LCDSE: Enable Efficient Design Space Exploration for DCNN Accelerator Based on Layer Clustering
IEEE Transactions on Circuits and Systems II: Express Briefs(2024)
摘要
Efficient design space exploration method is crucial for optimizing deep Convolutional Neural Network accelerators, as the large design space comprising hardware architecture and dataflow mapping parameters leads to prohibitive optimization time costs. Prior works employ space compression to improve exploration efficiency but focus only on single layers or partial spaces, failing to prevent expansion as network model depth increases. Therefore, in this work, we propose the LCDSE framework to address the dilemma of space expansion. LCDSE clusters similar layers into blocks as coarse-grained optimization units to constrain parameters and prune redundant design space. Compared to former frameworks, LCDSE achieves up to 4x delay speedup, 7.9x energy-delay-product decrease and 2.5x area-delay-product reduction along with time cost saving by up to 11.3x, demonstrating its superior optimization efficiency.
更多查看译文
关键词
Neural Network,Accelerator,Design Space Exploration,Reinforcement Learning,Design Automation
AI 理解论文
溯源树
样例
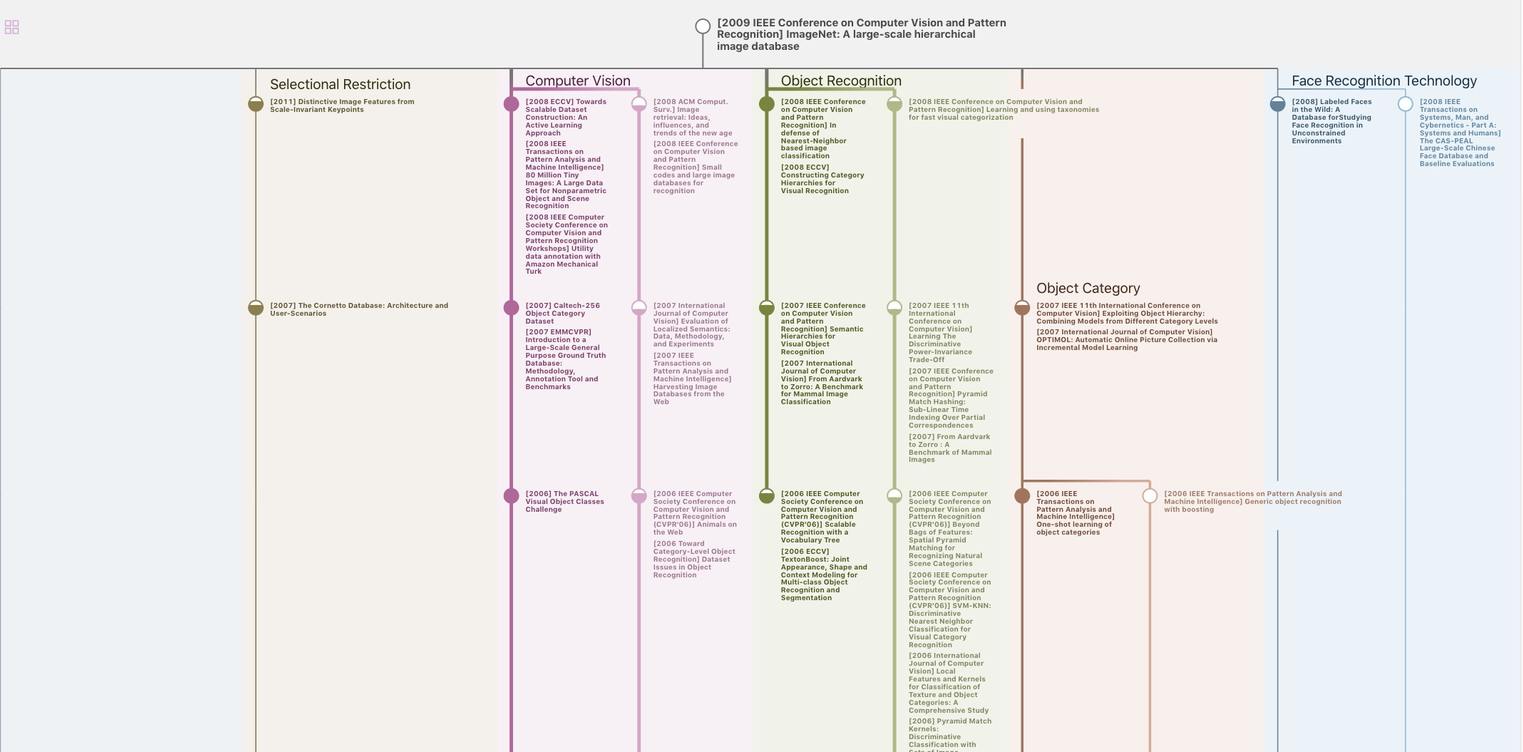
生成溯源树,研究论文发展脉络
Chat Paper
正在生成论文摘要