Classification of hand movements based on EMD-CCT feature extraction method through EMG using machine learning
Multimedia Tools and Applications(2024)
摘要
Internal and external noise frequently contaminates surface electromyography (sEMG) signals. These noises may impair the classifier’s ability to recognize upper limb movements accurately. Classification of hand movements is followed by several processes such as pre-processing, feature extraction and classification. Various Machine Learning (ML) and Deep Learning (DL) methods are employed in the early stages to classify hand movements. However, previous studies have various limitations regarding computation complexity, less accuracy and low precision. To overcome these limitations, a novel algorithm is proposed for processing hand movement EMG signals in this study, which consists of empirical mode decomposition (EMD) based on the denoising of sEMG signals inspired by correlation coefficient threshold (CCT). Furthermore, ML classifiers have been applied to recognize the various hand movement stages, such as extension, supination, radial deviation, flexion, pronation, and ulnar deviation. This study processed EMG signals of six hand movements performed by five subjects from Database 2 (intact) of the Ninapro benchmark database. In the first stage, EMD is applied to divide the noisy EMG signal into intrinsic mode functions (IMFs). CCT is used to determine relevant IMFs depending on the similarity between the decomposed IMFs and the input signal, and then the denoised signal is reconstructed from these selected IMFs. The performance of denoising is evaluated by the root mean square error (RMSE) and signal-to-noise ratio (SNR). The second stage involved extracting time-domain (TD) and histogram features from relevant IMFs and feeding them to four ML algorithms such as linear discriminant analysis (LDA), K-nearest neighbour (KNN), support vector machine (SVM), and Decision Tree (DT). In conclusion, LDA outperformed the other classifiers with an average accuracy of 94.63
更多查看译文
关键词
Electromyogram,EMD,Hand movements,Histogram,Machine learning classifiers,Time-domain features correlation coefficient
AI 理解论文
溯源树
样例
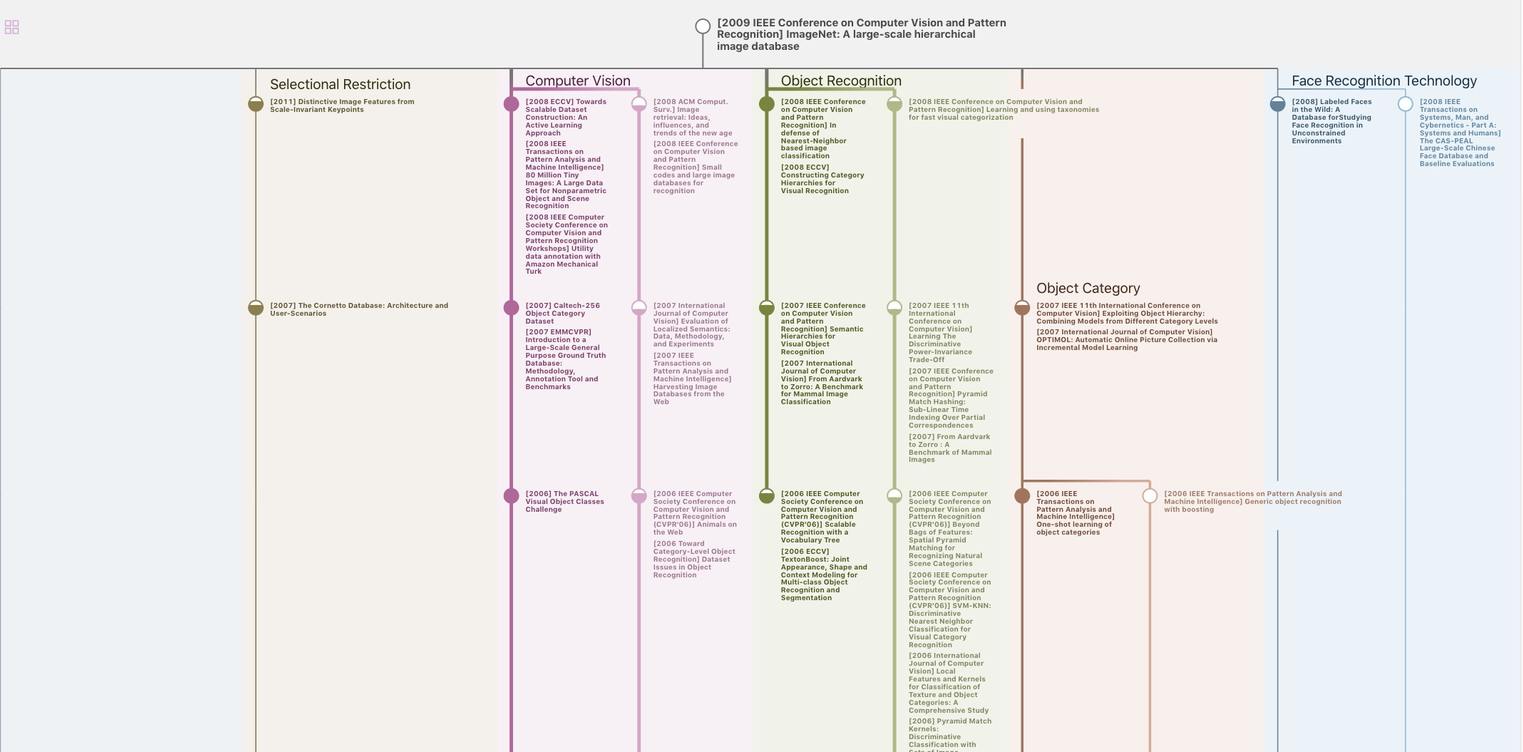
生成溯源树,研究论文发展脉络
Chat Paper
正在生成论文摘要