Modeling of water gas shift reaction using neural network trained on detailed kinetic mechanisms
Chemical Engineering Journal(2024)
摘要
For the optimization of chemical reactor or its control, computational fluid dynamics (CFD) that considers accurate reaction models is effective. Although detailed kinetic mechanisms can accurately predict gas-phase reactions, integrating them with CFD is too computationally expensive. In contrast, global reactions have lower computational costs but significantly reduced accuracy outside of tuned conditions. This study proposes a method for constructing a model using a neural network to predict the reaction rate of water gas shift reaction based on detailed kinetic mechanisms. It includes techniques to avoid unrealistic solutions inherent to detailed kinetic mechanisms and methods to minimize biases in training data. The neural network input was log-transformed concentrations and inverse temperatures, and the output was the logarithm of the absolute value of the reaction rate and direction, enabling the prediction of bidirectional reactions over a wide temperature range. The quality of the dataset significantly impacted the accuracy of the neural network. Using a dataset with less bias in chemical species concentrations, the model achieved accuracy similar to detailed models while facilitating an over 400 times faster computation. The study also discussed the impact of temperature change rate, showing that at about 72 K/min, the detailed kinetic mechanism's solution can be accurately replicated, but at 720 K/min, the radical concentration deviates from the quasi-steady state, leading to discrepancies. This study opens up new possibilities for computational methods in the prediction and optimization of chemical reactions.
更多查看译文
关键词
Water gas shift reaction,Neural network,Kinetic analysis,Antibias for concentration,Detailed kinetic mechanism
AI 理解论文
溯源树
样例
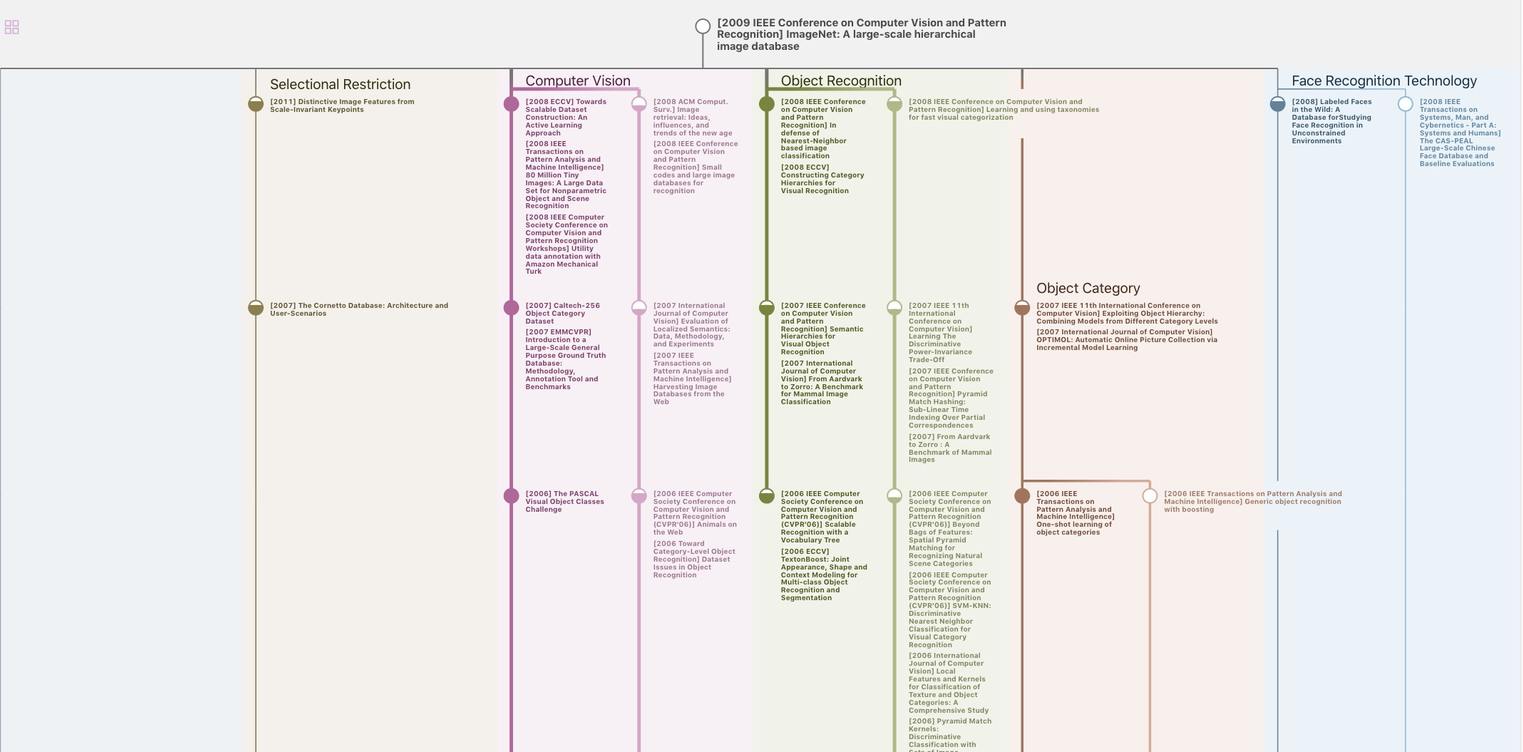
生成溯源树,研究论文发展脉络
Chat Paper
正在生成论文摘要