Lightweight object detection network for multi‐damage recognition of concrete bridges in complex environments
Computer-Aided Civil and Infrastructure Engineering(2024)
摘要
AbstractTo solve the challenges of low recognition accuracy, slow speed, and weak generalization ability inherent in traditional methods for multi‐damage recognition of concrete bridges, this paper proposed an efficient lightweight damage recognition model, constructed by improving the you only look once v4 (YOLOv4) with MobileNetv3 and fused inverted residual blocks, named YOLOMF. First, a novel lightweight network named MobileNetv3 with fused inverted residual (MobileNetv3‐FusedIR) is constructed as the backbone network for YOLOMF. This is achieved by integrating the fused mobile inverted bottleneck convolution (Fused‐MBConv) into the shallow layers of MobileNetv3. Second, the standard convolution in YOLOv4 is replaced with the depthwise separable convolution, resulting in a reduction in the number of parameters and complexity of the model. Third, the effects of different activation functions on the damage recognition performance of YOLOMF are thoroughly investigated. Finally, to verify the effectiveness of the proposed method in complex environments, a data enhancement library named Imgaug is used to simulate concrete bridge damage images under challenging conditions such as motion blur, fog, rain, snow, noise, and color variations. The results indicate that the YOLOMF shows excellent multi‐damage recognition proficiency for concrete bridges across varying field‐of‐view sizes as well as complex environmental conditions. The detection speed of YOLOMF reaches 85f/s, facilitating effective real‐time multi‐damage detection for concrete bridges under complex environments.
更多查看译文
AI 理解论文
溯源树
样例
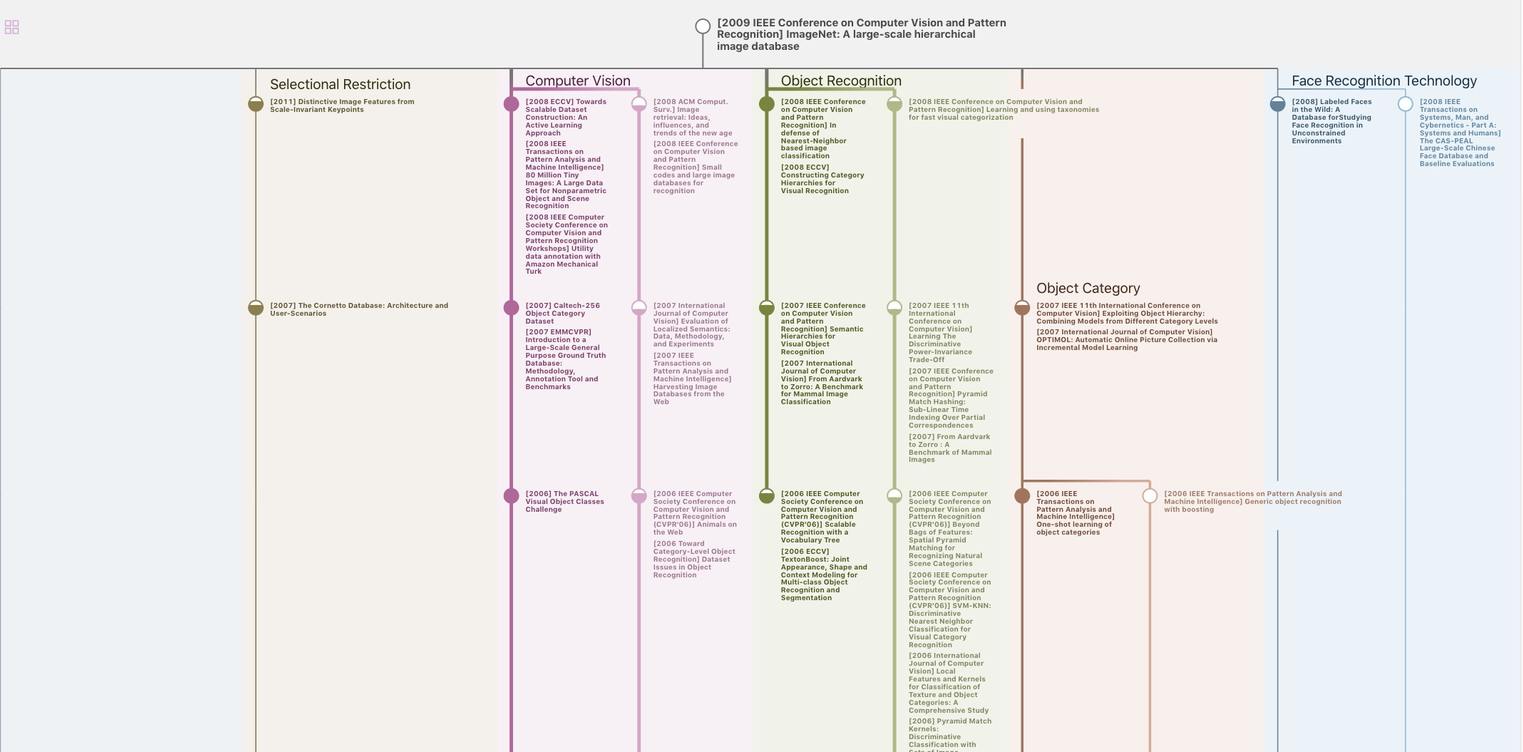
生成溯源树,研究论文发展脉络
Chat Paper
正在生成论文摘要