Dynamic multi-scale spatial-temporal graph convolutional network for traffic flow prediction
Future Generation Computer Systems(2024)
摘要
As a critical component of Intelligent Transportation Systems (ITS), traffic flow prediction is indispensable for vehicle routing and transportation management. However, traffic prediction is an intractable task due to the complex spatial–temporal dependencies. First, traffic data has multiple spatial dependencies that arise from different contexts and vary with time. Second, multi-scale temporal features naturally exist in the traffic data and have dynamic significance for predicting future traffic states. Insufficiently capturing such spatial and temporal dependencies will lead to the prediction performance degradation. To this end, we propose a dynamic multi-scale spatial–temporal graph convolutional network for traffic flow prediction. Unlike existing methods, we propose a novel attention layer assigning weights to each feature of nodes to augment the dynamic spatial dependence modeling and a dynamic multi-branch temporal network learning temporal features from the time domain and frequency domain complementary to enhance the dynamic multi-scale temporal features learning capability. Extensive experiments on four well-known traffic datasets demonstrate that the proposed model outperforms the best baselines with a ratio of 5.27%, 2.44%, 3.27%, and 7.34% in terms of mean absolute error and also has superior long-term prediction performance than state-of-the-art baselines.
更多查看译文
关键词
Multi-scale spatial–temporal modeling,Sampling convolution,Attention mechanism,Traffic flow prediction
AI 理解论文
溯源树
样例
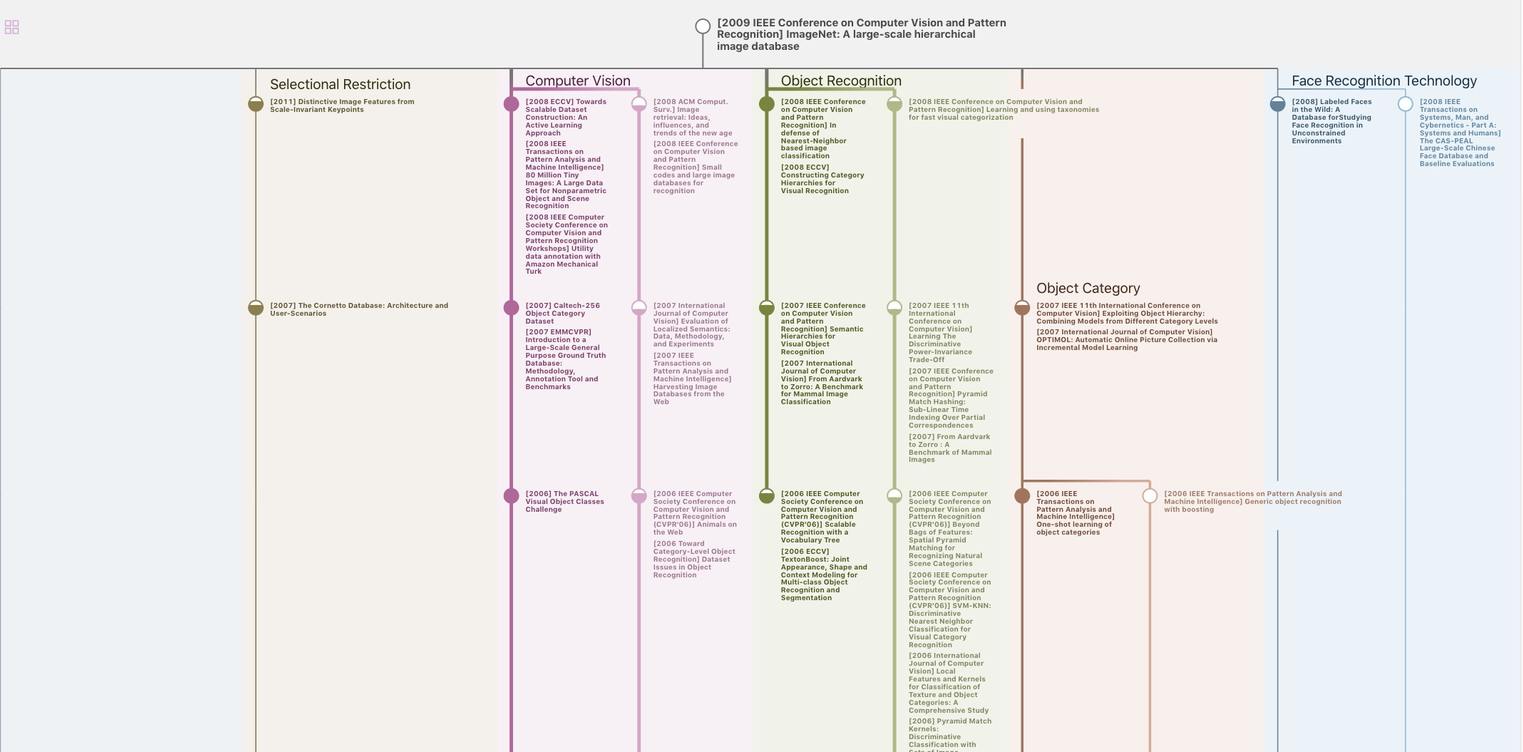
生成溯源树,研究论文发展脉络
Chat Paper
正在生成论文摘要