Game-Theoretic Design of Quality-Aware Incentive Mechanisms for Hierarchical Federated Learning
IEEE Internet of Things Journal(2024)
摘要
Hierarchical Federated Learning (HFL) improves the scalability and communication efficiency of the system and achieves load balancing at each level. Incentive mechanisms enhance participant motivation and optimize resource allocation for HFL. However, existing mechanisms mainly focus on maximizing individual utility from the quantity of client data while neglecting to optimize social utility from the learning quality perspective. Meanwhile, strategic behavior and heterogeneous devices can significantly degrade the performance of incentive mechanisms. To this end, we propose a quality-aware incentive mechanism (QAIM) for HFL to improve training efficiency. Specifically, we first systematically evaluate the learning quality of clients based on their training loss and historical records, which allows us to recruit high-quality clients for model updating selectively. Then, we model the cloud-edge-end interaction and cooperation as a three-layer Stackelberg game to analyze the strategies of participants and utilize carefully designed algorithms to derive the solution of the unique Stackelberg Equilibrium (SE). Through the Pareto improvement of client association modeled as a coalition game, we can maximize social utility. Experimental results on both synthetic and real-world datasets demonstrate that our QAIM outperforms the state-of-the-art baselines, with an average increase in accuracy and social utility of 17% and 45%, respectively.
更多查看译文
关键词
Incentive mechanism,Hierarchical federated learning,Quality-aware,Game theory,Client association
AI 理解论文
溯源树
样例
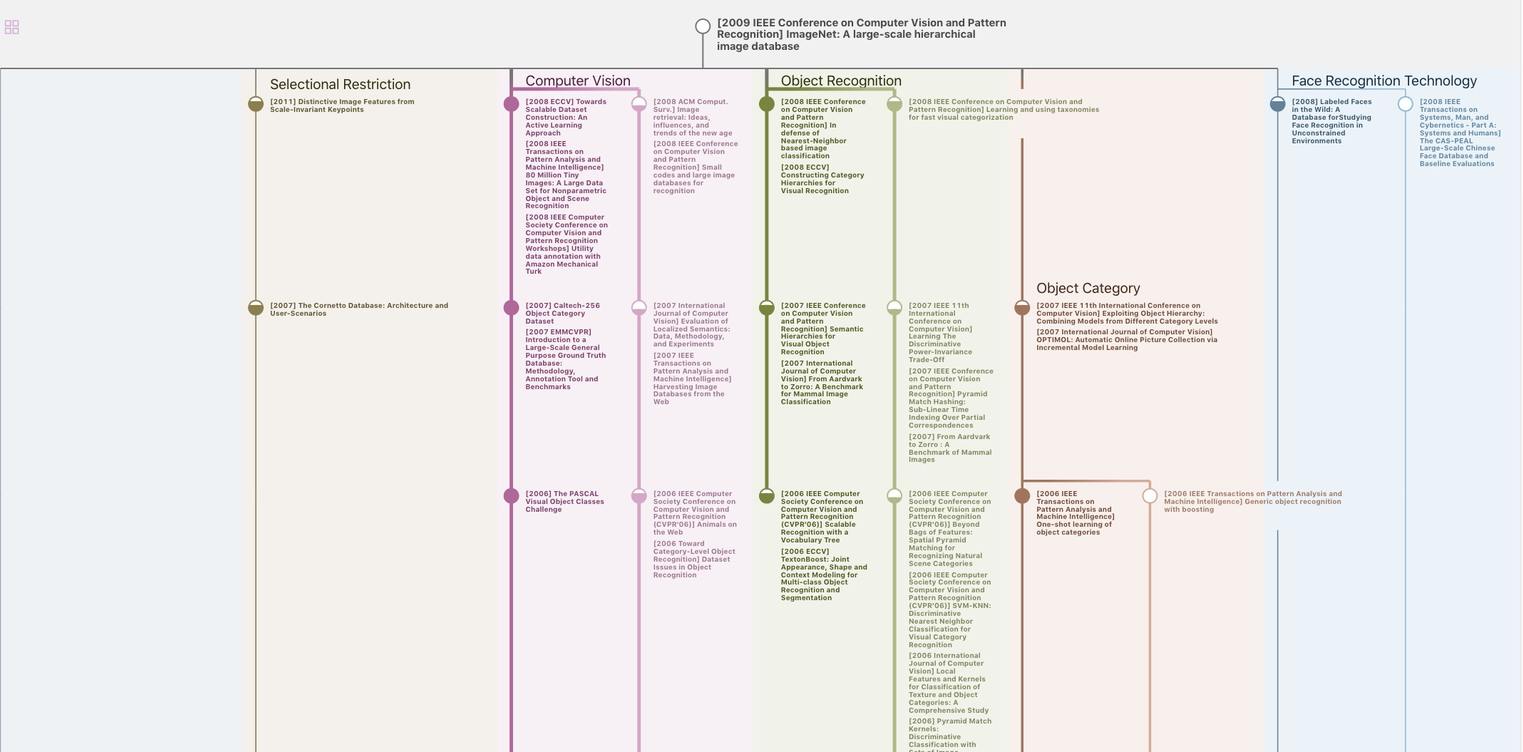
生成溯源树,研究论文发展脉络
Chat Paper
正在生成论文摘要