Off-Dynamics Inverse Reinforcement Learning.
IEEE Access(2024)
摘要
Imitation learning is a widely-used paradigm for decision making that learns from expert demonstrations. Existing imitation algorithms often require multiple interactions between the agent and the environment from which the demonstration is obtained. The acquisition of expert demonstrations in simulator usually requires specialized knowledge. In addition, real-world interactions are limited due to security or cost concerns. Therefore, the direct application of existing imitation learning algorithms in either real world or simulator is not an ideal strategy. In this paper, we propose a cross-domain Inverse Reinforcement Learning training paradigm that learns a reward function from hetero-domain expert’s demonstration, while the interaction with the environment that obtains demonstrations should be limited. In order to solve the distribution shift under such paradigm, we propose a transfer learning method called off-dynamics Inverse Reinforcement Learning. The intuition behind off-dynamics Inverse Reinforcement Learning is that the goal of reward function learning is not only to imitate experts, but also to promote action adaptation to the dynamic difference between two hetero-domain. Specifically, a widely-used Inverse Reinforcement Learning framework was adopted, and its discriminator for identifying agent-generated trajectories was modified with quantified dynamic differences. The training process of the discriminator yields the transferable reward function suitable for the target dynamics, which is guaranteed by our theoretical derivation. Off-dynamics Inverse Reinforcement Learning assigns higher rewards to demonstration trajectories that do not exploit discrepancies between the two domains. Our method demonstrates its effectiveness and scalability to high-dimensional tasks through extensive experiments on continuous control tasks. Our code is available on the project website: https://github.com/yachenkang/ODIRL.
更多查看译文
关键词
Hetero-Domain,Imitation Learning,Inverse Reinforcement Learning,Off-Dynamics,Transfer Learning
AI 理解论文
溯源树
样例
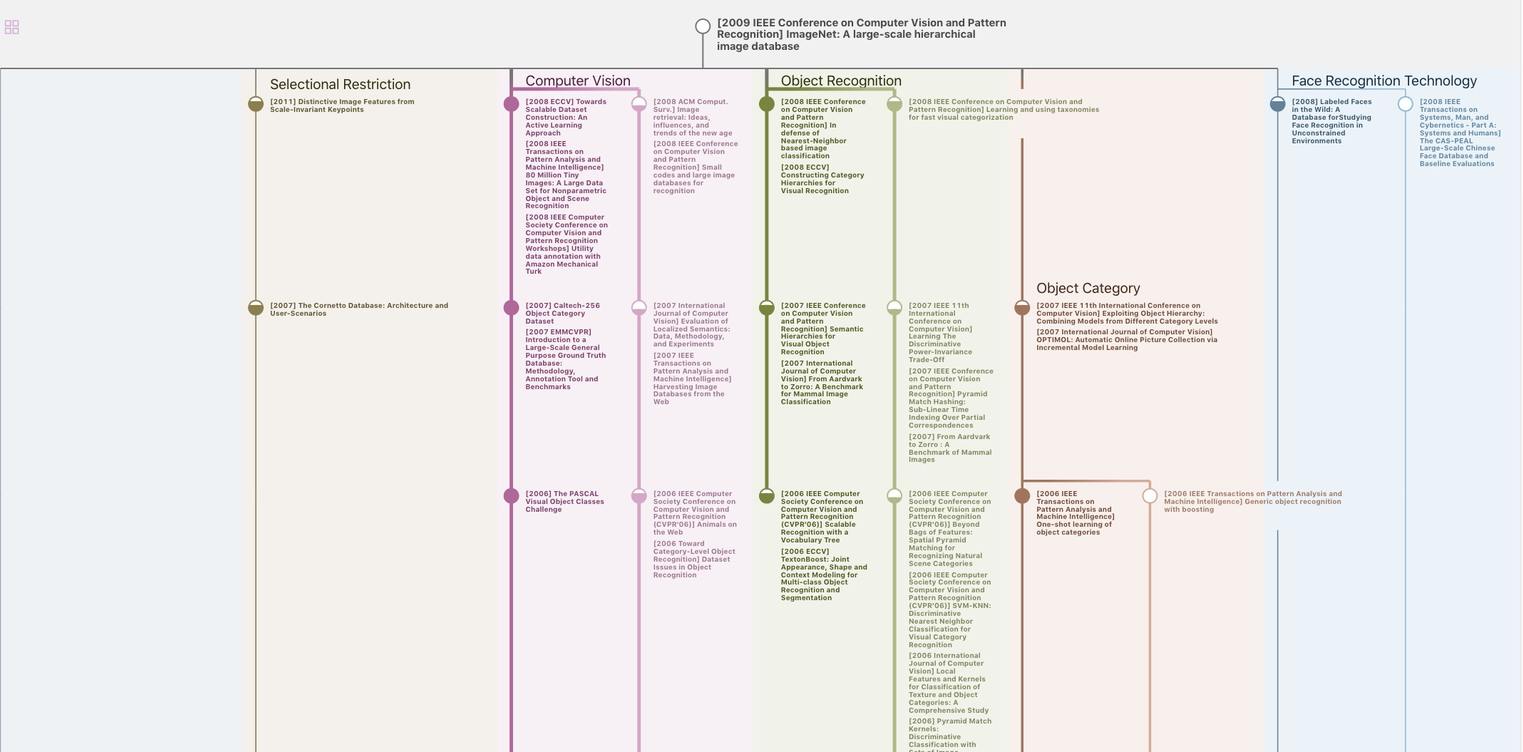
生成溯源树,研究论文发展脉络
Chat Paper
正在生成论文摘要