A fast hypergraph neural network with detail preservation for hyperspectral image classification
INTERNATIONAL JOURNAL OF REMOTE SENSING(2024)
摘要
Hypergraph neural networks (HGNNs), extending the techniques of graph neural networks, have been applied to various fields due to their ability to capture more complex high-order node relationships. However, for hyperspectral image (HSI) classification tasks, previous HGNN-based works usually constructed hypergraphs using pixels as nodes, resulting in massive computational costs. Meanwhile, pixel-level personalized features are required for HSI classification. To achieve high efficiency and accuracy simultaneously, this paper presents a fast hypergraph neural network with detail preservation (DPFHNet) for HSI classification. It constructs hypergraphs at the superpixel level to reduce time consumption and supplement pixel-level detail features through a classification refinement module. This framework contains multiple stages. Firstly, its main stage is designed with HGNNs from a superpixel viewpoint rather than pixels, providing a fast strategy to capture high-order complex relationships of multiple homogeneous irregular regions. After that, auxiliary stages based on convolutional neural networks are integrated into the main stage, which adopts a hierarchical design and attempts to acquire pixel-level spatial-spectral information before the hypergraph feature extraction of the main stage, assisting in learning more valuable features. Finally, a classification refinement module is constructed, which generates pixel-level detail features to refine the superpixel-level features obtained by HGNN. Experiments on three datasets illustrate that DPFHNet achieves competitive results and efficiency compared to advanced methodologies.
更多查看译文
关键词
Hyperspectral image classification,hypergraph learning,graph neural network,convolutional neural network
AI 理解论文
溯源树
样例
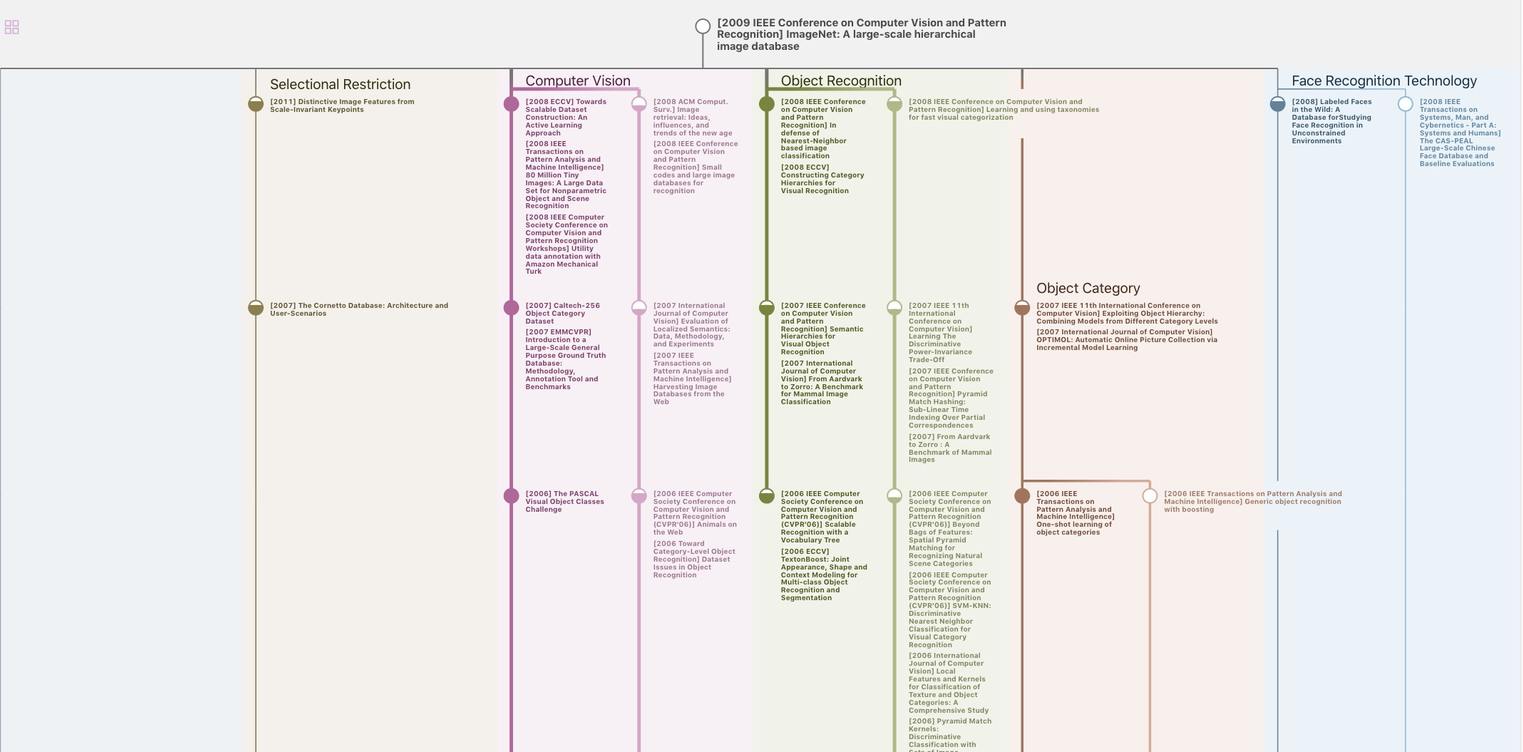
生成溯源树,研究论文发展脉络
Chat Paper
正在生成论文摘要