Target Oriented Dynamic Adaption for Cross-Domain Few-Shot Learning
Neural Processing Letters(2024)
摘要
Few-shot learning has achieved satisfactory progress over the years, but these methods implicitly hypothesize that the data in the base (source) classes and novel (target) classes are sampled from the same data distribution (domain), which is often invalid in reality. The purpose of cross-domain few-shot learning (CD-FSL) is to successfully identify novel target classes with a small quantity of labeled instances on the target domain under the circumstance of domain shift between the source domain and the target domain. However, in CD-FSL, the knowledge learned by the network on the source domain often suffers from the situation of inadaptation when it is transferred to the target domain, since the instances on the source and target domains do not obey the same data distribution. To surmount this problem, we propose a Target Oriented Dynamic Adaption (TODA) model, which uses a tiny amount of target data to orient the network to dynamically adjust and adapt during training. Specifically, this work proposes a domain-specific adapter to ameliorate the network inadaptability issues in transfer to the target domain. The domain-specific adapter can make the extracted features more specific to the tasks in the target domain and reduce the impact of tasks in the source domain by combining them with the mainstream backbone network. In addition, we propose an adaptive optimization method in the network optimization process, which assigns different weights according to the importance of different optimization tasks. Extensive experiments on several benchmark datasets demonstrate the effectiveness of our TODA method.
更多查看译文
关键词
Cross-domain few-shot learning,Domain shift,Domain-specific adapter,Adaptive optimization
AI 理解论文
溯源树
样例
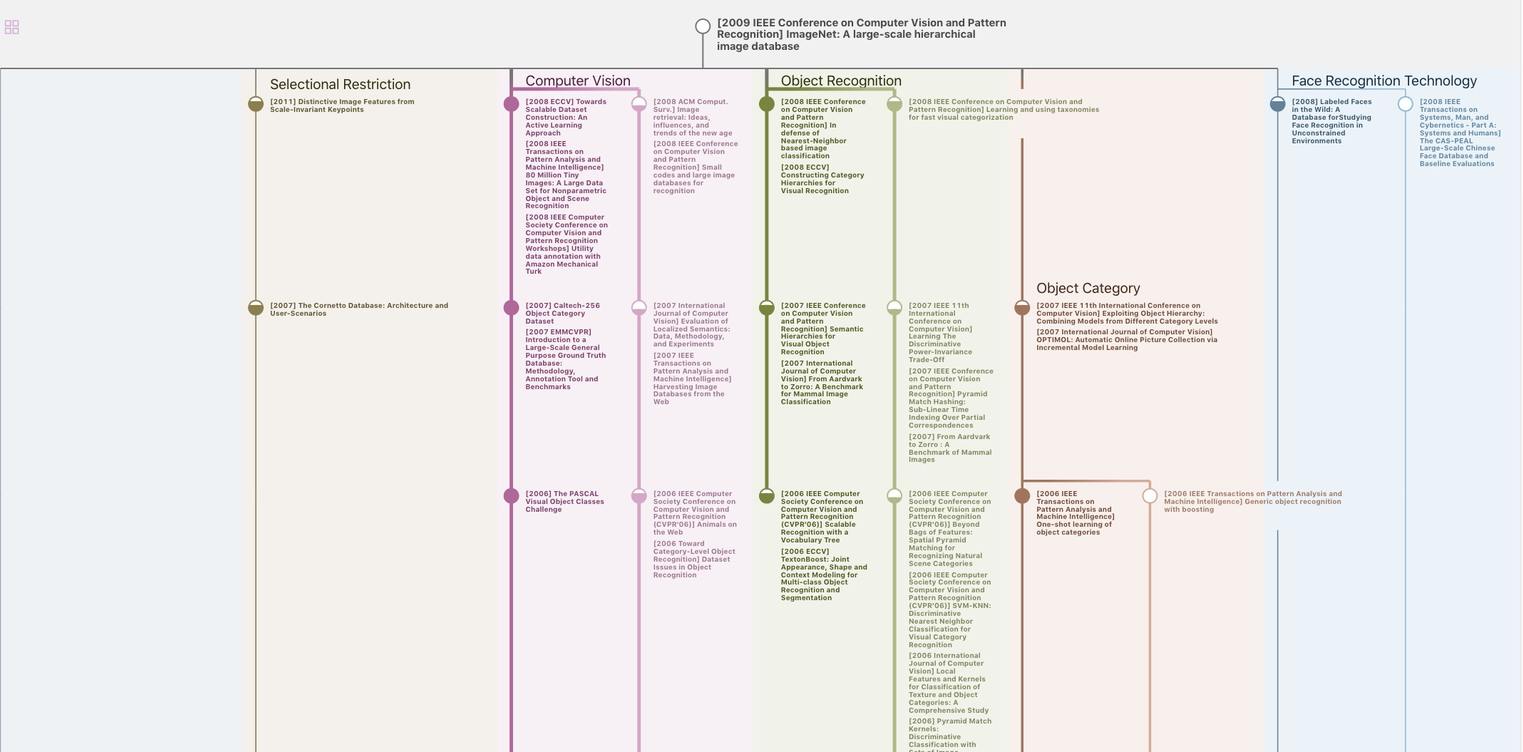
生成溯源树,研究论文发展脉络
Chat Paper
正在生成论文摘要