Exploring the Impact of Fine-Tuning the Wav2vec2 Model in Database-Independent Detection of Dysarthric Speech.
IEEE journal of biomedical and health informatics(2024)
摘要
Many acoustic features and machine learning models have been studied to build automatic detection systems to distinguish dysarthric speech from healthy speech. These systems can help to improve the reliability of diagnosis. However, speech recorded for diagnosis in real-life clinical conditions can differ from the training data of the detection system in terms of, for example, recording conditions, speaker identity, and language. These mismatches may lead to a reduction in detection performance in practical applications. In this study, we investigate the use of the wav2vec2 model as a feature extractor together with a support vector machine (SVM) classifier to build automatic detection systems for dysarthric speech. The performance of the wav2vec2 features is evaluated in two cross-database scenarios, language-dependent and language-independent, to study their generalizability to unseen speakers, recording conditions, and languages before and after fine-tuning the wav2vec2 model. The results revealed that the fine-tuned wav2vec2 features showed better generalization in both scenarios and gave an absolute accuracy improvement of 1.46% - 8.65% compared to the non-fine-tuned wav2vec2 features.
更多查看译文
关键词
Dysarthria,fine-tuning,self-supervised learning,wav2vec 2.0
AI 理解论文
溯源树
样例
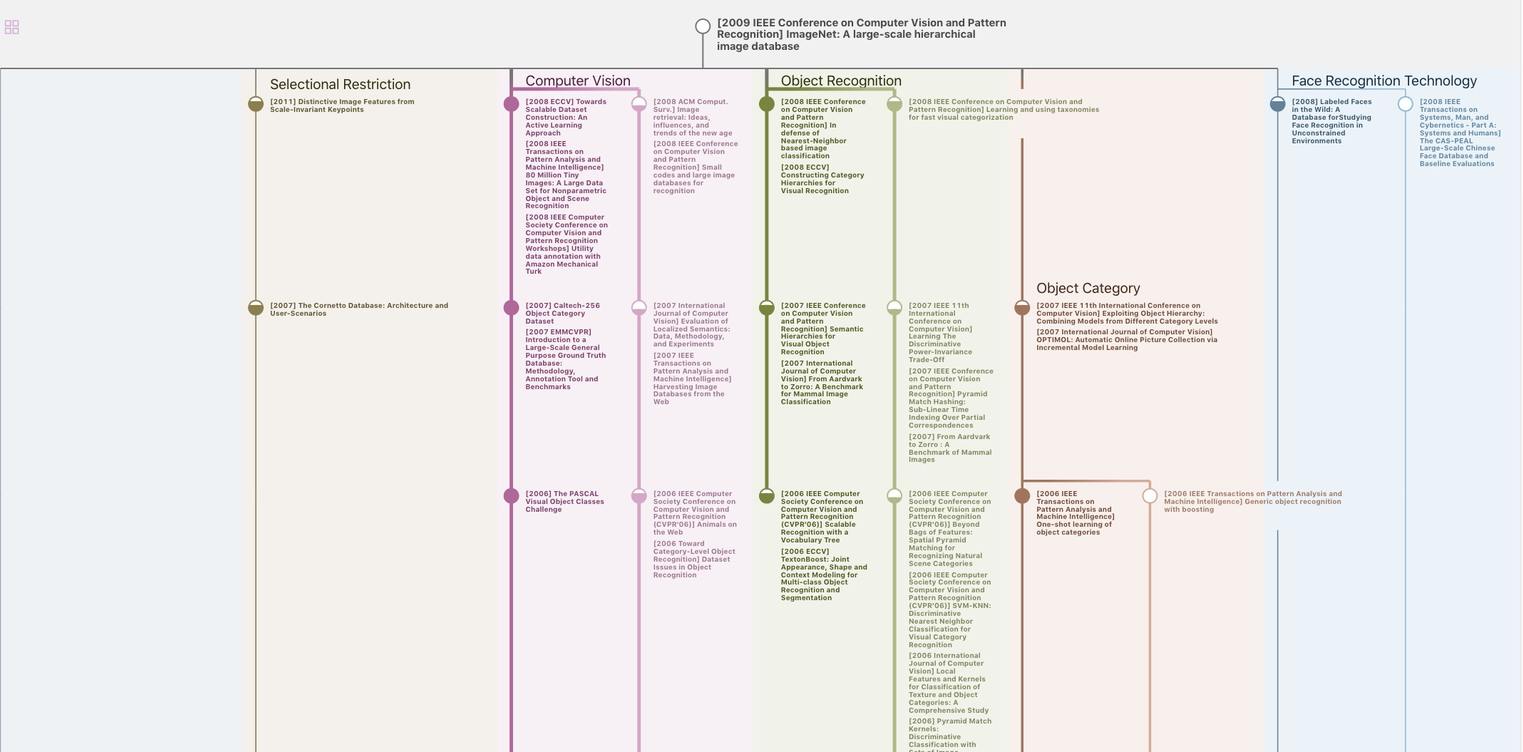
生成溯源树,研究论文发展脉络
Chat Paper
正在生成论文摘要