Using transformer and a reweighting technique to develop a remaining useful life estimation method for turbofan engines
Engineering Applications of Artificial Intelligence(2024)
摘要
Estimating the Remaining Useful Life (RUL) of industrial machinery is an important task in Prognostics and Health Management (PHM). Accurate RUL prediction based on current health status and real-time sensor measurements can help establish efficient maintenance strategies in advance, thus improving the productivity and efficiency of machinery operations. However, considering the nature of industrial machinery, a data imbalance problem, which exists in current RUL estimation datasets like the Commercial Modular Aero-Propulsion System Simulation (C-MAPSS) dataset, hinders data-driven approaches. To address the detrimental data imbalance problem and improve estimation performance, this research proposes an Adaptive RUL-wise Reweighting (ARR) technique. ARR uses kernel smoothing to maintain the inherent continuity of RUL and a reweighting method to rebalance the effects of samples with different ground-truth RULs. In addition, this research proposes a novel RUL estimation method that uses a transformer architecture, which has not yet been widely applied for PHM tasks. Using a transformer-based estimation model with high expressive power capable of handling multivariate time-series data has proven effective in RUL estimation. Comprehensive experiments using the C-MAPSS dataset based on cross validation are conducted to find optimal hyperparameter configurations and to validate the proposed method’s effectiveness in RUL estimation. For subsets FD001 and FD003 of the C-MAPSS dataset, the proposed method provides state-of-the-art estimation performance with the lowest Root Mean Squared Error (RMSE) values of 11.36 and 11.28 and score values of 192.22 and 133.41, respectively. The proposed method also shows highly competitive performance on subsets FD002 and FD004 of the C-MAPSS dataset.
更多查看译文
关键词
Deep learning,Predictive maintenance,Prognostics and health management,Remaining useful life,Reweighting technique,Transformer
AI 理解论文
溯源树
样例
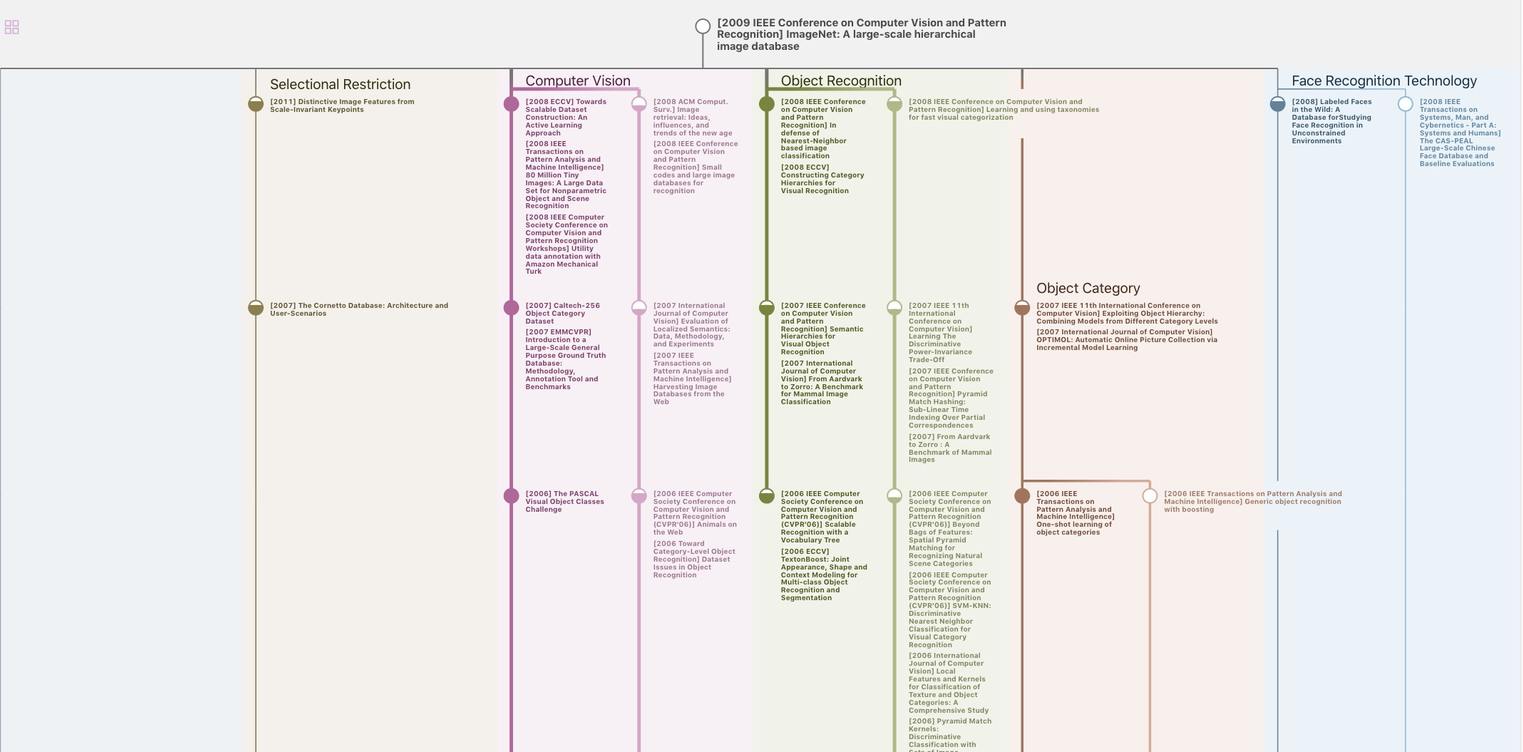
生成溯源树,研究论文发展脉络
Chat Paper
正在生成论文摘要