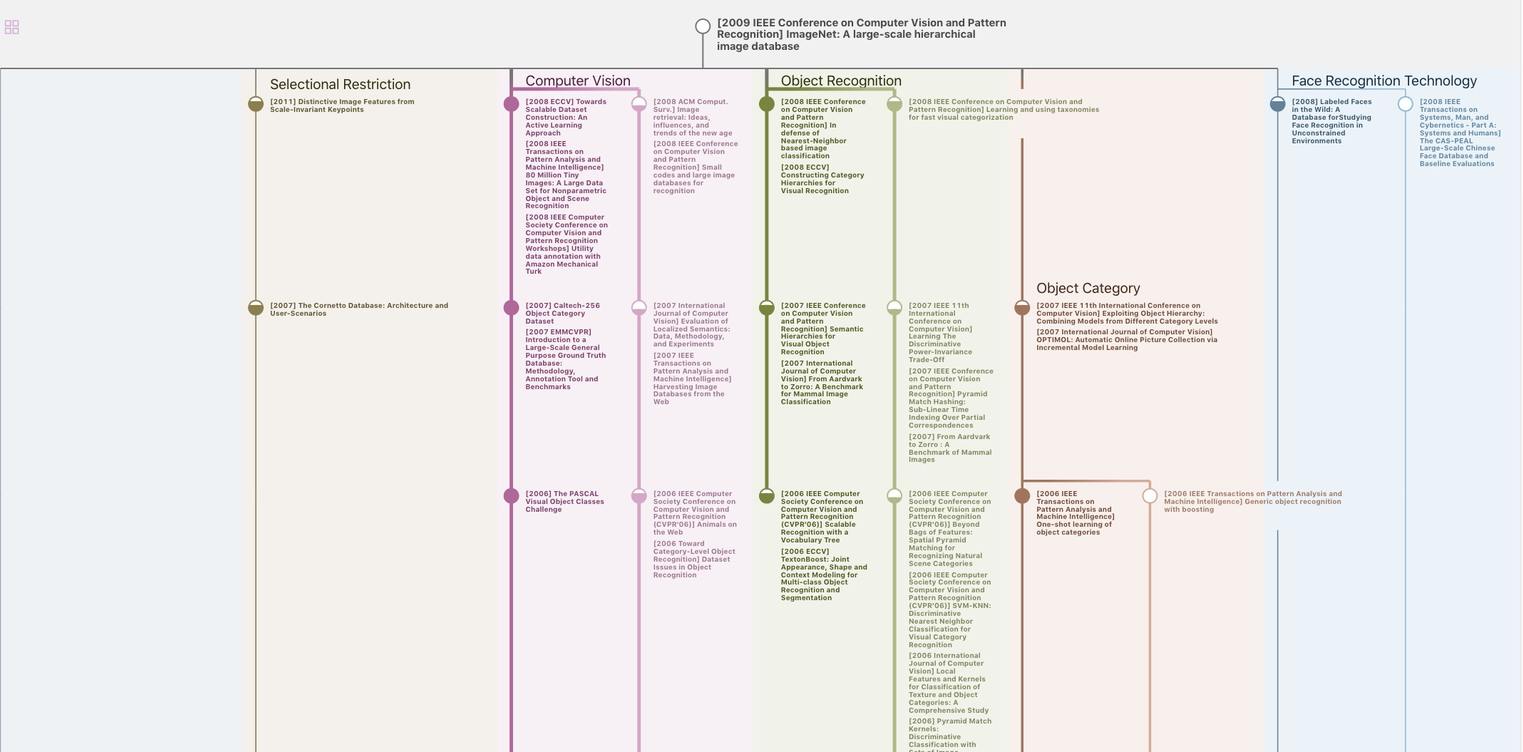
生成溯源树,研究论文发展脉络
Chat Paper
正在生成论文摘要