Domain-Agnostic Priors for Semantic Segmentation Under Unsupervised Domain Adaptation and Domain Generalization
International Journal of Computer Vision(2024)
摘要
In computer vision, an important challenge to deep neural networks comes from adjusting the varying properties of different image domains. To study this problem, researchers have been investigating a practical setting in which the deep neural networks are trained on a labeled source domain and then transferred to an unlabeled or even unseen target domain. The major difficulty lies in the potential domain gap, which essentially arises from the overfitting in the source domain. Hence, it is important to introduce generalized priors to alleviate the issue. From this perspective, this paper presents a novel framework that forces visual features to align with domain-agnostic priors (DAP). Specifically, we study two kinds of priors, (i) language-guided embedding and (ii) class-level relationship, and we believe that more such priors can be constructed. Our framework, referred to as DAP, is evaluated on both unsupervised domain adaptation (UDA) and domain generalization (DG) where the target domain is unlabeled and even unseen, respectively. We use the standard benchmark that performs transfer semantic segmentation on synthesized datasets (i.e., GTAv and SYNTHIA) and a real dataset (i.e., Cityscapes). Experiments validate the effectiveness of DAP with competitive accuracy in all tasks. In particular, language-guided priors work sufficiently well for UDA, while class-level priors serve as useful complements for DG. The proposed frameworks shed light that domain transfer benefits from better proxies, possibly from other modalities.
更多查看译文
关键词
Unsupervised domain adaptation,Domain generalization,Semantic segmentation,Domain-agnostic priors
AI 理解论文
溯源树
样例
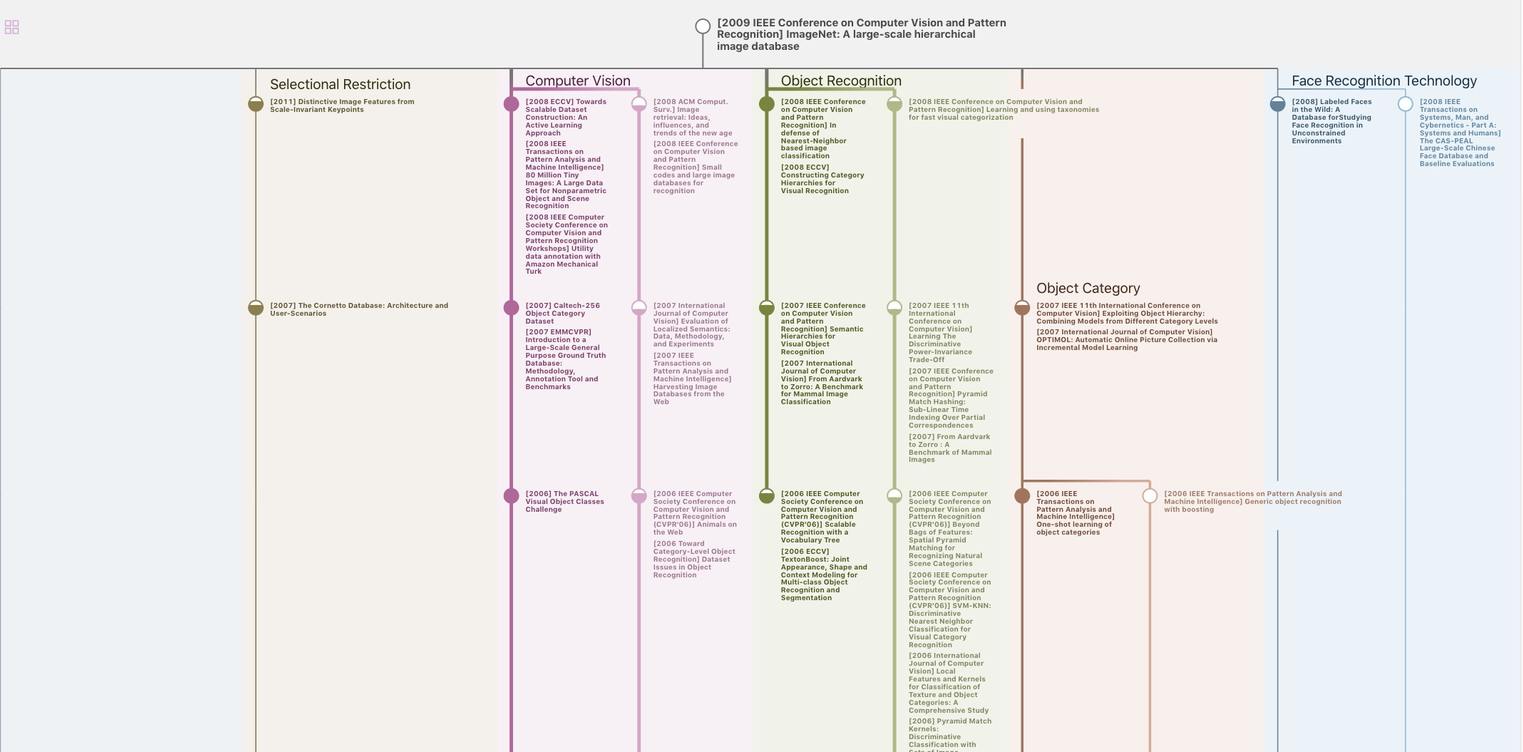
生成溯源树,研究论文发展脉络
Chat Paper
正在生成论文摘要